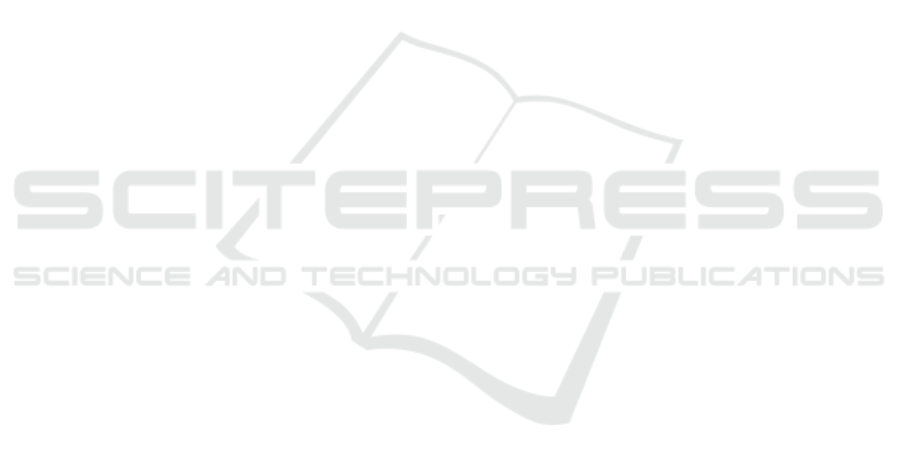
Economic Affairs and Energy (01MD15005).
REFERENCES
Austin, S. R. et al. (2015). Why Summary Comorbidity
Measures such as the Charlson Comorbidity Index and
Elixhauser Score Work. Medical care, 53(9):e65.
Bai, B. M., Mangathayaru, N., and Rani, B. P. (2015). An
Approach to Find Missing Values in Medical Datasets.
In Proc. Intl. Conf. on Engineering & MIS, pages
70:1–70:7, New York, NY, USA. ACM.
Bewick, V., Cheek, L., and Ball, J. (2004). Statistics Re-
view 13: Receiver Operating Characteristic Curves.
Critical Care, 8(6):508.
Caruana, R. et al. (2015). Intelligible Models for Health-
Care: Predicting Pneumonia Risk and Hospital 30-day
Readmission. In Intl. Conf. Knowledge Discovery and
Data Mining, pages 1721–1730, New York, NY, USA.
ACM.
Che, Z., Purushotham, S., Khemani, R., and Liu, Y. (2016).
Interpretable Deep Models for ICU Outcome Predic-
tion. AMIA Symposium, 2016:371–380.
Elith, J., Leathwick, J. R., and Hastie, T. (2008). A Working
Guide to Boosted Regression Trees.
Elixhauser, A. et al. (1998). Comorbidity Measures for Use
with Administrative Data. Medical Care, 36(1):8–27.
Flynn, N. and Dawnay, A. (2015). A Simple Electronic
Alert for Acute Kidney Injury. Ann Clin Biochem,
52(2):206–212.
Glas, A. S. et al. (2003). The Diagnostic Odds Ratio: A Sin-
gle Indicator of Test Performance. Journal of Clinical
Epidemiology, 56(11):1129–1135.
Goodman, B. and Flaxman, S. (2016). EU Regulations on
Algorithmic Decision-making and a ”Right to Expla-
nation”. In ICML Workshop on Human Interpretabil-
ity in Machine Learning.
Gron, A. (2017). Hands-On Machine Learning with Scikit-
Learn and TensorFlow. O’Reilly Media, Inc., 1st edi-
tion.
Huen, S. C. and Parikh, C. R. (2012). Predicting Acute
Kidney Injury after Cardiac Surgery: a Systematic Re-
view. Ann Thorac Surg, 93(1):337–47.
Jiang, W. et al. (2016). Dynamic Predictive Scores for Car-
diac Surgery–Associated Acute Kidney Injury. Am
Heart J, 5(8).
