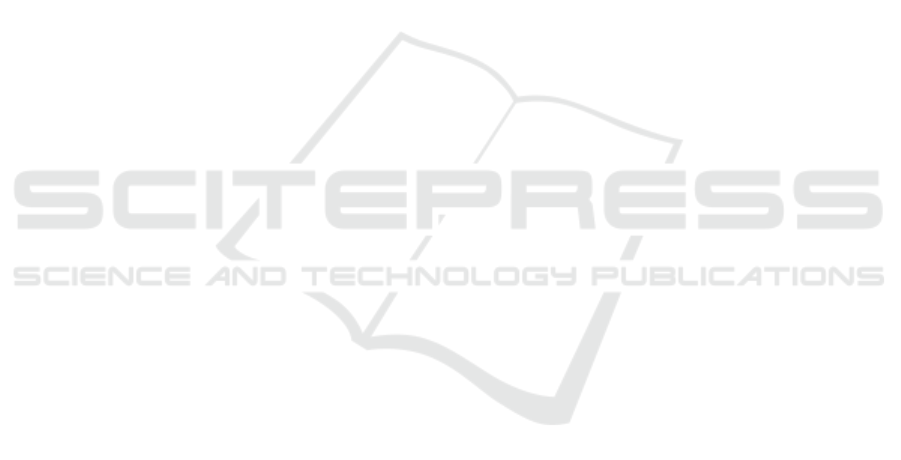
tion. Physical Work and Effort G. Borg (ED), pages
255–256.
Beaver, L. (2016). The smartwatch report: Forecasts,
adoption trends, and why the market isn’t living
up to the hype. https://www.businessinsider.
com/smartwatch-and-wearables-research-\
forecasts-trends-market-use-cases-2016-9.
Benz Scott, L. A., Ben-Or, K., and Allen, J. K. (2002). Why
are women missing from outpatient cardiac rehabilita-
tion programs? a review of multilevel factors affect-
ing referral, enrollment, and completion. Journal of
Women’s Health, 11(9):773–791.
Bharti, P., De, D., Chellappan, S., and Das, S. K. (2018a).
Human: Complex activity recognition with multi-
modal multi-positional body sensing. IEEE Transac-
tions on Mobile Computing, pages 1–1.
Bharti, P., Panwar, A., Gopalakrishna, G., and Chellappan,
S. (2018b). Watch-dog: detecting self-harming activi-
ties from wrist worn accelerometers. IEEE Journal of
Biomedical and Health Informatics, 22/3:686–696.
Borg, G. (1998). Borg’s perceived exertion and pain scales.
Human kinetics.
Breiman, L. (2001). Random forests. Machine learning,
45(1):5–32.
Burns, A., Greene, B. R., McGrath, M. J., O’Shea, T. J.,
Kuris, B., Ayer, S. M., Stroiescu, F., and Cionca, V.
(2010). Shimmer–a wireless sensor platform for non-
invasive biomedical research. IEEE Sensors Journal,
10(9):1527–1534.
De, D., Bharti, P., Das, S. K., and Chellappan, S. (2015).
Multimodal wearable sensing for fine-grained activity
recognition in healthcare. IEEE Internet Computing,
19(5):26–35.
de la Concepci
´
on, M.
´
A.
´
A., Morillo, L. M. S., Garc
´
ıa, J.
A.
´
A., and Gonz
´
alez-Abril, L. (2017). Mobile activ-
ity recognition and fall detection system for elderly
people using ameva algorithm. Pervasive and Mobile
Computing, 34:3–13.
Del Rosario, M. B., Wang, K., Wang, J., Liu, Y., Brodie,
M., Delbaere, K., Lovell, N. H., Lord, S. R., and Red-
mond, S. J. (2014). A comparison of activity classi-
fication in younger and older cohorts using a smart-
phone. Physiological measurement, 35(11):2269.
