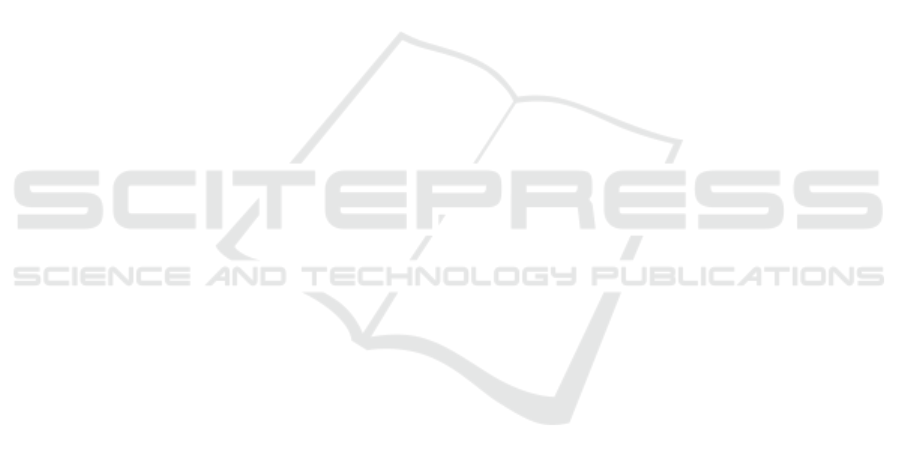
ACKNOWLEDGEMENTS
This work has been supported by the Croatian Science
Foundation under Project IP-06-2016-2092.
REFERENCES
Bani
´
c, N. and Lon
ˇ
cari
´
c, S. (2015a). Color Cat: Remembe-
ring Colors for Illumination Estimation. Signal Pro-
cessing Letters, IEEE, 22(6):651–655.
Bani
´
c, N. and Lon
ˇ
cari
´
c, S. (2015b). Using the red chroma-
ticity for illumination estimation. In Image and Signal
Processing and Analysis (ISPA), 2015 9th Internatio-
nal Symposium on, pages 131–136. IEEE.
Bani
´
c, N. and Lon
ˇ
cari
´
c, S. (2017). Unsupervised
Learning for Color Constancy. arXiv preprint
arXiv:1712.00436.
Bani
´
c, N. and Lon
ˇ
cari
´
c, S. (2013). Using the Random
Sprays Retinex Algorithm for Global Illumination Es-
timation. In Proceedings of The Second Croatian
Computer Vision Workshopn (CCVW 2013), pages 3–
7. University of Zagreb Faculty of Electrical Engi-
neering and Computing.
Bani
´
c, N. and Lon
ˇ
cari
´
c, S. (2014a). Color Rabbit: Gui-
ding the Distance of Local Maximums in Illumina-
tion Estimation. In Digital Signal Processing (DSP),
2014 19th International Conference on, pages 345–
350. IEEE.
Bani
´
c, N. and Lon
ˇ
cari
´
c, S. (2014b). Improving the White
patch method by subsampling. In Image Processing
(ICIP), 2014 21st IEEE International Conference on,
pages 605–609. IEEE.
Bani
´
c, N. and Lon
ˇ
cari
´
c, S. (2015a). A Perceptual Measure
of Illumination Estimation Error. In VISAPP, pages
136–143.
Bani
´
c, N. and Lon
ˇ
cari
´
c, S. (2015b). Color Dog: Guiding the
Global Illumination Estimation to Better Accuracy. In
VISAPP, pages 129–135.
Barnard, K., Cardei, V., and Funt, B. (2002). A compa-
rison of computational color constancy algorithms. i:
Methodology and experiments with synthesized data.
Image Processing, IEEE Transactions on, 11(9):972–
984.
Barron, J. T. (2015). Convolutional Color Constancy. In
Proceedings of the IEEE International Conference on
Computer Vision, pages 379–387.
