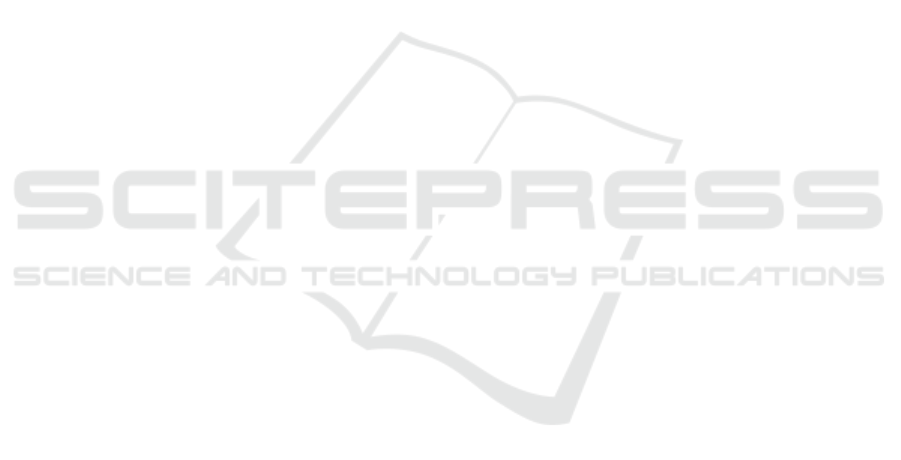
Peng, X., Wang, L., Wang, X., and Qiao, Y. (2016). Bag
of Visual Words and Fusion Methods for Action Re-
cognition: Comprehensive Study and Good Practice.
Computer Vision and Image Understanding, 150:109–
125.
Peng, X., Zou, C., Qiao, Y., and Peng, Q. (2014). Action
Recognition with Stacked Fisher Vectors. In Fleet, D.,
Pajdla, T., Schiele, B., and Tuytelaars, T., editors, Eu-
ropean Conference on Computer Vision, pages 581–
595, Cham. Springer International Publishing.
Perez, E. A., Mota, V. F., Maciel, L. M., Sad, D., and Vieira,
M. B. (2012). Combining Gradient Histograms using
Orientation Tensors for Human Action Recognition.
In 21st International Conference on Pattern Recogni-
tion, pages 3460–3463. IEEE.
Phan, H.-H., Vu, N.-S., Nguyen, V.-L., and Quoy, M.
(2016). Motion of Oriented Magnitudes Patterns for
Human Action Recognition. In International Sympo-
sium on Visual Computing, pages 168–177. Springer.
Ravanbakhsh, M., Mousavi, H., Rastegari, M., Murino,
V., and Davis, L. S. (2015). Action Recognition
with Image based CNN Features. arXiv preprint
arXiv:1512.03980.
Russakovsky, O., Deng, J., Su, H., Krause, J., Satheesh, S.,
Ma, S., Huang, Z., Karpathy, A., Khosla, A., Bern-
stein, M., Berg, A. C., and Fei-Fei, L. (2015). Ima-
geNet Large Scale Visual Recognition Challenge. In-
ternational Journal of Computer Vision, 115(3):211–
252.
Ryoo, M. S. and Matthies, L. (2016). First-Person Acti-
vity Recognition: Feature, Temporal Structure, and
Prediction. International Journal of Computer Vision,
119(3):307–328.
Shi, F., Laganiere, R., and Petriu, E. (2015). Gradient Boun-
dary Histograms for Action Recognition. In IEEE
Winter Conference on Applications of Computer Vi-
sion, pages 1107–1114.
Simonyan, K. and Zisserman, A. (2014a). Two-Stream
Convolutional Networks for Action Recognition in
Videos. In Advances in Neural Information Proces-
sing Systems, pages 568–576.
Simonyan, K. and Zisserman, A. (2014b). Two-Stream
Convolutional Networks for Action Recognition in
Videos. In Ghahramani, Z., Welling, M., Cortes, C.,
Lawrence, N., and Weinberger, K., editors, Advances
in Neural Information Processing Systems 27, pages
568–576. Curran Associates, Inc.
Soomro, K., Zamir, A. R., and Shah, M. (2012a). UCF101:
A Dataset of 101 Human Actions Classes from Videos
in the Wild. arXiv preprint arXiv:1212.0402.