Gupta, P. and Dallas, T. (2014). Feature selection and ac-
tivity recognition system using a single triaxial ac-
celerometer. IEEE Transactions on Biomedical En-
gineering, 61(6):1780–1786.
Hwang, H. and Haddad, R. A. (1995). Adaptive median
filters: new algorithms and results. IEEE Transactions
on image processing, 4(4):499–502.
Jiang, W. and Yin, Z. (2015). Human activity recognition
using wearable sensors by deep convolutional neural
networks. In Proceedings of the 23rd ACM interna-
tional conference on Multimedia, pages 1307–1310.
ACM.
Kaur, R. and Kaur, P. D. (2017). Review on fall detection
techniques based on elder people. International Jour-
nal of Advanced Research in Computer Science, 8(3).
Landau, H. (1967). Sampling, data transmission, and the
nyquist rate. Proceedings of the IEEE, 55(10):1701–
1706.
Larose, D. T. (2005). K-nearest neighbor algorithm. Dis-
covering Knowledge in Data: An Introduction to Data
Mining, pages 90–106.
Lee, L. and Grimson, W. E. L. (2002). Gait analysis for
recognition and classification. In Automatic Face and
Gesture Recognition, 2002. Proceedings. Fifth IEEE
International Conference on, pages 155–162. IEEE.
Levy, B. R. and Myers, L. M. (2004). Preventive health be-
haviors influenced by self-perceptions of aging. Pre-
ventive medicine, 39(3):625–629.
Mika, S., Ratsch, G., Weston, J., Scholkopf, B., and
Mullers, K.-R. (1999). Fisher discriminant analysis
with kernels. In Neural Networks for Signal Process-
ing IX, 1999. Proceedings of the 1999 IEEE Signal
Processing Society Workshop., pages 41–48. IEEE.
Reyes-Ortiz, J.-L., Oneto, L., Sam
`
a, A., Parra, X., and
Anguita, D. (2016). Transition-aware human activ-
ity recognition using smartphones. Neurocomputing,
171:754–767.
Safavian, S. R. and Landgrebe, D. (1991). A survey of de-
cision tree classifier methodology. IEEE transactions
on systems, man, and cybernetics, 21(3):660–674.
San-Segundo, R., Montero, J. M., Barra-Chicote, R., Fer-
nandez, F., and Pardo, J. M. (2016). Feature extrac-
tion from smartphone inertial signals for human activ-
ity segmentation. Signal Processing, 120:359 – 372.
S
´
anchez, D., Tentori, M., and Favela, J. (2008). Activity
recognition for the smart hospital. IEEE intelligent
systems, 23(2).
Scholkopf, B., Sung, K.-K., Burges, C. J., Girosi, F.,
Niyogi, P., Poggio, T., and Vapnik, V. (1997). Com-
paring support vector machines with gaussian kernels
to radial basis function classifiers. IEEE transactions
on Signal Processing, 45(11):2758–2765.
Sousa, W., Souto, E., Rodrigres, J., Sadarc, P., Jalali, R.,
and El-Khatib, K. (2017). A comparative analysis of
the impact of features on human activity recognition
with smartphone sensors. In Proceedings of the 23rd
Brazillian Symposium on Multimedia and the Web,
pages 397–404. ACM.
Suykens, J. A. and Vandewalle, J. (1999). Least squares
support vector machine classifiers. Neural processing
letters, 9(3):293–300.
Valencia, H. E., Savage, P. D., and Ades, P. A. (2011). Car-
diac rehabilitation participation in underserved pop-
ulations. Journal of cardiopulmonary rehabilitation
and prevention, 31(4):203–210.
Wang, Y., Wu, K., and Ni, L. M. (2017). Wifall: Device-
free fall detection by wireless networks. IEEE Trans-
actions on Mobile Computing, 16(2):581–594.
Wold, S., Esbensen, K., and Geladi, P. (1987). Principal
component analysis. Chemometrics and intelligent
laboratory systems, 2(1-3):37–52.
HEALTHINF 2019 - 12th International Conference on Health Informatics
374