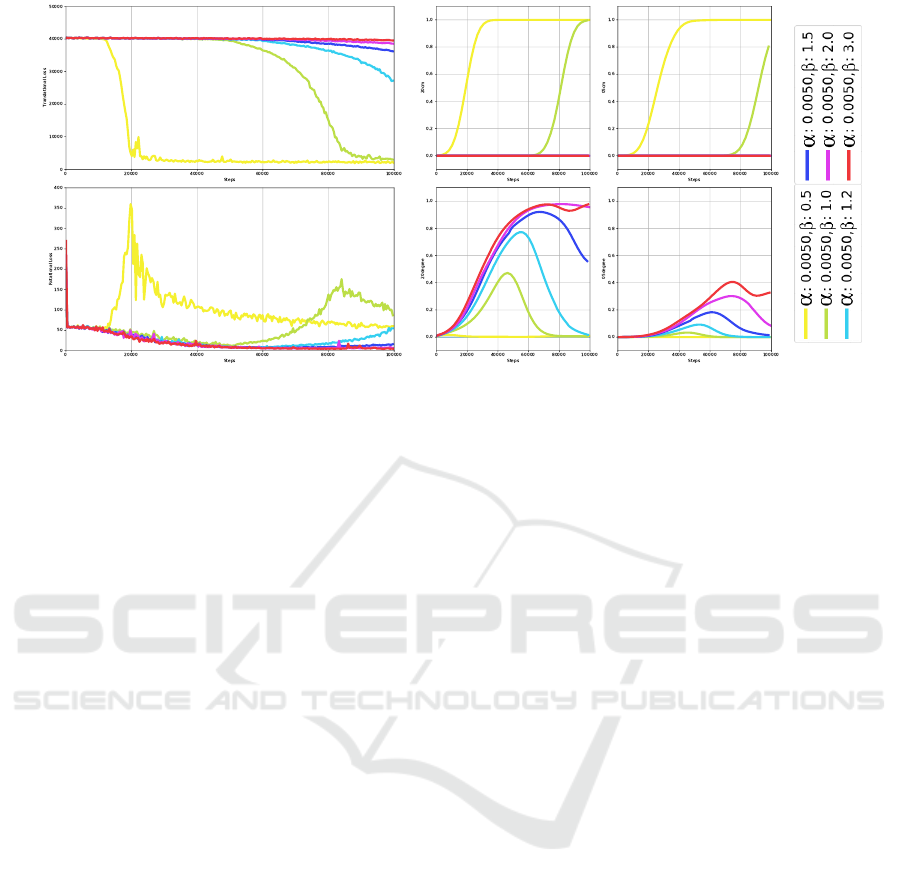
(a) Translation and rotation losses (b) Translation and rotation accuracies (c) Legend
Figure 5: First row: translation, second row: rotation. (a) Euclidean loss over training steps for α = 0.005 and various values
of β. (b) Accuracy at τ = 20 and τ = 5 over training steps for α = 0.005 and various values of β.
Crivellaro, A., Rad, M., Verdie, Y., Yi, K., Fua, P., and Le-
petit, V. (2015a). A novel representation of parts for
accurate 3d object detection and tracking in monocu-
lar images. In 2015 IEEE International Conference on
Computer Vision (ICCV), pages 4391–4399.
Crivellaro, A., Rad, M., Verdie, Y., Yi, K. M., Fua, P., and
Lepetit, V. (2015b). A novel representation of parts
for accurate 3d object detection and tracking in mo-
nocular images. In Proceedings of the 2015 IEEE In-
ternational Conference on Computer Vision (ICCV),
ICCV ’15, pages 4391–4399, Washington, DC, USA.
IEEE Computer Society.
Didier, J.-Y., Roussel, D., Mallem, M., Otmane, S., Naudet,
S., Pham, Q.-C., Bourgeois, S., M
´
egard, C., Leroux,
C., and Hocquard, A. (2005). AMRA: Augmented re-
ality assistance for train maintenance tasks. In Works-
hop Industrial Augmented Reality, 4th ACM/IEEE In-
ternational Symposium on Mixed and Augmented Re-
ality (ISMAR 2005), page (Elect. Proc.), Vienna, Au-
stria.
Do, T., Cai, M., Pham, T., and Reid, I. D. (2018). Deep-
6DPose: Recovering 6D object pose from a single
RGB image. CoRR, abs/1802.10367.
Geiger, A., Lenz, P., and Urtasun, R. (2012). Are we ready
for autonomous driving? the KITTI vision benchmark
suite. In 2012 IEEE Conference on Computer Vision
and Pattern Recognition, pages 3354–3361.
H
¨
aming, K. and Peters, G. (2010). The structure-from-
motion reconstruction pipeline a survey with focus
on short image sequences. Kybernetika, 5.
Hinton, G., Krizhevsky, A., and Wang, S. (2011). Transfor-
ming auto-encoders. In Honkela, T., Duch, W., Giro-
lami, M., and Kaski, S., editors, Artificial Neural Net-
works and Machine Learning – ICANN 2011, pages
44–51. Springer Berlin Heidelberg.
Hoda
ˇ
n, T., Haluza, P., Obdr
ˇ
z
´
alek,
ˇ
S., Matas, J., Lourakis,
M., and Zabulis, X. (2017). T-LESS: An RGB-D da-
taset for 6D pose estimation of texture-less objects.
IEEE Winter Conference on Applications of Compu-
ter Vision (WACV).
IKEA (2017). Place app.
Kendall, A., Grimes, M., and Cipolla, R. (2015). PoseNet:
A convolutional network for real-time 6-DOF camera
relocalization. CoRR, abs/1505.07427.
Krizhevsky, A., Sutskever, I., and Hinton, G. E. (2012).
Imagenet classification with deep convolutional neu-
ral networks. In Proceedings of the 25th Internatio-
nal Conference on Neural Information Processing Sy-
stems - Volume 1, NIPS’12, pages 1097–1105, USA.
Curran Associates Inc.
Le Cun, Y., Jackel, L. D., Boser, B., Denker, J. S., Graf,
H. P., Guyon, I., Henderson, D., Howard, R. E.,
and Hubbard, W. (1990). Handwritten digit recog-
nition: Applications of neural net chips and automa-
tic learning. In Souli
´
e, F. F. and H
´
erault, J., editors,
Neurocomputing, pages 303–318, Berlin, Heidelberg.
”Springer Berlin Heidelberg.
Li, Y., Wang, G., Ji, X., Xiang, Y., and Fox, D. (2018). Dee-
pIM: Deep iterative matching for 6D pose estimation.
CoRR, abs/1804.00175.
Lowe, D. G. (1999). Object recognition from local scale-
invariant features. In Proceedings of the Seventh
IEEE International Conference on Computer Vision,
volume 2, pages 1150–1157 vol.2.
Microsoft Hololens
R
(2015-2017). Webpage.
Rad, M. and Lepetit, V. (2017). BB8: A scalable, accurate,
robust to partial occlusion method for predicting the
3d poses of challenging objects without using depth.
2017 IEEE International Conference on Computer Vi-
sion (ICCV), pages 3848–3856.
Ronneberger, O., Fischer, P., and Brox, T. (2015). U-
Net: Convolutional networks for biomedical image
segmentation. CoRR, abs/1505.04597.
Sabour, S., Frosst, N., and Hinton, G. (2017). Dynamic
routing between capsules. CoRR, abs/1710.09829.
VISAPP 2019 - 14th International Conference on Computer Vision Theory and Applications
596