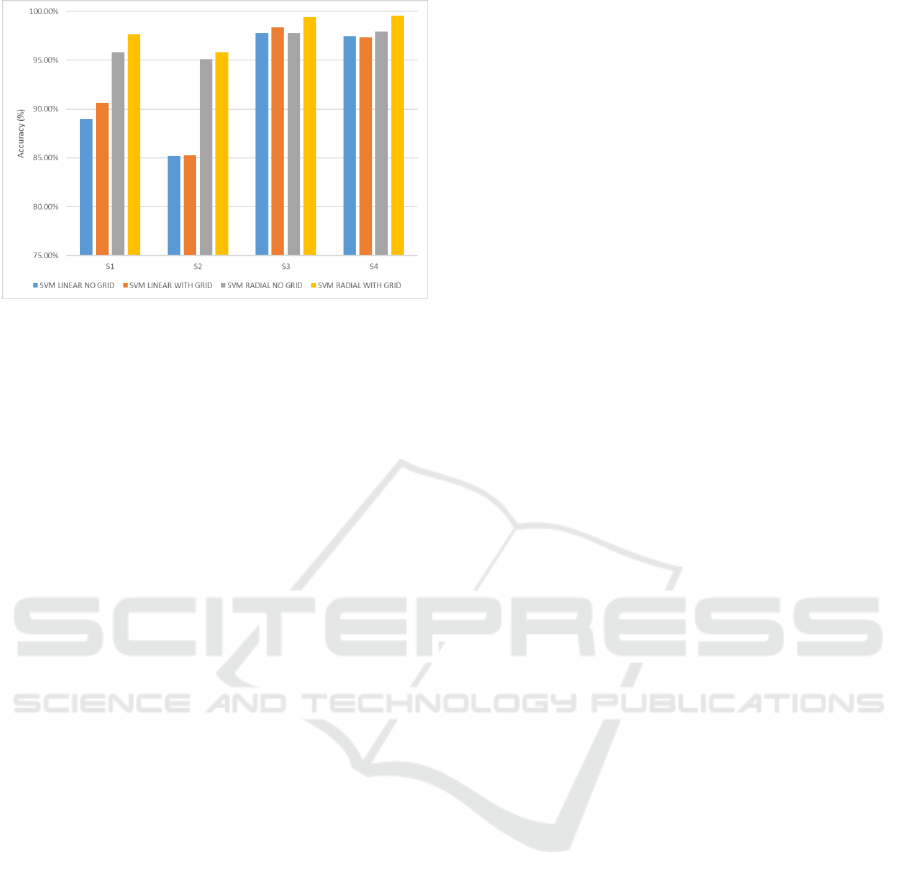
Figure 11: Performance comparison of four subjects using
10-fold cross-validation and SVM classifier without using
components from PCA.
3 CONCLUSIONS
This paper has presented the method of capturing on
breathing activities data from image depth of
Microsoft Kinect v2. This method is the noninvasive
mechanism to estimate the activities of the subject
from breathing activities monitoring. Those data were
used to calculate the mean depth value on Thorax area
and were displayed on time series signal. FFT had
been applied to do the feature extraction from time
series into numeric values. PCA is optionally used for
feature reduction on this classification, but the result
exposed that the highest accuracy was achieved
without using PCA components. As a result, we have
seen that, feature reduction using PCA is not effective
on time series signal in our study. Besides, the process
had carried out the clustering using non-parametric
density estimation, and the supervised machine
learning, classification, the algorithm had been
implemented by doing 10-fold cross-validation and
using SVM classifier for all four subjects. It has been
shown the SVM radial with the grid is the most
efficient classifier with the highest accuracy for all
the subjects over 99%. The result obtained is
promising to predict activities from breathing.
However, further work is required, especially for
feature selection in order to get better classification
results for a larger dataset.
ACKNOWLEDGEMENTS
The first author would like to gratefully acknowledge
the Indonesian Endowment Fund for Education
(LPDP) and The Directorate General of Higher Edu-
cation (DIKTI) for providing BUDI-LN scholarship.
In this research, the super-computing resource
was provided by Human Genome Center, the Institute
of Medical Science, the University of Tokyo.
Additional computation time was provided by the
super computer system in Research Organization of
Information and Systems (ROIS), National Institute
of Genetics (NIG). This work was supported by JSPS
KAKENHI Grant Number JP18K11525.
REFERENCES
Al-Khalidi, F.Q, R., Saatchi, D., Burke, Elphick., Tan, S.,
2011. Respiration Rate Monitoring Methods: A
Review. In International Journal of Pediatric
Pulmonolog.
Aliverti, A., Dellaca, R.L., Pelosi, R., Chiumello, D.,
Pedotti, A. and Gatinoni, L., 2000. Opto-Electronic
Plethysmography in Intensive Care Patients. In
American Journal of Respiratory and Critical Care
Medicine.
Aoki, H., Koshiji, K., Nakamura, H., Takemura, Y. and
Nakajima, M., 2005. Study On Respiration Monitoring
Method Using Near-Infrared Multipleslit-Lights
Projection. In Proceeding of IEEE International
Symposium on Micro-NanoMechatronics and Human
Science.
Azzalini, A. and Torelli, N., 2007. Clustering via
Nonparametric Density Estimation. In Statistics and
Computing.
Cala, S.J., Kenyon, C.M. and Ferrigno, G., 1996. Chest
Wall and Lung Volume Estimation by Optical
Reflectance Motion Analysis. In Journal of Applied
Physiology.
Corbishley, P., Rodriguez-Villegas, E., 2008. Breathing
Detection: Towards a Miniaturized, Wearable, Battery-
Operated Monitoring System. In IEEE Transactions on
Biomedical Engineering.
Devis, D., Gilberto, G., Guido, L., Massimiliano, P., Carlo,
A., Sergio, B., Gianna, C., Walter, C., Massimo, M. and
Juri, L.D., 2009. Non-Contact Detection of Breathing
Using a Microwave Sensor. In Sensors.
Faisal, M.R., Abapihi, B., Nguyen, N.G., Purnama, B.,
Delimayanti, M.K., Phan, D., Lumbanraja, F.R., Kubo,
M., Satou, K., 2018. Improving Protein Sequence
Classification Performance Using Adjacend and
Overlapped Segments on Existing Protein Descriptors.
In Journal Biomedical Science and Engineering.
Groote, A.D., Verbandt, Y., Paiva, Mand Mathys, P., 2000.
Measurement of Thoracoabdominal Asynchrony:
Importance of Sensor Sensitivity To Cross-Section
Deformations. In Journal of Applied Physiology.
Harper, V.P., Pasterkamp, H., Kiyokawa, H. and Wodicka,
G.R., 2003. Modeling and Measurement of Flow
Effects On Tracheal Sounds. In IEEE Transactions on
Biomedical Engineering.
BIOINFORMATICS 2019 - 10th International Conference on Bioinformatics Models, Methods and Algorithms
268