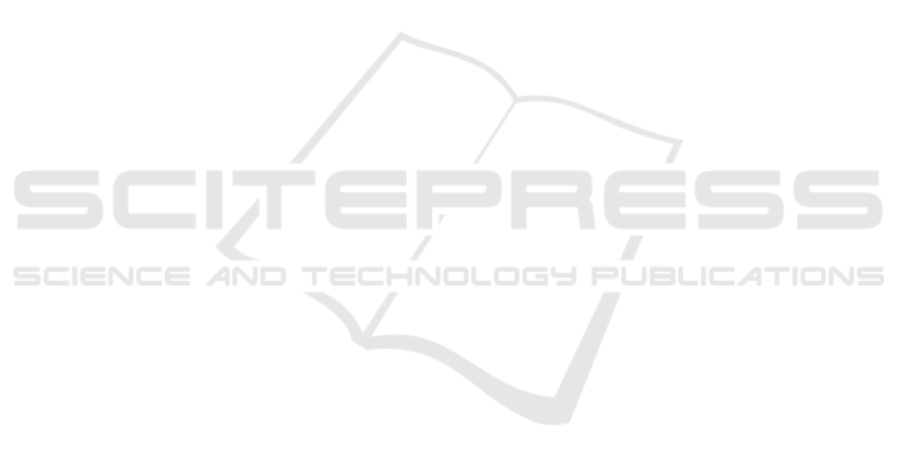
Cassidy, A. and Ekanayake, V. (2006). A biologically inspi-
red tactile sensor array utilizing phase-based computa-
tion. In Biomedical Circuits and Systems Conference,
2006. BioCAS 2006. IEEE, pages 45–48. IEEE.
Chen, H. T., Ng, K. T., Bermak, A., Law, M. K., and Mar-
tinez, D. (2011). Spike latency coding in biologically
inspired microelectronic nose. IEEE transactions on
biomedical circuits and systems, 5(2):160–168.
Chou, C.-N., Chung, K.-M., and Lu, C.-J. (2018). On the
algorithmic power of spiking neural networks. arXiv
preprint arXiv:1803.10375.
Diehl, P. U., Neil, D., Binas, J., Cook, M., Liu, S.-C., and
Pfeiffer, M. (2015). Fast-classifying, high-accuracy
spiking deep networks through weight and threshold
balancing. In Neural Networks (IJCNN), 2015 Inter-
national Joint Conference on, pages 1–8. IEEE.
Escobar, M.-J., Masson, G. S., Vieville, T., and Kornprobst,
P. (2009). Action recognition using a bio-inspired
feedforward spiking network. International Journal
of Computer Vision, 82(3):284.
Farabet, C., Paz, R., P
´
erez-Carrasco, J., Zamarre
˜
no,
C., Linares-Barranco, A., LeCun, Y., Culurciello,
E., Serrano-Gotarredona, T., and Linares-Barranco,
B. (2012). Comparison between frame-constrained
fix-pixel-value and frame-free spiking-dynamic-pixel
convnets for visual processing. Frontiers in neuros-
cience, 6:32.
Fu, J., Li, G., Qin, Y., and Freeman, W. J. (2007). A pattern
recognition method for electronic noses based on an
olfactory neural network. Sensors and Actuators B:
Chemical, 125(2):489–497.
Furber, S. B., Galluppi, F., Temple, S., and Plana, L. A.
(2014). The spinnaker project. Proceedings of the
IEEE, 102(5):652–665.
Gerstner, W. and Kistler, W. M. (2002). Spiking neuron
models: Single neurons, populations, plasticity. Cam-
bridge university press.
Girshick, R. (2015). Fast r-cnn. In Proceedings of the IEEE
international conference on computer vision, pages
1440–1448.
Heimberger, M., Horgan, J., Hughes, C., McDonald, J., and
Yogamani, S. (2017). Computer vision in automated
parking systems: Design, implementation and chal-
lenges. Image and Vision Computing, 68:88–101.
Hertz, J., Krogh, A., and Palmer, R. G. (1991). Intro-
duction to the theory of neural computation. Addison-
Wesley/Addison Wesley Longman.
