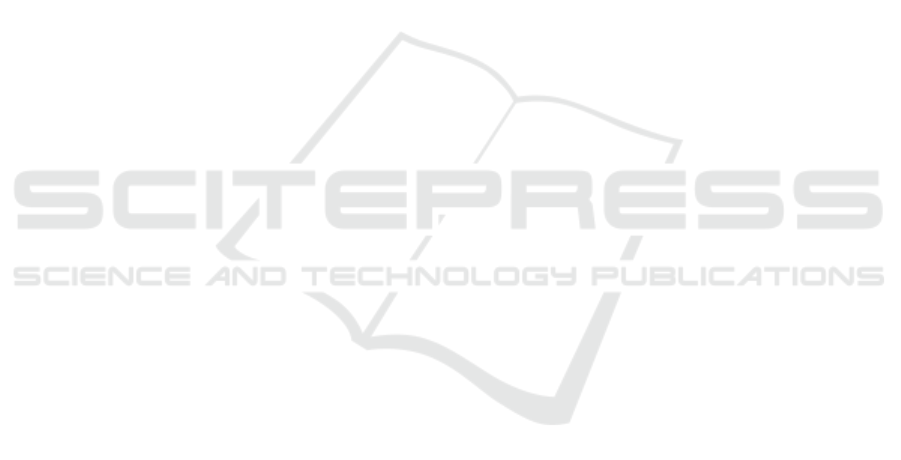
Survey of Model Compression and Acceleration for
Deep Neural Networks. CoRR.
Chu, X., Yang, W., Ouyang, W., Ma, C., Yuille, A. L., and
Wang, X. (2017). Multi-Context Attention for Human
Pose Estimation. CoRR.
Elhayek, A., De Aguiar, E., Jain, A., Tompson, J., Pishchu-
lin, L., Andriluka, M., Bregler, C., Schiele, B., and
Theobalt, C. (2015). Efficient ConvNet-based marker-
less motion capture in general scenes with a low num-
ber of cameras. In Proceedings of the IEEE Computer
Society Conference on Computer Vision and Pattern
Recognition, volume 07-12-June, pages 3810–3818.
Ionescu, C., Papava, D., Olaru, V., and Sminchisescu, C.
(2014). Human3.6M: Large scale datasets and pre-
dictive methods for 3D human sensing in natural en-
vironments. IEEE Transactions on Pattern Analysis
and Machine Intelligence, 36(7):1325–1339.
Joo, H., Liu, H., Tan, L., Gui, L., Nabbe, B., Matthews, I.,
Kanade, T., Nobuhara, S., and Sheikh, Y. (2015). Pan-
optic studio: A massively multiview system for social
motion capture. Proceedings of the IEEE Internatio-
nal Conference on Computer Vision, 2015 Inter:3334–
3342.
Li, S. and Chan, A. B. (2015). 3D human pose esti-
mation from monocular images with deep convolu-
tional neural network. Lecture Notes in Computer
Science (including subseries Lecture Notes in Artifi-
cial Intelligence and Lecture Notes in Bioinformatics),
9004:332–347.
Martinez, J., Hossain, R., Romero, J., and Little, J. J.
(2017). A simple yet effective baseline for 3d human
pose estimation.
Mehta, D., Sridhar, S., Sotnychenko, O., Rhodin, H.,
Shafiei, M., Seidel, H.-P., Xu, W., Casas, D., and The-
obalt, C. (2017). VNect: Real-time 3D Human Pose
Estimation with a Single RGB Camera. In ACM Tran-
sactions on Graphics, page 14.
Newell, A., Yang, K., and Deng, J. (2016). Stacked Hour-
glass Networks for Human Pose Estimation. Euro-
pean Conference on Computer Vision, pages 483–499.
Rafi, U., Leibe, B., Gall, J., and Kostrikov, I. (2016). An
Efficient Convolutional Network for Human Pose Es-
timation. Procedings of the British Machine Vision
Conference 2016, pages 109.1–109.11.
Shotton, J., Fitzgibbon, A., Cook, M., Sharp, T., Finocchio,
M., Moore, R., Kipman, A., and Blake, A. (2013).
Real-time human pose recognition in parts from single
depth images. Studies in Computational Intelligence,
411:119–135.
Tekin, B., Marquez-Neila, P., Salzmann, M., and Fua, P.
(2017). Learning to Fuse 2D and 3D Image Cues for
Monocular Body Pose Estimation. Proceedings of the
IEEE International Conference on Computer Vision,
2017-October:3961–3970.
Toshev, A. and Szegedy, C. (2013). DeepPose: Human Pose
Estimation via Deep Neural Networks. 2014 IEEE
Conference on Computer Vision and Pattern Recogni-
tion, pages 1653–1660.
Wei, S. E., Ramakrishna, V., Kanade, T., and Sheikh,
Y. (2016). Convolutional pose machines. Pro-
ceedings of the IEEE Computer Society Conference
on Computer Vision and Pattern Recognition, 2016-
December:4724–4732.
Wu, S., Zhong, S., and Liu, Y. (2017). Deep residual lear-
ning for image steganalysis. In Multimedia Tools and
Applications, pages 1–17.
Zhang, L., Sturm, J., Cremers, D., and Lee, D. (2012). Real-
time human motion tracking using multiple depth ca-
meras. Intelligent Robots and Systems (IROS), 2012
IEEE/RSJ International Conference on, pages 2389–
2395.
VISAPP 2019 - 14th International Conference on Computer Vision Theory and Applications
724