Soomro, K., Zamir, A. R., and Shah, M. (2012b). UCF101:
A Dataset of 101 Human Actions Classes from Videos
in the Wild. arXiv preprint arXiv:1212.0402.
Szegedy, C., Vanhoucke, V., Ioffe, S., Shlens, J., and Wojna,
Z. (2016). Rethinking the Inception Architecture for
Computer Vision. In IEEE Conference on Computer
Vision and Pattern Recognition, pages 2818–2826.
Torres, B. S. and Pedrini, H. (2016). Detection of Com-
plex Video Events through Visual Rhythm. The Visual
Computer, pages 1–21.
Tran, A. and Cheong, L. F. (2017). Two-Stream Flow-
Guided Convolutional Attention Networks for Action
Recognition. In IEEE International Conference on
Computer Vision Workshops, pages 3110–3119.
Varol, G., Laptev, I., and Schmid, C. (2016). Long-Term
Temporal Convolutions for Action Recognition. arXiv
preprint arXiv:1604.04494.
Wang, H., Kläser, A., Schmid, C., and Liu, C.-L. (2011).
Action Recognition by Dense Trajectories. In IEEE
Conference on Computer Vision and Pattern Recogni-
tion, pages 3169–3176. IEEE.
Wang, H. and Schmid, C. (2013). Action Recognition with
Improved Trajectories. In International Conference
on Computer Vision, pages 3551–3558.
Wang, H., Yang, Y., Yang, E., and Deng, C. (2017a). Explo-
ring Hybrid Spatio-Temporal Convolutional Networks
for Human Action Recognition. Multimedia Tools and
Applications, 76(13):15065–15081.
Wang, L., Ge, L., Li, R., and Fang, Y. (2017b). Three-
stream CNNs for Action Recognition. Pattern Recog-
nition Letters, 92:33–40.
Wang, L., Ge, L., Li, R., and Fang, Y. (2017c). Three-
Stream CNNs for Action Recognition. Pattern Recog-
nition Letters, 92(Supplement C):33–40.
Wang, L., Qiao, Y., and Tang, X. (2015a). Action Recogni-
tion with Trajectory-Pooled Deep-Convolutional Des-
criptors. In Computer Vision and Pattern Recognition,
pages 4305–4314.
Wang, L., Xiong, Y., Wang, Z., and Qiao, Y. (2015b). To-
wards Good Practices for very Deep Two-Stream Con-
vnets. arXiv preprint arXiv:1507.02159.
Wang, L., Xiong, Y., Wang, Z., Qiao, Y., Lin, D., Tang,
X., and Van Gool, L. (2016a). Temporal Segment
Networks: Towards Good Practices for Deep Action
Recognition. In European Conference on Computer
Vision, pages 20–36. Springer.
Wang, X., Farhadi, A., and Gupta, A. (2016b). Actions
Transformations. In IEEE Conference on Computer
Vision and Pattern Recognition, pages 2658–2667.
Wang, Z., Bovik, A. C., Sheikh, H. R., and Simoncelli, E. P.
(2004). Image Quality Assessment: From Error Visi-
bility to Structural Similarity. IEEE Transactions on
Image Processing, 13(4):600–612.
Yeffet, L. and Wolf, L. (2009). Local Trinary Patterns for
Human Action Recognition. In IEEE 12th Internatio-
nal Conference on Computer Vision, pages 492–497.
IEEE.
Zeiler, M. D. (2012). ADADELTA: An Adaptive Learning
Rate Method. arXiv preprint arXiv:1212.5701.
Zhao, H., Gallo, O., Frosio, I., and Kautz, J. (2017a). Loss
Functions for Image Restoration with Neural Net-
works. IEEE Transactions on Computational Ima-
ging, 3(1):47–57.
Zhao, Y., Deng, B., Shen, C., Liu, Y., Lu, H., and Hua, X.-
S. (2017b). Spatio-Temporal Autoencoder for Video
Anomaly Detection. In ACM on Multimedia Confe-
rence, pages 1933–1941. ACM.
Zhu, Y., Lan, Z., Newsam, S., and Hauptmann, A. G.
(2017). Hidden Two-Stream Convolutional Net-
works for Action Recognition. arXiv preprint
arXiv:1704.00389.
Spatio-temporal Video Autoencoder for Human Action Recognition
123