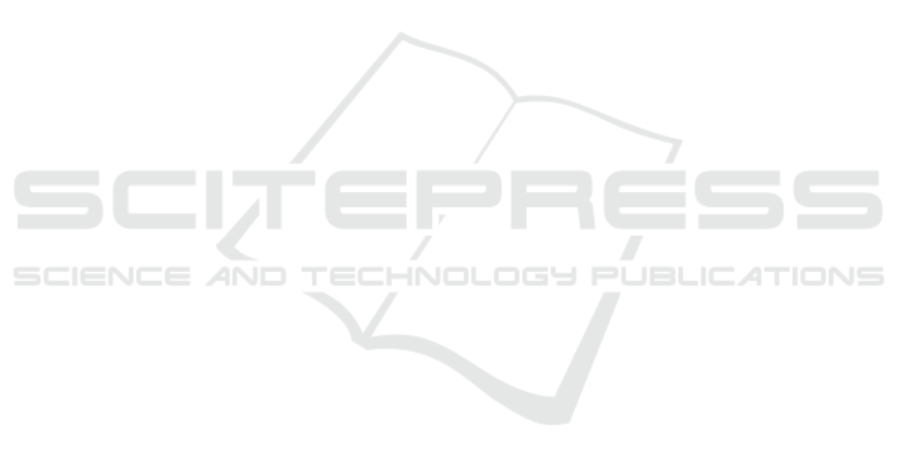
Toselli 29, Pisa. We would like to thank Giulia Fe-
riani and Sharon Gruttadauria for the contribution to
the realization of the dataset.
REFERENCES
Alonzo-Proulx, O., Mawdsley, G. E., Patrie, J. T., Yaffe,
M. J., and Harvey, J. A. (2015). Reliability of au-
tomated breast density measurements. Radiology,
275(2):366–376.
Chollet, F. (2018). Keras documentation.
Ciatto, S., Houssami, N., Apruzzese, A., Bassetti, E., Bran-
cato, B., Carozzi, F., Catarzi, S., Lamberini, M., Mar-
celli, G., Pellizzoni, R., Pesce, B., Risso, G., Russo,
F., and Scorsolini, A. (2005). Categorizing breast
mammographic density: intra- and interobserver re-
producibility of BI-RADS density categories. The
breast, 14(4):269–275.
D. R. Dance, S. Christofides, I.D. McLean, and A.D.A.
Maidment, K.H. Ng (2014). Diagnostic Radiology
Physics: A Handbook for Teachers and Students.
Ekpo, E. U., Ujong, U. P., Mello-Thoms, C., and McEn-
tee, M. F. (2016). Assessment of Interradiologist
Agreement Regarding Mammographic Breast Den-
sity Classification Using the Fifth Edition of the BI-
RADS Atlas. American Journal of Roentgenology,
206(5):1119–1123.
Euratom (2013). Council directive 2013/59/euratom
of 5 december 2013 laying down basic safety
standards for protection against the dangers aris-
ing from exposure to ionising radiation, and re-
pealing directives 89/618/euratom, 90/641/euratom,
96/29/euratom, 97/43/euratom and 2003/122/eu-
ratom. page 73.
Fonseca, P., Casta
˜
neda, B., Valenzuela, R., and Wainer,
J. (2017). Breast density classification with convo-
lutional neural networks. In Beltr
´
an-Casta
˜
n
´
on, C.,
Nystr
¨
om, I., and Famili, F., editors, Progress in Pat-
tern Recognition, Image Analysis, Computer Vision,
and Applications, volume 10125, pages 101–108.
Springer International Publishing.
He, K., Zhang, X., Ren, S., and Sun, J. (2015). Deep resid-
ual learning for image recognition. Conference on
Computer Vision and Pattern Recognition (CVPR).
International Agency for Research on Cancer (2018).
http://gco.iarc.fr/today/home.
Ioffe, S. and Szegedy, C. (2015). Batch normalization: Ac-
celerating deep network training by reducing internal
covariate shift. arXiv:1502.03167.
Krishnan, K., Baglietto, L., Stone, J., Simpson, J. A., Sev-
eri, G., Evans, C. F., MacInnis, R. J., Giles, G. G.,
Apicella, C., and Hopper, J. L. (2017). Longitudinal
study of mammographic density measures that predict
breast cancer risk. 26(4):651–660.
Loberg, M., Lousdal, M. L., Bretthauer, M., and Kalager,
M. (2015). Benefits and harms of mammography
screening. Breast Cancer Res. 2015;17:63., 17(1).
McCormack, V. A. (2006). Breast density and parenchy-
mal patterns as markers of breast cancer risk: A meta-
analysis. Cancer Epidemiology Biomarkers & Pre-
vention, 15(6):1159–1169.
Miglioretti, D. L., Lange, J., van den Broek, J. J., Lee,
C. I., van Ravesteyn, N. T., Ritley, D., Kerlikowske,
K., Fenton, J. J., Melnikow, J., de Koning, H. J., and
Hubbard, R. A. (2016). Radiation-induced breast can-
cer incidence and mortality from digital mammogra-
phy screening: A modeling study. Annals of Internal
Medicine, 164(4):205.
Sickles, E., D’Orsi, C., and Bassett, L. e. a. (2013). ACR
BI-RADS
R
atlas, breast imaging reporting and data
system.
Sottocornola, C., Traino, A., Barca, P., Aringhieri, G.,
Marini, C., Retico, A., Caramella, D., and Fantacci,
M. E. (2018). Evaluation of dosimetric properties in
full field digital mammography (ffdm) - development
of a new dose index. In Proceedings of the 11th Inter-
national Joint Conference on Biomedical Engineering
Systems and Technologies - Volume 1: BIODEVICES,,
pages 212–217. INSTICC, SciTePress.
Traino, A. C., Sottocornola, C., Barca, P., Marini, C.,
Aringhieri, G., Caramella, D., and Fantacci, M. E.
(2017). Average absorbed breast dose in mammogra-
phy: a new possible dose index matching the require-
ments of the european directive 2013/59/EURATOM.
European Radiology Experimental, 1(1).
Wu, N., Geras, K. J., Shen, Y., Su, J., Kim, S. G., Kim,
E., Wolfson, S., Moy, L., and Cho, K. (2017). Breast
density classification with deep convolutional neural
networks. arXiv:1711.03674.
Residual Convolutional Neural Networks for Breast Density Classification
263