Hinton, G. E., Sejnowski, T. J., and Poggio, T. A. (1999).
Unsupervised learning: foundations of neural compu-
tation. MIT press.
Hu, Y., Liu, H., Pfeiffer, M., and Delbruck, T. (2016). Dvs
benchmark datasets for object tracking, action recog-
nition, and object recognition. Frontiers in neuros-
cience, 10:405.
Hunsberger, E. and Eliasmith, C. (2015). Spiking
deep networks with lif neurons. arXiv preprint
arXiv:1510.08829.
Indiveri, G., Chicca, E., and Douglas, R. J. (2006). A vlsi
array of low-power spiking neurons and bistable syn-
apses with spike-timing dependent plasticity. IEEE
transactions on neural networks, 17(1).
Indiveri, G. and Fusi, S. (2007). Spike-based learning in
vlsi networks of integrate-and-fire neurons. In Circuits
and Systems, 2007. ISCAS 2007. IEEE International
Symposium on, pages 3371–3374. IEEE.
Lenero-Bardallo, J. A., Serrano-Gotarredona, T., and
Linares-Barranco, B. (2010). A signed spatial contrast
event spike retina chip. In Circuits and Systems (IS-
CAS), Proceedings of 2010 IEEE International Sym-
posium on, pages 2438–2441. IEEE.
Lichtsteiner, P., Posch, C., and Delbruck, T. (2008). A
128x128 120 db 15 microsec latency asynchronous
temporal contrast vision sensor. IEEE journal of solid-
state circuits, 43(2):566–576.
Maass, W. (1997). Networks of spiking neurons: the third
generation of neural network models. Neural net-
works, 10(9):1659–1671.
Maqueda, A. I., Loquercio, A., Gallego, G., Garcıa, N.,
and Scaramuzza, D. (2018). Event-based vision meets
deep learning on steering prediction for self-driving
cars. In Proceedings of the IEEE Conference on Com-
puter Vision and Pattern Recognition, pages 5419–
5427.
Ponulak, F. and Kasinski, A. (2011). Introduction to spi-
king neural networks: Information processing, lear-
ning and applications. Acta neurobiologiae experi-
mentalis, 71(4):409–433.
Redmon, J., Divvala, S., Girshick, R., and Farhadi, A.
(2016). You only look once: Unified, real-time object
detection. In Proceedings of the IEEE conference on
computer vision and pattern recognition, pages 779–
788.
Rueckauer, B., Lungu, I.-A., Hu, Y., Pfeiffer, M., and Liu,
S.-C. (2017). Conversion of continuous-valued deep
networks to efficient event-driven networks for image
classification. Frontiers in neuroscience, 11:682.
Russakovsky, O., Deng, J., Su, H., Krause, J., Satheesh, S.,
Ma, S., Huang, Z., Karpathy, A., Khosla, A., Bern-
stein, M., et al. (2015). Imagenet large scale visual
recognition challenge. International Journal of Com-
puter Vision, 115(3):211–252.
Sawada, J., Akopyan, F., Cassidy, A. S., Taba, B., De-
bole, M. V., Datta, P., Alvarez-Icaza, R., Amir, A.,
Arthur, J. V., Andreopoulos, A., et al. (2016). Tru-
enorth ecosystem for brain-inspired computing: sca-
lable systems, software, and applications. In Procee-
dings of the International Conference for High Perfor-
mance Computing, Networking, Storage and Analysis,
page 12. IEEE Press.
Sengupta, A., Ye, Y., Wang, R., Liu, C., and Roy,
K. (2018). Going deeper in spiking neural net-
works: Vgg and residual architectures. arXiv preprint
arXiv:1802.02627.
Siam, M., Elkerdawy, S., Jagersand, M., and Yogamani, S.
(2017). Deep semantic segmentation for automated
driving: Taxonomy, roadmap and challenges. In In-
telligent Transportation Systems (ITSC), 2017 IEEE
20th International Conference on, pages 1–8. IEEE.
VISAPP 2019 - 14th International Conference on Computer Vision Theory and Applications
554