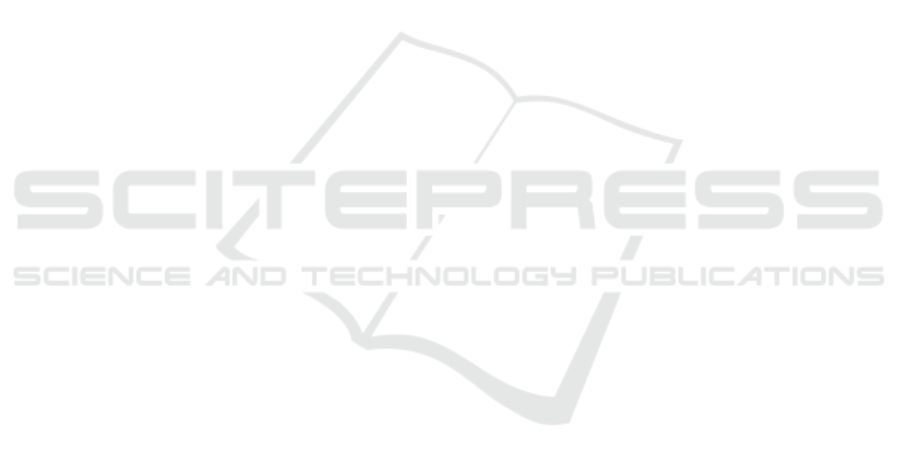
films produced by Spin Coating, the values did not
vary significantly for any of the classifiers. Therefore,
we conclude that both spreading method techniques
are very good for sensing films production, and none
of them revealed to be better than the others for VOCs
classification.
The E-nose system and the machine learning algo-
rithms applied in the present study demonstrated ca-
pability to distinguish the different VOCs in a quick,
simple and accurate way, using both sensing film pro-
duction types.
Future studies can be performed in order to ex-
plore the application of the E-nose in many different
sectors, such as food and beverage evaluation, envi-
ronmental safety or medical research. Moreover, ot-
her Machine Learning algorithms can be explored and
optimised.
REFERENCES
Barbri, N. E., Mirhisse, J., Ionescu, R., Bari, N. E., Correig,
X., Bouchikhi, B., and Llobet, E. (2009). An electro-
nic nose system based on a micro-machined gas sen-
sor array to assess the freshness of sardines. Sensors
& Actuators: B. Chemical, 141:538 – 543.
Cho, J. H. and Kurup, P. U. (2011). Decision tree appro-
ach for classification and dimensionality reduction of
electronic nose data. Sensors & Actuators: B. Chemi-
cal, 160:542 – 548.
Ghasemi-Varnamkhasti, M., Mohtasebi, S. S., Siadat, M.,
Ahmadi, H., and Razavi, S. H. (2015). Research pa-
per: From simple classification methods to machine
learning for the binary discrimination of beers using
electronic nose data. Engineering in Agriculture, En-
vironment and Food, 8:44 – 51.
Gutirrez, J. and Horrillo, M. (2014). Advances in arti-
ficial olfaction: sensors and applications. Talanta,
124:95105.
Hotel, O., Poli, J.-P., Mer-Calfati, C., Scorsone, E., and
Saada, S. (2018). A review of algorithms for saw sen-
sors e-nose based volatile compound identification.
Sensors & Actuators: B. Chemical, 255(Part 3):2472
– 2482.
Hussain, A., Semeano, A. T. S., Palma, S. I. C. J., Pina,
A. S., Almeida, J., Medrado, B. F., Padua, A. C. C. S.,
Carvalho, A. L., Dionisio, M., Li, R. W. C., Gam-
boa, H., Ulijn, R. V., Gruber, J., and Roque, A. C. A.
(2017). Liquid crystals: Tunable gas sensing gels
by cooperative assembly (adv. funct. mater. 27/2017).
Advanced Functional Materials, 27(27):n/a.
Ordukaya, E. and Karlik, B. (2017). Quality control of olive
oils using machine learning and electronic nose. Jour-
nal of Food Quality, Vol 2017 (2017).
Padua, A. C., Palma, S., Gruber, J., Gamboa, H., and Ro-
que, A. C. (2018). Design and evolution of an opto-
electronic device for vocs detection. In Proceedings of
the 11th International Joint Conference on Biomedi-
cal Engineering Systems and Technologies - Volume 1:
BIODEVICES,, pages 48–55. INSTICC, SciTePress.
Peris, M. and Escuder-Gilabert, L. (2009). A 21st century
technique for food control: Electronic noses. Analy-
tica Chimica Acta, 638(1):1 – 15.
Phaisangittisagul, E., Nagle, H. T., and Areekul, V. (2010).
Intelligent method for sensor subset selection for ma-
chine olfaction. Sensors & Actuators: B. Chemical,
145:507 – 515.
Polaka, I., Gaenko, E., Barash, O., Haick, H., and Leja, M.
(2017). Constructing interpretable classifiers to diag-
nose gastric cancer based on breath tests. Procedia
Computer Science, 104(ICTE 2016, Riga Technical
University, Latvia):279 – 285.
Sankaran, S., Khot, L. R., and Panigrahi, S. (2012). Review:
Biology and applications of olfactory sensing system:
A review. Sensors & Actuators: B. Chemical, 171-
172:1 – 17.
Siavash, E., Alfian, W., Ella, M., Ramesh P., A., and Ja-
mes A., C. (2018). Non-invasive diagnosis of diabetes
by volatile organic compounds in urine using faims
and fox4000 electronic nose. Biosensors, Vol 8, Iss 4,
p 121 (2018), (4):121.
Xu, L., He, J., Duan, S., Wu, X., and Wang, Q. (2016).
Comparison of machine learning algorithms for con-
centration detection and prediction of formaldehyde
based on electronic nose. Sensor Review, 36(2):207.
Yan, J., Xiuzhen, G., Shukai, D., Pengfei, J., Lidan, W.,
Chao, P., and Songlin, Z. (2015). Electronic nose fe-
ature extraction methods: A review. Sensors, Vol 15,
Iss 11, Pp 27804-27831 (2015), (11):27804.
Zhang, H., Chang, M., Wang, J., and Ye, S. (2008). Evalua-
tion of peach quality indices using an electronic nose
by mlr, qpst and bp network. Sensors & Actuators: B.
Chemical, 134:332 – 338.
BIODEVICES 2019 - 12th International Conference on Biomedical Electronics and Devices
64