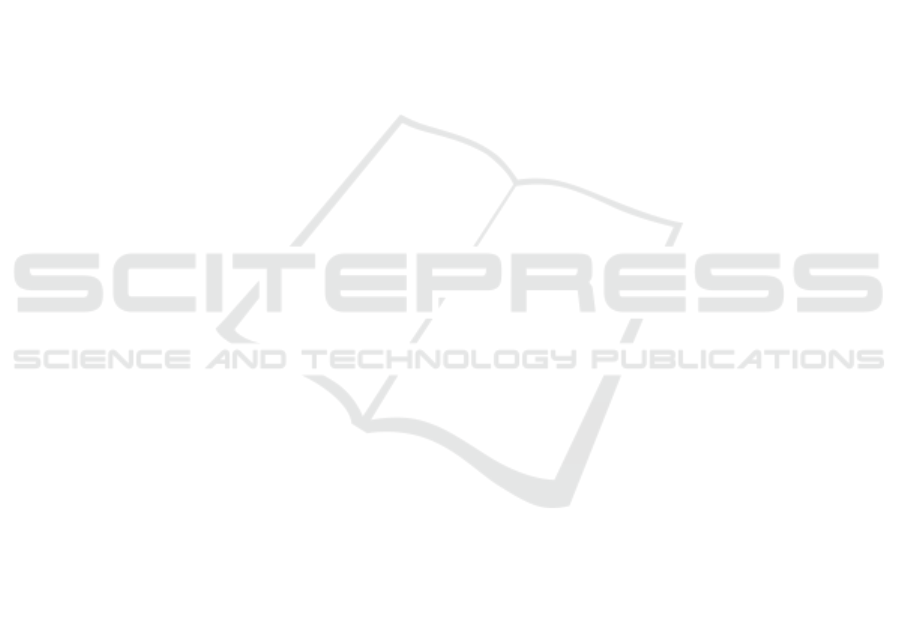
REFERENCES
Adler A., Gaburro R., WRB Lionheart, “Electrical
Impedance Tomography”, in Handbook of
Mathematical Methods in Imaging, 2nd ed O Scherzer
(Ed), Springer, 2016.
Akar S.A., Kara S., Agambayev S., Bilgic V. (2015)
Nonlinear analysis of EEG in major depression with
fractal dimensions. 37th Annual International
Conference of the IEEE on Engineering in Medicine
and Biology Society: 7410–7413. DOI:
10.1109/EMBC.2015.7320104.
Betelin, V.B., Eskov, V.M., Galkin, V.A. et al. (2017)
Stochastic volatility in the dynamics of complex
homeostatic systemsDokl. Math. 95: 92. DOI:
10.1134/S1064562417010240.
Caprioli, J., Coleman, A. L. (2010) Blood Pressure,
Perfusion Pressure, and Glaucoma. Am. J.
Ophthalmol. 149, 704–712.
Calvo, P. et al. (2012) Predictive value of retrobulbar
blood flow velocities in glaucoma suspects. Investig.
Ophthalmol. Vis. Sci. 53, 3875–3884.
Charlton P.H., Bonnici T., Tarassenko L., et al. (2017)
Extraction of respiratory signals from the
electrocardiogram and photoplethysmogram: Technical
and physiological determinants. Physiological
Measurement 38(5): 669-690. DOI: 10.1088/1361-
6579/aa670e.
Cherecheanu A.P., Garhofer G., Schmidl D., Werkmeister
R., Schmetterer L. Ocular perfusion pressure and ocular
blood flow in glaucoma. CurrOpinPharmacol. 2013;
13: 36-42. DOI: 10.1016/j.coph.2012. 09.003.
Elhaj, F., Salim, N., Harris, A., Swee, T. and Ahmed, T.
(2016). Arrhythmia recognition and classification using
combined linear and nonlinear features of ECG signals.
Computer Methods and Programs in Biomedicine, 127,
pp.52-63. DOI 10.1016/j.cmpb.2015.12.024.
Gracia J., Seppa V-P., Pelkonen A., Kotaniemi-Syrjanen A.
et al. (2017) Nonlinear Local Projection Filter for
Impedance Pneumography. European Medical
andBiological Engineering Conference 65: 306-309.
