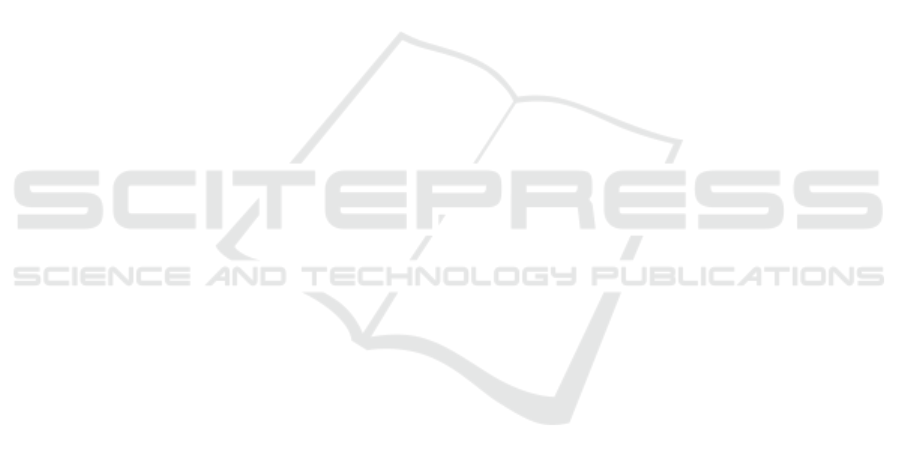
REFERENCES
Armato, S. G., McLennan, G., Bidaut, L., McNitt-Gray,
M. F., Meyer, C. R., Reeves, A. P., Zhao, B., Aberle,
D. R., Henschke, C. I., Hoffman, E. A., Kazerooni,
E. A., MacMahon, H., Beek, E. J. R., Yankelevitz,
D., Biancardi, A. M., Bland, P. H., Brown, M. S.,
Engelmann, R. M., Laderach, G. E., Max, D., Pais,
R. C., Qing, D. P.-Y., Roberts, R. Y., Smith, A. R.,
Starkey, A., Batra, P., Caligiuri, P., Farooqi, A., Gla-
dish, G. W., Jude, C. M., Munden, R. F., Petkovska,
I., Quint, L. E., Schwartz, L. H., Sundaram, B., Dodd,
L. E., Fenimore, C., Gur, D., Petrick, N., Freymann,
J., Kirby, J., Hughes, B., Vande Casteele, A., Gupte,
S., Sallam, M., Heath, M. D., Kuhn, M. H., Dharaiya,
E., Burns, R., Fryd, D. S., Salganicoff, M., Anand, V.,
Shreter, U., Vastagh, S., Croft, B. Y., and Clarke, L. P.
(2011). The lung image database consortium (lidc)
and image database resource initiative (idri): A com-
pleted reference database of lung nodules on ct scans.
Medical Physics, 38(2):915–931.
Awai, K., Murao, K., Ozawa, A., Komi, M., Hayakawa,
H., Hori, S., and Nishimura, Y. (2004). Pulmonary
nodules at chest ct: Effect of computer-aided diagno-
sis on radiologists detection performance. Radiology,
230(2):347–352.
Brown, M. S., Lo, P., Goldin, J. G., Barnoy, E., Kim, G.
H. J., McNitt-Gray, M. F., and Aberle, D. R. (2014).
Toward clinically usable cad for lung cancer screen-
ing with computed tomography. European Radiology,
24(11):2719–2728.
Ciompi, F., Chung, K., van Riel, S. J., Setio, A. A. A.,
Gerke, P. K., Jacobs, C., Scholten, E. T., Schaefer-
Prokop, C., Wille, M. M. W., Marchiano, A., Pasto-
rino, U., Prokop, M., and van Ginneken, B. (2016).
Towards automatic pulmonary nodule management in
lung cancer screening with deep learning. CoRR,
abs/1610.09157.
Ding, J., Li, A., Hu, Z., and Wang, L. (2017). Accurate
pulmonary nodule detection in computed tomography
images using deep convolutional neural networks. In
Descoteaux, M., Maier-Hein, L., Franz, A., Jannin,
P., Collins, D. L., and Duchesne, S., editors, Medi-
cal Image Computing and Computer-Assisted Inter-
vention MICCAI 2017, pages 559–567, Cham. Sprin-
ger International Publishing.
Dou, Q., Chen, H., Jin, Y., Lin, H., Qin, J., and Heng, P.-A.
