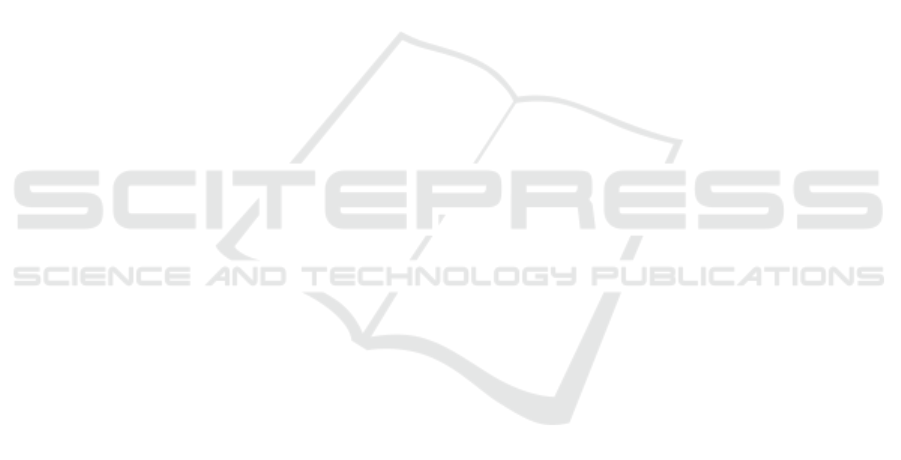
is able to assess cognitive workload similarly to
larger, more expensive, and more established devices.
ACKNOWLEDGEMENTS
This work was supported by NASA Contract Nos.
NNX15CJ17P and NNX16CJ08C.
REFERENCES
Ayaz, H., Shewokis, P. A., Bunce, S., Izzetoglu, K.,
Willems, B., & Onaral, B., 2012. Optical brain
monitoring for operator training and mental workload
assessment. NeuroImage, 59(1), 36–47.
https://doi.org/10.1016/j.neuroimage.2011.06.023.
Bracken, B. K., Elkin-Frankston, S., Palmon, N., Farry, M.,
& de B Frederick, B., 20170. A System to Monitor
Cognitive Workload in Naturalistic High-Motion
Environments.
Bracken, B., Romero, V., Guarino, S., & Pfautz, J., 2013.
Designing an Adaptive Approach for the Real-Time
Assessment and Augmentation of Performance of
Cyber Analyst Teams. In Proceedings of the Human
Factors and Ergonomics Society Annual Meeting (Vol.
57, pp. 124–128). SAGE Publications Sage CA: Los
Angeles, CA. Retrieved from http://journals.sagepub.
com/doi/abs/10.1177/1541931213571029.
Braver, T. S., Cohen, J. D., Nystrom, L. E., Jonides, J.,
Smith, E. E., & Noll, D. C., 1997. A parametric study
of prefrontal cortex involvement in human working
memory. Neuroimage, 5(1), 49–62.
Bunce, S. C., Izzetoglu, K., Ayaz, H., Shewokis, P.,
Izzetoglu, M., Pourrezaei, K., & Onaral, B., 2011.
Implementation of fNIRS for Monitoring Levels of
Expertise and Mental Workload. In D. D. Schmorrow
& C. M. Fidopiastis (Eds.), Foundations of Augmented
Cognition. Directing the Future of Adaptive Systems
(Vol. 6780, pp. 13–22). Berlin, Heidelberg: Springer
Berlin Heidelberg. https://doi.org/10.1007/978-3-642-
21852-1_2.
Cao, A., Chintamani, K. K., Pandya, A. K., & Ellis, R. D.,
2009. NASA TLX: Software for assessing subjective
mental workload. Behavior Research Methods, 41(1),
113–117.
Comstock, J. R., & Arnegard, R. J., 1992. MAT: Multi-
Attribute Task Battery for Human Operator Workload
and Strategic Behavior Research. NASA Technical
Memorandum, (January).
Hart, S. G., & Staveland, L. E., 1988. Development of
NASA-TLX (Task Load Index): Results of empirical
and theoretical research. Advances in Psychology, 52,
139–183.
Kirchner, W. K., 1958. Age differences in short-term
retention of rapidly changing information. Journal of
Experimental Psychology, 55(4), 352.
Leather, C., Palmon, N., Bracken, B.K., 2018. Continuous
workload assessment and combined metrics of
performance on the multi-attribute task battery.
Presentation at the International Conference on
Applied Human Facotros and Ergonomics (AHFE)I,
July 2018.
Markey, A., Chin, A., Vanepps, E. M., & Loewenstein, G.,
2014. Identifying a reliable boredom induction.
Perceptual and Motor Skills, 119(1), 237–253.
McKendrick, R., Parasuraman, R., & Ayaz, H., 2015.
Wearable functional near infrared spectroscopy
(fNIRS) and transcranial direct current stimulation
(tDCS): expanding vistas for neurocognitive
augmentation. Frontiers in Systems Neuroscience, 27.
https://doi.org/10.3389/fnsys.2015.00027.
Nelson, J., 2016. The Development of a Human Operator
Informatic Model (HOIM) Incorporating the Effects of
Non-Invasive Brain Stimulation on Information
Processing while Performing Multi-Attribute Task
Battery (MATB).
Santiago-Espada, Y., Myer, R. R., Latorella, K. A., &
Comstock Jr, J. R., 2011. The multi-attribute task
battery ii (matb-ii) software for human performance and
workload research: A user’s guide.
Strangman G, Franceschini MA, Boas DA, 2003. Factors
affecting the accuracy of near-infrared spectroscopy
concentration calculations for focal changes in
oxygenation parameters. Neuroimage 18:865-879.
Strangman GE, Ivkovic V, Zhang Q, 2018. Wearable brain
imaging with multimodal physiological monitoring. J
Appl Physiol (1985) 124:564-572.
Validation of the fNIRS Pioneer
TM
, a Portable, Durable, Rugged functional Near-Infrared Spectroscopy (fNIRS) Device
531