DOI: 10.1007/978-981-10-5122-7_77.
Kiseleva A., Luzhnov P., Dyachenko A. and Semenov Y.
(2018). Rheography and Spirography Signal Analysis
by Method of Nonlinear Dynamics.In Proceedings of
the 11th International Joint Conference on Biomedical
Engineering Systems and Technologies - Volume 1:
BIODEVICES, ISBN 978-989-758-277-6, pages 136-
140. DOI: 10.5220/0006579301360140.
Lazarenko V.I., Komarovskikh E.N. Results of the
examination of hemodynamics of the eye and brain in
patients with primary
open-angle glaucoma. Vestnik Oftal’mologii 120(1),
32–36 (2004).
Luzhnov P.V., Shamaev D.M., Iomdina E.N., Markosyan
G.A., Tarutta E.P., Sianosyan A.A. (2017) Using
quantitative parameters of ocular blood filling with
transpalpebralrheoophthalmography. In: Eskola H.,
Väisänen O., Viik J., Hyttinen J. (editors). EMBEC &
NBC 2017. IFMBE Proceedings, vol. 65; p. 37–40,
https://doi. org/10.1007/978-981-10-5122-7_10.
Luzhnov P.V., Shamaev D.M., Iomdina E.N., Tarutta E.P.,
Markosyan G.A., Shamkina L.A., Sianosyan A.A.
(2015) Transpalpebraltetrapolarreoophtalmography in
the assessment of parameters of the eye blood
circulatory system.
VestnikRossiiskoiakademiimeditsinskikhnauk; 70(3):
372–377, https://doi.org/10.15690/vramn.v70i3.1336.
Luzhnov P. V., Shamaev D. M., Kiseleva A. A., Iomdina E.
N. (2018), Analyzing rheoophthalmic signals in
glaucoma by nonlinear dynamics methods. IFMBE
Proceedings 68/2: pp.827-831. DOI: 10.1007/978-981-
10-9038-7_152.
Maiorov O. Yu., Glukhov A. B., Prognimak A. B.,
Fenchenko V. N. (2007) Realization of the method of
displacement by estimating the sizes of the axes of the
attractor of the dynamic brain system. - Proceedings of
the Institute of Cybernetics. - K., p. 153, pp. 5–12.
Palit K., Mukherjee S., Bhattacharya D.K. (2013) A high
dimensional delay selection for the reconstruction of
proper phase space with cross auto-
correlation.Neurocomputing 113: 49-
57.https://doi.org/10.1016/j.neucom.2013.01.034.
Palit K., Mukherjee S. (2011) Generalized autocorrelation
and its application in attractorreconstruction. Bull. Pure
Appl. Math. 5(2): 218–230.
Quigley, H. A., Broman, A. T. (2006) Number of people
with glaucoma worldwide. Br. J. Ophthalmol. 90, 262–
267.
Shamaev D. M., Luzhnov P. V., Pika T. O., Iomdina E. N.,
Kleyman A. P., Sianosyan A. A. (2016), Applying
transpalpebral rheoophthalmography to monitor
effectiveness of the treatment of patients with
glaucoma”. International Journal of Biomedicine 6(4):
pp.287–289. DOI: 10.21103/Article6(4)_OA8.
Strogatz H., S. (2014). Nonlinear Dynamics and Chaos.
With Applications to Physics, Biology, Chemistry, and
Engineering. 2nd ed. Boca Raton, p.532.
Takens F. Detecting strange attractors in turbulence / In
Rand D.A. and Young L.S. // Dynamical Systems and
Turbulence, Lecture Notes in Mathematics. 1981. V.
898. Р. 366–381.
Venkataraman, S. T., Flanagan, J. G. & Hudson, C.
(2010) Vascular Reactivity of Optic Nerve Head and
Retinal Blood Vessels in Glaucoma- A
Review. Microcirculation 17, 568–581.
Webber C. L., Sbilut J. P. Dynamic Assessment of
Physiological Systems and States Using Rrecurrence
Plot Strategies.// J. Appl. Physiol., 1994, 76, p.965–
993.
BIODEVICES 2019 - 12th International Conference on Biomedical Electronics and Devices
80