Jiang, W. et al. (2017). Validation of Four Prediction
Scores for Cardiac Surgery-Associated Acute Kidney
Injury in Chinese Patients. Braz J Cardiovasc Surg,
32(6):481–486.
Johnson, A. E. et al. (2016). MIMIC-III, a Freely Accessi-
ble Critical Care Database. Scientific Data, 3.
Katuwal, G. J. and Chen, R. (2016). Machine Learning
Model Interpretability for Precision Medicine. ArXiv
e-prints.
Latini, R., Aleksova, A., and Masson, S. (2016). Novel
Biomarkers and Therapies in Cardiorenal Syndrome.
Letham, B. et al. (2015). Interpretable Classifiers us-
ing Rules and Bayesian Analysis: Building a Better
Stroke Prediction Model. Annals of Applied Statistics,
9(3):1350–1371.
Lipton, Z. C. (2016). The Mythos of Model Interpretability.
ArXiv e-prints.
Lopes, J. A. and Jorge, S. (2013). The RIFLE and AKIN
Classifications for Acute Kidney Injury: a Critical and
Comprehensive Review. Clin Kidney J, 6(1):8–14.
McKinney, W. (2010). Data Structures for Statistical Com-
puting in Python. In van der Walt, S. and Millman,
J., editors, Proc. 9th Python in Science Conference,
pages 51 – 56.
Moon, H. et al. (2007). Ensemble Methods for Classifica-
tion of Patients for Personalized Medicine with High-
Dimensional Data. Artif Intell Med, 41(3):197–207.
Ng, S. Y. et al. (2014). Prediction of Acute Kidney Injury
within 30 Days of Cardiac Surgery. J Thorac Cardio-
vasc Surg, 147(6):1875–1883.e1.
O’Neal, Jason and others (2016). Acute Kidney Injury Fol-
lowing Cardiac Surgery: Current Understanding and
Future Directions. Critical Care, 20(1):187.
Palomba, H. et al. (2007). Acute Kidney Injury Prediction
following Elective Cardiac Surgery: AKICS Score.
Kidney International, 72(5):624–631.
Ribeiro, M. T., Singh, S., and Guestrin, C. (2016). ”Why
Should I Trust You?”: Explaining the Predictions of
Any Classifier. In Proc. 22nd Intl. Conf. on Knowl-
edge Discovery and Data Mining, pages 1135–1144,
New York, NY, USA. ACM.
Rosner, M. H. and Okusa, M. D. (2006). Acute Kidney
Injury Associated with Cardiac Surgery. Clin J Am
Soc Nephrol, 1(1):19–32.
Rossum, G. V. and Drake, F. L. (2010). Python Tutorial.
History, 42(4):1–122.
Rubinsteyn, A. and Feldman, S. (2018). fancyim-
pute: A Variety of Matrix Completion and Im-
putation Algorithms Implemented in Python.
https://github.com/iskandr/fancyimpute Accessed:
October, 2018.
Sawhney, S. et al. (2015). Acute Kidney Injury - How Does
Automated Detection Perform? Nephrol. Dial. Trans-
plant., 30(11):1853–61.
Targher, G. et al. (2008). Non-alcoholic fatty liver
disease is independently associated with an in-
creased prevalence of chronic kidney disease and
proliferative/laser-treated retinopathy in type 2 dia-
betic patients. Diabetologia, 51(3):444–450.
Thakar, C. V. et al. (2004). A Clinical Score to Predict
Acute Renal Failure after Cardiac Surgery. J Am Soc
Nephrol, 14(8):2176–7.
Valdes, G. et al. (2016). MediBoost: A Patient Stratification
Tool for Interpretable Decision Making in the Era of
Precision Medicine. Scientific Reports, 6.
Wijeysundera, D. N. et al. (2007). Derivation and Valida-
tion of a Simplified Predictive Index for Renal Re-
placement Therapy After Cardiac Surgery. JAMA,
297(16):1801.
Prediction of Acute Kidney Injury in Cardiac Surgery Patients: Interpretation using Local Interpretable Model-agnostic Explanations
387