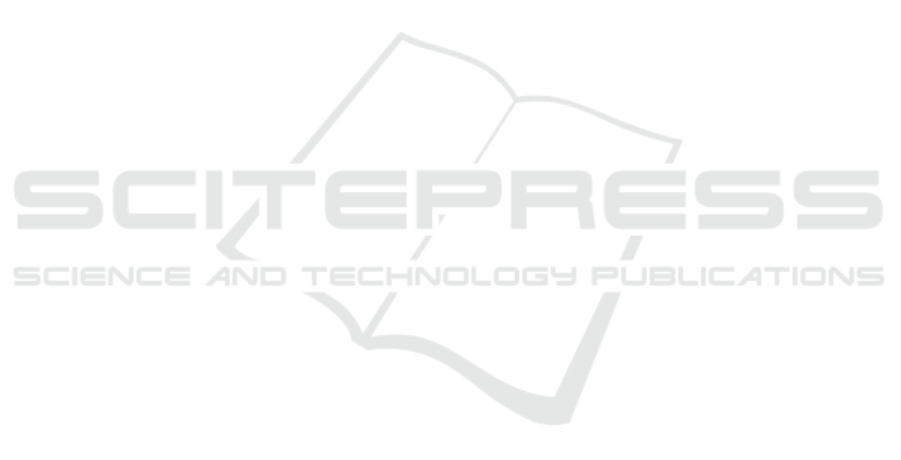
Cameras, pages 243–252. Springer International Pu-
blishing, Cham.
Gauthier, J. (2014). Conditional generative adversarial nets
for convolutional face generation. Class Project for
Stanford CS231N: Convolutional Neural Networks for
Visual Recognition, Winter semester, 2014(5):2.
Gil-Jim
´
enez, P., L
´
opez-Sastre, R., Siegmann, P., Acevedo-
Rodr
´
ıguez, J., and Maldonado-Basc
´
on, S. (2007). Au-
tomatic Control of Video Surveillance Camera Sabo-
tage, pages 222–231. Springer Berlin Heidelberg,
Berlin, Heidelberg.
Goodfellow, I., Pouget-Abadie, J., Mirza, M., Xu, B.,
Warde-Farley, D., Ozair, S., Courville, A., and Ben-
gio, Y. (2014). Generative adversarial nets. In Advan-
ces in neural information processing systems, pages
2672–2680.
Guler, P., Emeksiz, D., Temizel, A., Teke, M., and Temizel,
T. T. (2016). Real-time multi-camera video analytics
system on gpu. Journal of Real-Time Image Proces-
sing, 11(3):457–472.
Harasse, S., Bonnaud, L., Caplier, A., and Desvignes, M.
(2004). Automated camera dysfunctions detection.
In 6th IEEE Southwest Symposium on Image Analy-
sis and Interpretation, 2004., pages 36–40.
Huang, D.-Y., Chen, C.-H., Chen, T.-Y., Hu, W.-C., and
Chen, B.-C. (2014). Rapid detection of camera tampe-
ring and abnormal disturbance for video surveillance
system. Journal of Visual Communication and Image
Representation, 25(8):1865 – 1877.
Ivanov, A. and Yudin, D. (2019). Visibility loss detection
for video camera using deep convolutional neural net-
works: Volume 1. pages 434–443.
Javadi, M. S., Kadim, Z., Woon, H. H., Khairunnisa, M. J.,
and Samudin, N. (2015). Video stabilization and tam-
pering detection for surveillance systems using homo-
graphy. In 2015 International Conference on Compu-
ter, Communications, and Control Technology (I4CT),
pages 275–279.
Kim, K., Chalidabhongse, T. H., Harwood, D., and Da-
vis, L. (2005). Real-time foreground–background seg-
mentation using codebook model. Real-time imaging,
11(3):172–185.
Kryjak, T., Komorkiewicz, M., and Gorgon, M. (2012).
Fpga implementation of camera tamper detection in
real-time. In Proceedings of the 2012 Conference on
Design and Architectures for Signal and Image Pro-
cessing, pages 1–8.
