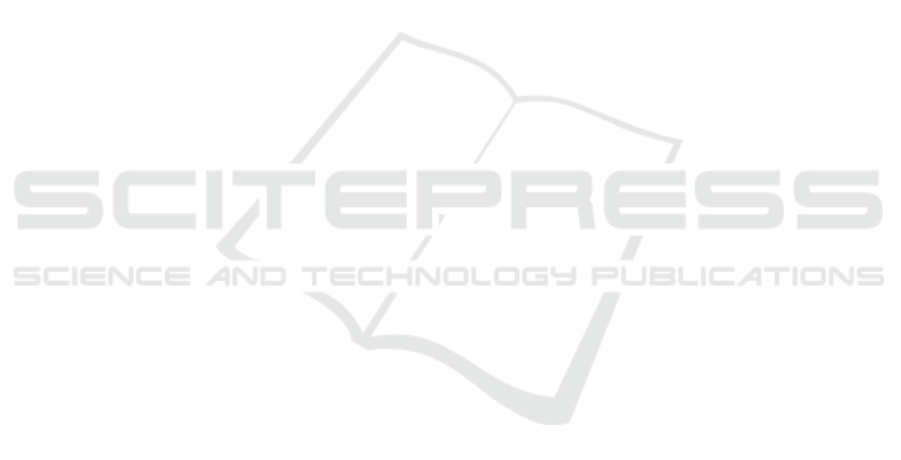
Cheng, D. and Liu, M. (2017). Combining convolutional
and recurrent neural networks for alzheimer’s disease
diagnosis using pet images. In 2017 IEEE Internati-
onal Conference on Imaging Systems and Techniques,
pages 1–5.
Cristianini, N. and Shawe-Taylor, J. (2000). An Introduction
to Support Vector Machines and Other Kernel-based
Learning Methods. Cambridge University Press.
Duarte, K. T. N. and Paiva, P. V. V. (2018). Combining
tl, svm, and voting for alzheimer’s detection. https:
//github.com/enemy537/Multi-ModalCNN.
Ferreira, L. K. and Busatto, G. F. (2011). Neuroimaging
in alzheimers disease: current role in clinical practice
and potential future applications. Clinics, 66:19–24.
Fiot, J.-B., Fripp, J., and Cohen, L. D. (2012). Combi-
ning imaging and clinical data in manifold learning:
Distance-based and graph-based extensions of lapla-
cian eigenmaps. In 2012 9th IEEE International Sym-
posium on Biomedical Imaging. IEEE.
Gray, K. R., Wolz, R., Keihaninejad, S., Heckemann, R. A.,
Aljabar, P., Hammers, A., and Rueckert, D. (2011).
Regional analysis of fdg-pet for use in the classifica-
tion of alzheimer’s disease. In IEEE International
Symposium on Biomedical Imaging: From Nano to
Macro, pages 1082–1085.
Guerreiro, R. and Bras, J. (2015). The age factor in alzhei-
mer’s disease. Genome Medicine, 7(1).
Hearst, M., Dumais, S., Osuna, E., Platt, J., and Scholkopf,
B. (1998). Support vector machines. IEEE Intelligent
Systems and their Applications, 13(4):18–28.
Jenkinson, M., Pechaud, M., and Smith, S. (2005). BET2:
MR-based estimation of brain, skull and scalp surfa-
ces. In Eleventh Annual Meeting of the Organization
for Human Brain Mapping.
Khedher, L., Ramrez, J., Grriz, J., Brahim, A., and Segovia,
F. (2015). Early diagnosis of alzheimers disease based
on partial least squares, principal component analysis
and support vector machine using segmented mri ima-
ges. Neurocomputing, 151:139 – 150.
Lebedev, A., Westman, E., Westen, G. V., Kramberger,
M., Lundervold, A., Aarsland, D., Soininen, H.,
Kłoszewska, I., Mecocci, P., Tsolaki, M., Vellas, B.,
Lovestone, S., and Simmons, A. (2014). Random fo-
rest ensembles for detection and prediction of alzhei-
mers disease with a good between-cohort robustness.
NeuroImage: Clinical, 6:115–125.
Lordkaniche (2011). Share-cg face 01 - 3d mo-
del. http://www.sharecg.com/v/55159/view/5/
3D-Model/Face-01.
on Aging, N. I. (2016). Alzheimer’s disease fact
sheet. https://www.nia.nih.gov/health/
alzheimers-disease-fact-sheet.
Petersen, R. C., Smith, G. E., Waring, S. C., Ivnik, R. J.,
Tangalos, E. G., and Kokmen, E. (1999). Mild cog-
nitive impairment: clinical characterization and out-
come. Arch. Neurol., 56:303–308.
Suk, H.-I. and Shen, D. (2016). Deep ensemble sparse re-
gression network for alzheimer’s disease diagnosis. In
Wang, L., Adeli, E., Wang, Q., Shi, Y., and Suk, H.-I.,
editors, Machine Learning in Medical Imaging, pages
113–121, Cham. Springer International.
Wang, S., Shen, Y., Chen, W., Xiao, T., and Hu, J. (2017).
Automatic recognition of mild cognitive impairment
from mri images using expedited convolutional neu-
ral networks. In Lintas, A., Rovetta, S., Verschure,
P. F., and Villa, A. E., editors, Artificial Neural Net-
works and Machine Learning – ICANN, pages 373–
380. Springer.
Zhou, T., Thung, K.-H., Zhu, X., and Shen, D. (2017). Fe-
ature learning and fusion of multimodality neuroima-
ging and genetic data for multi-status dementia diag-
nosis. In Wang, Q., Shi, Y., Suk, H.-I., and Suzuki, K.,
editors, Machine Learning in Medical Imaging, pages
132–140, Cham. Springer International Publishing.
VISAPP 2019 - 14th International Conference on Computer Vision Theory and Applications
560