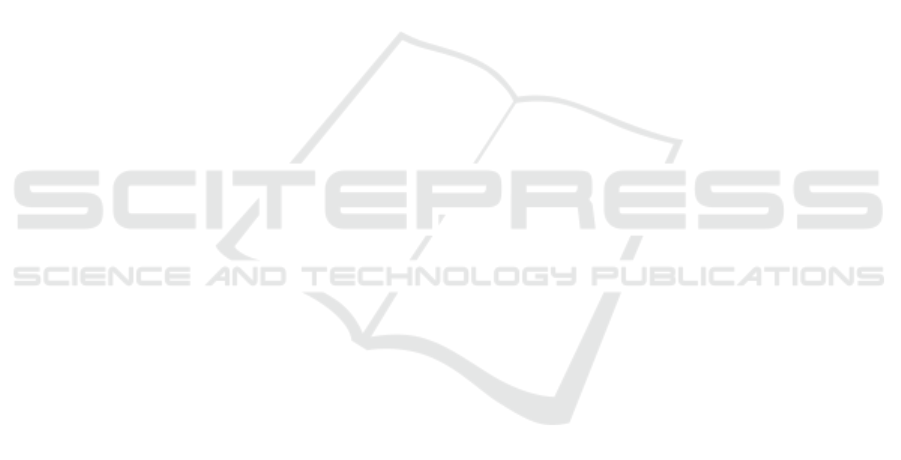
Proceedings of the IEEE conference on computer vi-
sion and pattern recognition, pages 3431–3440.
Luc, P., Couprie, C., Chintala, S., and Verbeek, J. (2016).
Semantic segmentation using adversarial networks. In
NIPS Workshop on Adversarial Training.
Mathieu, M., Couprie, C., and LeCun, Y. (2016). Deep
multi-scale video prediction beyond mean square er-
ror. ICLR.
Moriyuki, S. and Fukuda, H. (2016). High-throughput gro-
wth prediction for lactuca sativa l. seedlings using
chlorophyll fluorescence in a plant factory with arti-
ficial lighting. Frontiers in plant science, 7:394.
Nair, V. and Hinton, G. E. (2010). Rectified linear units im-
prove restricted boltzmann machines. In Proceedings
of the 27th international conference on machine lear-
ning (ICML-10), pages 807–814.
Ranzato, M., Szlam, A., Bruna, J., Mathieu, M., Collo-
bert, R., and Chopra, S. (2014). Video (language)
modeling: a baseline for generative models of natu-
ral videos. CoRR, abs/1412.6604.
Ren, M. and Zemel, R. S. (2017). End-to-end instance seg-
mentation with recurrent attention. In Proceedings of
the 2017 IEEE Conference on Computer Vision and
Pattern Recognition (CVPR), Honolulu, HI, USA, pa-
ges 21–26.
Sakurai, S., Uchiyama, H., Shimada, A., Arita, D., and
ichiro Taniguchi, R. (2018a). Two-step transfer le-
arning for semantic plant segmentation. In Procee-
dings of the 7th International Conference on Pattern
Recognition Applications and Methods - Volume 1:
ICPRAM,, pages 332–339. INSTICC, SciTePress.
Sakurai, S., Uchiyama, H., Shimada, A., Arita, D., and Ta-
niguchi, R. (2018b). Two-step transfer learning for se-
mantic plant segmentation. In 7th International Con-
ference on Pattern Recognition Applications and Met-
hods (ICPRAM2018).
Silberman, N., Sontag, D., and Fergus, R. (2014). Instance
segmentation of indoor scenes using a coverage loss.
In European Conference on Computer Vision, pages
616–631. Springer.
Srivastava, N., Hinton, G., Krizhevsky, A., Sutskever, I.,
and Salakhutdinov, R. (2014). Dropout: A simple way
to prevent neural networks from overfitting. The Jour-
nal of Machine Learning Research, 15(1):1929–1958.
Srivastava, N., Mansimov, E., and Salakhudinov, R. (2015).
Unsupervised learning of video representations using
lstms. In International conference on machine lear-
ning, pages 843–852.
Sutskever, I., Vinyals, O., and Le, Q. V. (2014). Sequence
to sequence learning with neural networks. In Advan-
ces in neural information processing systems, pages
3104–3112.
Ubbens, J. R. and Stavness, I. (2017). Deep plant pheno-
mics: a deep learning platform for complex plant phe-
notyping tasks. Frontiers in plant science, 8:1190.
Uchiyama, H., Sakurai, S., Mishima, M., Arita, D., Oka-
yasu, T., Shimada, A., and Taniguchi, R.-i. (2017).
An easy-to-setup 3d phenotyping platform for komat-
suna dataset. In The IEEE International Conference
on Computer Vision (ICCV) Workshops.
Villegas, R., Yang, J., Hong, S., Lin, X., and Lee, H. (2017).
Decomposing motion and content for natural video se-
quence prediction. ICLR.
Walter, A., Liebisch, F., and Hund, A. (2015). Plant
phenotyping: from bean weighing to image analysis.
Plant methods, 11(1):14.
Xingjian, S., Chen, Z., Wang, H., Yeung, D.-Y., Wong, W.-
K., and Woo, W.-c. (2015). Convolutional lstm net-
work: A machine learning approach for precipitation
nowcasting. In Advances in neural information pro-
cessing systems, pages 802–810.
Zaidi, M., Murase, H., and Honami, N. (1999). Neural net-
work model for the evaluation of lettuce plant gro-
wth. Journal of agricultural engineering research,
74(3):237–242.
Plant Growth Prediction using Convolutional LSTM
113