Barron, J. T. and Tsai, Y.-T. (2017). Fast Fourier Color
Constancy. In Computer Vision and Pattern Recogni-
tion, 2017. CVPR 2017. IEEE Computer Society Con-
ference on, volume 1. IEEE.
Bianco, S., Cusano, C., and Schettini, R. (2015). Color
Constancy Using CNNs. In Proceedings of the IEEE
Conference on Computer Vision and Pattern Recogni-
tion Workshops, pages 81–89.
Buchsbaum, G. (1980). A spatial processor model for object
colour perception. Journal of The Franklin Institute,
310(1):1–26.
Canon (2018). Canon celebrates 15th consecutive year of
No.1 share of global interchangeable-lens digital ca-
mera market.
Chakrabarti, A., Hirakawa, K., and Zickler, T. (2012). Color
constancy with spatio-spectral statistics. Pattern Ana-
lysis and Machine Intelligence, IEEE Transactions on,
34(8):1509–1519.
Cheng, D., Prasad, D. K., and Brown, M. S. (2014). Illu-
minant estimation for color constancy: why spatial-
domain methods work and the role of the color distri-
bution. JOSA A, 31(5):1049–1058.
Cheng, D., Price, B., Cohen, S., and Brown, M. S. (2015).
Effective learning-based illuminant estimation using
simple features. In Proceedings of the IEEE Confe-
rence on Computer Vision and Pattern Recognition,
pages 1000–1008.
Ebner, M. (2007). Color Constancy. The Wiley-IS&T Se-
ries in Imaging Science and Technology. Wiley.
Finlayson, G. D. (2013). Corrected-moment illuminant es-
timation. In Proceedings of the IEEE International
Conference on Computer Vision, pages 1904–1911.
Finlayson, G. D., Hemrit, G., Gijsenij, A., and Gehler, P.
(2017). A Curious Problem with Using the Colour
Checker Dataset for Illuminant Estimation. In Color
and Imaging Conference, volume 2017, pages 64–69.
Society for Imaging Science and Technology.
Finlayson, G. D., Hordley, S. D., and Tastl, I. (2006). Ga-
mut constrained illuminant estimation. International
Journal of Computer Vision, 67(1):93–109.
Finlayson, G. D. and Trezzi, E. (2004). Shades of gray
and colour constancy. In Color and Imaging Confe-
rence, volume 2004, pages 37–41. Society for Ima-
ging Science and Technology.
Finlayson, G. D. and Zakizadeh, R. (2014). Reproduction
angular error: An improved performance metric for
illuminant estimation. perception, 310(1):1–26.
Funt, B. and Shi, L. (2010). The rehabilitation of MaxRGB.
In Color and Imaging Conference, volume 2010,
pages 256–259. Society for Imaging Science and
Technology.
Gao, S., Han, W., Yang, K., Li, C., and Li, Y. (2014). Ef-
ficient color constancy with local surface reflectance
statistics. In European Conference on Computer Vi-
sion, pages 158–173. Springer.
Gehler, P. V., Rother, C., Blake, A., Minka, T., and Sharp, T.
(2008). Bayesian color constancy revisited. In Com-
puter Vision and Pattern Recognition, 2008. CVPR
2008. IEEE Conference on, pages 1–8. IEEE.
Gijsenij, A. and Gevers, T. (2007). Color Constancy using
Natural Image Statistics. In CVPR, pages 1–8.
Gijsenij, A. and Gevers, T. (2011). Color constancy using
natural image statistics and scene semantics. IEEE
Transactions on Pattern Analysis and Machine Intel-
ligence, 33(4):687–698.
Gijsenij, A., Gevers, T., and Lucassen, M. P. (2009). Per-
ceptual analysis of distance measures for color con-
stancy algorithms. JOSA A, 26(10):2243–2256.
Gijsenij, A., Gevers, T., and Van De Weijer, J. (2010).
Generalized gamut mapping using image derivative
Color Beaver: Bounding Illumination Estimations for Higher Accuracy
189