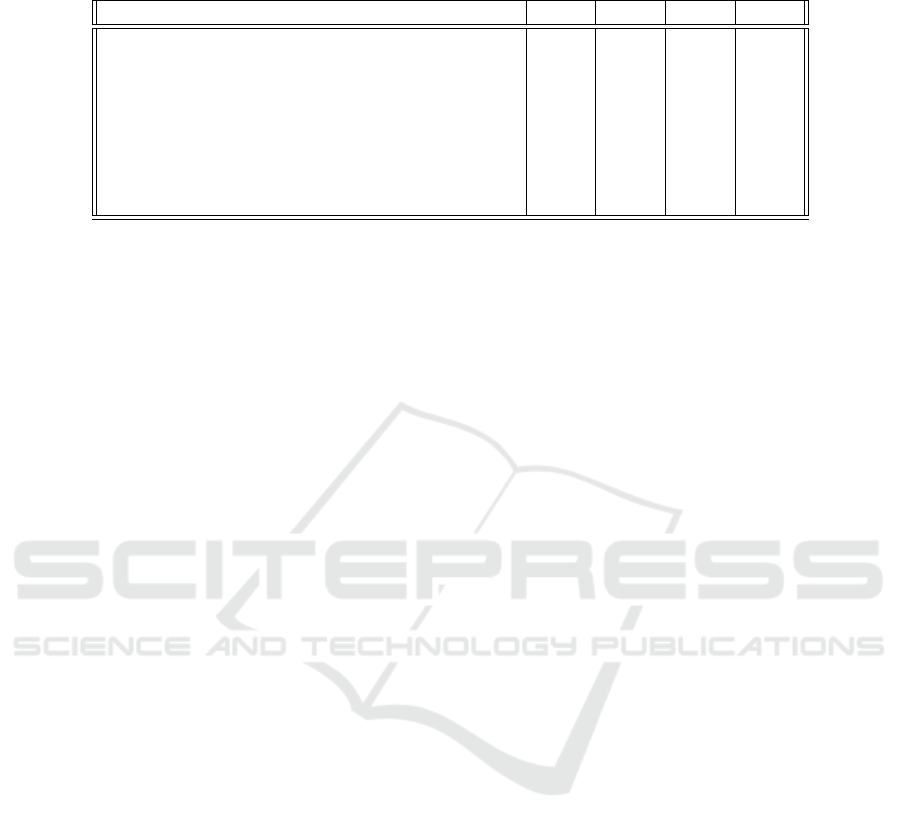
Table 1: Nuclei detection results with UCSB-58 and CRC-100 histopathological image data sets by using Tiny-CNN and
FCM semiautomatic, automatic method, and, combined FCM semiautomatic with Tiny-CNN.
Data Set TPR PPV F-M DSC
UCSB-58 set with Tiny-CNN 0.964 0.996 0.979 0.979
UCSB-58 set with FCM semiautomatic 0.881 0.994 0.933 0.933
UCSB-58 set with FCM automatic 0.828 0.994 0.899 0.899
CRC-100 set with Tiny-CNN 0.621 0.989 0.769 0.769
CRC-100 set with FCM semiautomatic 0.466 0.994 0.617 0.617
CRC-100 set with FCM automatic 0.451 0.994 0.528 0.528
UCSB-58 set with combined FCM and Tiny-CNN 0.863 0.994 0.934 0.934
CRC-100 set with combined FCM and Tiny-CNN 0.547 0.967 0.741 0.741
Hatipoglu, N. and Bilgin, G. (2017). Cell segmentation
in histopathological images with deep learning algo-
rithms by utilizing spatial relationships. Medical &
Biological Engineering & Computing, 55(10):1829–
1848.
John, J., Nair, M. S., Kumar, P. A., and Wilscy, M. (2016).
A novel approach for detection and delineation of cell
nuclei using feature similarity index measure. Biocy-
bernetics and Biomedical Engineering, 36(1):76–88.
Kainz, P., Urschler, M., Schulter, S., Wohlhart, P., and Lep-
etit, V. (2015). You should use regression to detect
cells. In International Conference on Medical Im-
age Computing and Computer-Assisted Intervention,
pages 276–283. Springer.
Khoshdeli, M., Cong, R., and Parvin, B. (2017). Detection
of nuclei in H&E stained sections using convolutional
neural networks. In IEEE EMBS Int. Conf. on Biomed-
ical & Health Informatics, BHI’17, pages 105–108.
Kong, H., Akakin, H. C., and Sarma, S. E. (2013). A gen-
eralized laplacian of gaussian filter for blob detection
and its applications. IEEE Transactions on Cybernet-
ics, 43(6):1719–1733.
LeCun, Y., Kavukcuoglu, K., Farabet, C., et al. (2010).
Convolutional networks and applications in vision. In
ISCAS, volume 2010, pages 253–256.
Moita, A. S., Jacinto, F., and Moreira, A. L. N. (2018).
Design, test and fabrication of a droplet based mi-
crofluidic device for clinical diagnostics. In Proceed-
ings of the 11th Int. Joint Conference on Biomed-
ical Engineering Systems and Technologies-Volume
1: BIODEVICES, BIOSTEC’18, pages 88–95. IN-
STICC, SciTePress.
Phung, S. L. and Bouzerdoum, A. (2009). Matlab library
for convolutional neural networks. University of Wol-
longong, Tech. Rep., URL: http://www. elec. uow. edu.
au/staff/sphung.
Sirinukunwattana, K., Raza, S. E. A., Tsang, Y.-W., Snead,
D. R., Cree, I. A., and Rajpoot, N. M. (2016). Local-
ity sensitive deep learning for detection and classifi-
cation of nuclei in routine colon cancer histology im-
ages. IEEE Trans. on Medical Imaging, 35(5):1196–
1206.
Sornapudi, S., Stanley, R. J., Stoecker, W. V., Almubarak,
H., Long, R., Antani, S., Thoma, G., Zuna, R., and
Frazier, S. R. (2018). Deep learning nuclei detection
in digitized histology images by superpixels. Journal
of Pathology Informatics, 9.
Suganya, R. and Shanthi, R. (2012). Fuzzy c-means
algorithm-a review. Int. Journal of Scientific and Re-
search Publications, 2(11):1.
Vedaldi, A. and Lenc, K. (2015). Matconvnet: Convolu-
tional neural networks for matlab. In Proceedings of
the 23rd ACM International Conference on Multime-
dia, pages 689–692.
Vink, J. P., Van Leeuwen, M., Van Deurzen, C., and
De Haan, G. (2013). Efficient nucleus detector
in histopathology images. Journal of Microscopy,
249(2):124–135.
Xu, H., Lu, C., Berendt, R., Jha, N., and Mandal, M.
(2017). Automatic nuclei detection based on gener-
alized laplacian of gaussian filters. IEEE Journal of
Biomedical and Health Informatics, 21(3):826–837.
Xu, J., Xiang, L., Liu, Q., Gilmore, H., Wu, J., Tang, J.,
and Madabhushi, A. (2016). Stacked sparse autoen-
coder (SSAE) for nuclei detection on breast cancer
histopathology images. IEEE Transactions on Med-
ical Imaging, 35(1):119–130.
BIOSIGNALS 2019 - 12th International Conference on Bio-inspired Systems and Signal Processing
200