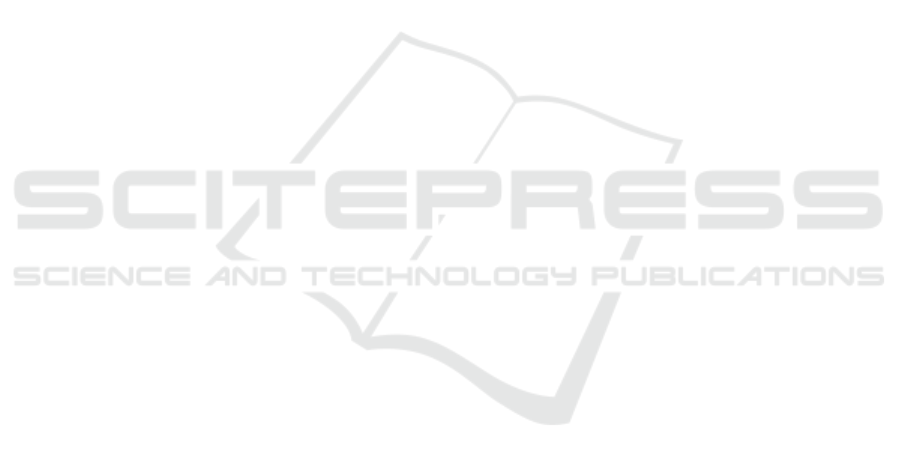
regularized hmax for visual recognition. PloS one,
9(1):e81813.
Krizhevsky, A., Sutskever, I., and Hinton, G. E. (2012).
Imagenet classification with deep convolutional neu-
ral networks. In Advances in neural information pro-
cessing systems, pages 1097–1105.
Lei, J., Guo, Z., and Wang, Y. (2017). Weakly supervised
image classification with coarse and fine labels. In
The 14th Conference on Computer and Robot Vision
(CRV), 2017, pages 231–239. IEEE.
Li, Y., Wu, W., Zhang, B., and Li, F. (2015). Enhan-
ced hmax model with feedforward feature learning for
multiclass categorization. Frontiers in computational
neuroscience, 9:123.
Riesenhuber, M. and Poggio, T. (1999). Hierarchical mo-
dels of object recognition in cortex. Nature neuros-
cience, 2(11):1019.
Ristin, M., Gall, J., Guillaumin, M., and Van Gool, L.
(2015). From categories to subcategories: large-scale
image classification with partial class label refine-
ment. In Proceedings of the IEEE conference on com-
puter vision and pattern recognition, pages 231–239.
Rosten, E. and Drummond, T. (2006). Machine learning for
high-speed corner detection. In European conference
on computer vision, pages 430–443. Springer.
Serre, T., Wolf, L., Bileschi, S., Riesenhuber, M., and Pog-
gio, T. (2007). Robust object recognition with cortex-
like mechanisms. IEEE Transactions on Pattern Ana-
lysis & Machine Intelligence, (3):411–426.
Singhal, N., Singhal, N., and Kalaichelvi, V. (2017). Image
classification using bag of visual words model with
fast and freak. In Electrical, Computer and Commu-
nication Technologies (ICECCT), 2017 Second Inter-
national Conference on, pages 1–5. IEEE.
Sivic, J. and Zisserman, A. (2003). Video google: A text
retrieval approach to object matching in videos. In
null, page 1470. IEEE.
Su, Y. and Jurie, F. (2012). Improving image classifica-
tion using semantic attributes. International journal
of computer vision, 100(1):59–77.
Szegedy, C., Vanhoucke, V., Ioffe, S., Shlens, J., and Wo-
jna, Z. (2016). Rethinking the inception architecture
for computer vision. In Proceedings of the IEEE con-
ference on computer vision and pattern recognition,
pages 2818–2826.
Theriault, C., Thome, N., and Cord, M. (2011). Hmax-
s: deep scale representation for biologically inspired
image categorization. In Image Processing (ICIP),
2011 18th IEEE International Conference on, pages
1261–1264. IEEE.
Theriault, C., Thome, N., and Cord, M. (2013). Extended
coding and pooling in the hmax model. IEEE Tran-
sactions on Image Processing, 22(2):764–777.
Wang, C. and Huang, K. (2015). How to use bag-of-words
model better for image classification. Image and Vi-
sion Computing, 38:65–74.
Wang, J., Yang, Y., Mao, J., Huang, Z., Huang, C., and Xu,
W. (2016a). Cnn-rnn: A unified framework for multi-
label image classification. In Proceedings of the IEEE
conference on computer vision and pattern recogni-
tion, pages 2285–2294.
Wang, R., Ding, K., Yang, J., and Xue, L. (2016b). A novel
method for image classification based on bag of visual
words. Journal of Visual Communication and Image
Representation, 40:24–33.
Wu, J., Yu, Y., Huang, C., and Yu, K. (2015). Deep multi-
ple instance learning for image classification and auto-
annotation. In Proceedings of the IEEE Conference
on Computer Vision and Pattern Recognition, pages
3460–3469.
Zhang, H.-Z., Lu, Y.-F., Kang, T.-K., and Lim, M.-T.
(2016). B-hmax: A fast binary biologically inspi-
red model for object recognition. Neurocomputing,
218:242–250.
VISAPP 2019 - 14th International Conference on Computer Vision Theory and Applications
134