Kylberg, G. (2011). Kylberg Texture Dataset v. 1.0. Centre
for Image Analysis, Swedish University of Agricultu-
ral Sciences and Uppsala University.
Ledig, C., Theis, L., Husz
´
ar, F., Caballero, J., Cunning-
ham, A., Acosta, A., Aitken, A. P., Tejani, A., Totz,
J., Wang, Z., et al. (2017). Photo-realistic single
image super-resolution using a generative adversarial
network. In CVPR, volume 2, page 4.
Lee, G.-b., Lee, M.-j., and Lim, J. (2015). Unified camera
tamper detection based on edge and object informa-
tion. Sensors, 15(5):10315–10331.
Lee, G.-b., Shin, Y.-c., Park, J.-h., and Lee, M.-j. (2014).
Low-complexity camera tamper detection based on
edge information.
Lin, D. T. and Wu, C. H. (2012). Real-time active tampering
detection of surveillance camera and implementation
on digital signal processor. In 2012 Eighth Internatio-
nal Conference on Intelligent Information Hiding and
Multimedia Signal Processing, pages 383–386.
Mantini, P. and Shah, S. K. (2014). Human trajectory fore-
casting in indoor environments using geometric con-
text. In Proceedings of the 2014 Indian Conference
on Computer Vision Graphics and Image Processing,
page 64. ACM.
Mantini, P. and Shah, S. K. (2015). Person re-identification
using geometry constrained human trajectory mo-
deling. In Technologies for Homeland Security (HST),
2015 IEEE International Symposium on, pages 1–6.
IEEE.
Mantini, P. and Shah, S. K. (2016a). Camera placement
optimization conditioned on human behavior and 3d
geometry. In VISIGRAPP (3: VISAPP), pages 227–
237.
Mantini, P. and Shah, S. K. (2016b). Multiple people
tracking using contextual trajectory forecasting. In
Technologies for Homeland Security (HST), 2016
IEEE Symposium on, pages 1–6. IEEE.
Mantini, P. and Shah, S. K. (2017). A signal detection
theory approach for camera tamper detection. In Ad-
vanced Video and Signal Based Surveillance (AVSS),
2017 14th IEEE International Conference on, pages
1–6. IEEE.
Nie, D., Trullo, R., Lian, J., Petitjean, C., Ruan, S.,
Wang, Q., and Shen, D. (2017). Medical image synt-
hesis with context-aware generative adversarial net-
works. In International Conference on Medical Image
Computing and Computer-Assisted Intervention, pa-
ges 417–425. Springer.
Osborne, M. J. and Rubinstein, A. (1994). A course in game
theory. MIT press.
Radford, A., Metz, L., and Chintala, S. (2015). Unsuper-
vised representation learning with deep convolutio-
nal generative adversarial networks. arXiv preprint
arXiv:1511.06434.
Raghavan, A., Price, R., and Liu, J. (2012). Detection
of scene obstructions and persistent view changes in
transportation camera systems. In 2012 15th Interna-
tional IEEE Conference on Intelligent Transportation
Systems, pages 957–962.
Ribnick, E., Atev, S., Masoud, O., Papanikolopoulos, N.,
and Voyles, R. (2006). Real-time detection of camera
tampering. In 2006 IEEE International Conference on
Video and Signal Based Surveillance, pages 10–10.
Saglam, A. and Temizel, A. (2009). Real-time adaptive ca-
mera tamper detection for video surveillance. In 2009
Sixth IEEE International Conference on Advanced Vi-
deo and Signal Based Surveillance, pages 430–435.
Shih, C. C., Chen, S. C., Hung, C. F., Chen, K. W., Lin,
S. Y., Lin, C. W., and Hung, Y. P. (2013). Real-time
camera tampering detection using two-stage scene
matching. In 2013 IEEE International Conference on
Multimedia and Expo (ICME), pages 1–6.
VISAPP 2019 - 14th International Conference on Computer Vision Theory and Applications
94