(2017). Automated pulmonary nodule detection via 3d
convnets with online sample filtering and hybrid-loss
residual learning. In Descoteaux, M., Maier-Hein, L.,
Franz, A., Jannin, P., Collins, D. L., and Duchesne,
S., editors, Medical Image Computing and Computer-
Assisted Intervention MICCAI 2017, pages 630–638,
Cham. Springer International Publishing.
Duggan, N., Bae, E., Shen, S., Hsu, W., Bui, A., Jones, E.,
Glavin, M., and Vese, L. (2015). A technique for lung
nodule candidate detection in ct using global minimi-
zation methods. In Tai, X.-C., Bae, E., Chan, T. F., and
Lysaker, M., editors, Energy Minimization Methods in
Computer Vision and Pattern Recognition, pages 478–
491, Cham. Springer International Publishing.
Gao, M., Bagci, U., Lu, L., Wu, A., Buty, M., Shin, H.-C.,
Roth, H., Papadakis, G. Z., Depeursinge, A., Sum-
mers, R. M., Xu, Z., and Mollura, D. J. (2016). Ho-
listic classification of ct attenuation patterns for in-
terstitial lung diseases via deep convolutional neural
networks. Computer Methods in Biomechanics and
Biomedical Engineering: Imaging & Visualization,
0(0):1–6.
Huang, X., Shan, J., and Vaidya, V. (2017). Lung nodule de-
tection in ct using 3d convolutional neural networks.
In 2017 IEEE 14th International Symposium on Bio-
medical Imaging (ISBI 2017), pages 379–383.
Jia, Y., Shelhamer, E., Donahue, J., Karayev, S., Long, J.,
Girshick, R., Guadarrama, S., and Darrell, T. (2014).
Caffe: Convolutional architecture for fast feature em-
bedding. arXiv preprint arXiv:1408.5093.
Li, W., Cao, P., Zhao, D., and Wang, J. (2016). Pulmonary
nodule classification with deep convolutional neural
networks on computed tomography images. Com-
putational and Mathematical Methods in Medicine,
2016(0):7.
Liu, W., Anguelov, D., Erhan, D., Szegedy, C., Reed, S.,
Fu, C.-Y., and Berg, A. C. (2016). Ssd: Single shot
multibox detector. In Leibe, B., Matas, J., Sebe, N.,
and Welling, M., editors, Computer Vision – ECCV
2016, pages 21–37, Cham. Springer International Pu-
blishing.
Lopez Torres, E., Fiorina, E., Pennazio, F., Peroni, C., Sa-
letta, M., Camarlinghi, N., Fantacci, M. E., and Ce-
rello, P. (2015). Large scale validation of the m5l
lung cad on heterogeneous ct datasets. Med Phys,
42(4):1477–1489.
Reeves, A. P. and Biancardi, A. M. (2011). The lung
image database consortium (lidc) nodule size report.
http://www.via.cornell.edu/lidc/.
Setio, A. A. A., Traverso, A., de Bel, T., Berens, M. S.,
van den Bogaard, C., Cerello, P., Chen, H., Dou, Q.,
Fantacci, M. E., Geurts, B., van der Gugten, R., Heng,
P. A., Jansen, B., de Kaste, M. M., Kotov, V., Lin,
J. Y.-H., Manders, J. T., Sora-Mengana, A., Garca-
Naranjo, J. C., Papavasileiou, E., Prokop, M., Saletta,
M., Schaefer-Prokop, C. M., Scholten, E. T., Schol-
ten, L., Snoeren, M. M., Torres, E. L., Vandemeule-
broucke, J., Walasek, N., Zuidhof, G. C., van Gin-
neken, B., and Jacobs, C. (2017). Validation, com-
parison, and combination of algorithms for automatic
detection of pulmonary nodules in computed tomo-
graphy images: The luna16 challenge. Medical Image
Analysis, 42:1 – 13.
world Health Organization (2018). Cancer.
http://www.who.int/mediacentre/factsheets/fs297/en/.
Accessed: 2018-09-03.
Yaniv Bar, Idit Diamant, L. W. H. G. (2015). Deep lear-
ning with non-medical training used for chest patho-
logy identification.
Zhao, Y., Zhao, L., Yan, Z., Wolf, M., and Zhan, Y. (2018).
A deep-learning based automatic pulmonary nodule
detection system.
Zhu, W., Liu, C., Fan, W., and Xie, X. (2018). Deeplung:
Deep 3d dual path nets for automated pulmonary no-
dule detection and classification. In 2018 IEEE Win-
ter Conference on Applications of Computer Vision,
WACV 2018, Lake Tahoe, NV, USA, March 12-15,
2018, pages 673–681.
VISAPP 2019 - 14th International Conference on Computer Vision Theory and Applications
544