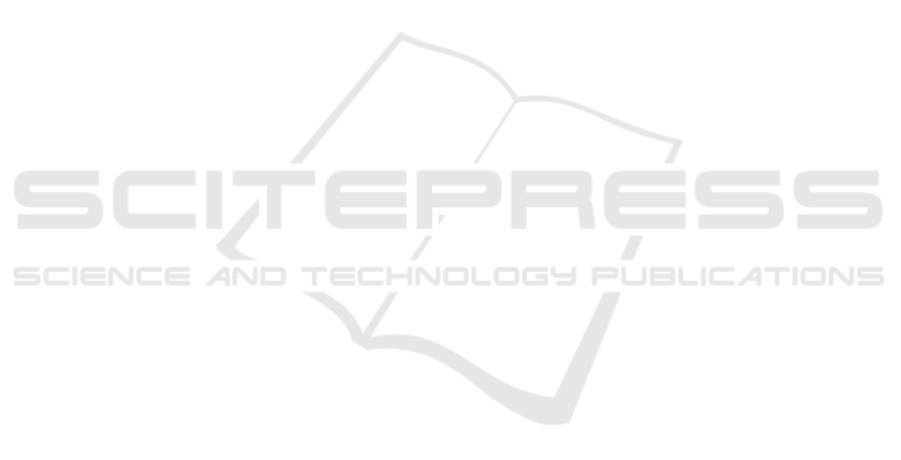
Doumanoglou, A., Kouskouridas, R., Malassiotis, S., and
Kim, T.-K. (2016). Recovering 6d object pose and
predicting next-best-view in the crowd. In Internati-
onal Conference on Computer Vision and Pattern Re-
cognition (CVPR), pages 3583–3592.
Drost, B., Ulrich, M., Navab, N., and Ilic, S. (2010). Model
globally, match locally: Efficient and robust 3d object
recognition. In International Conference on Computer
Vision and Pattern Recognition (CVPR), pages 998–
1005.
Ebden, M. (2008). Gaussian processes for regression: A
quick introduction. the website of robotics research
group in department on engineering science. Univer-
sity of Oxford: Oxford.
Guo, Y., Bennamoun, M., Sohel, F., Lu, M., and Wan,
J. (2014). 3d object recognition in cluttered scenes
with local surface features: a survey. IEEE Transacti-
ons on Pattern Analysis and Machine Intelligence,
36(11):2270–2287.
Guo, Y., Bennamoun, M., Sohel, F., Lu, M., Wan, J., and
Kwok, N. M. (2016). A comprehensive performance
evaluation of 3d local feature descriptors. Internatio-
nal Journal of Computer Vision, 116(1):66–89.
Hagelskjaer, F., Buch, A. G., et al. (2017). A novel 2.5 d
feature descriptor compensating for depth rotation. In
International conference on Computer Vision Theory
and Applications (VISAPP).
Hagelskjær, F., Kr
¨
uger, N., and Buch, A. G. (2017). Does
vision work well enough for industry? International
conference on Computer Vision Theory and Applica-
tions (VISAPP).
Hinterstoisser, S., Lepetit, V., Ilic, S., Holzer, S., Bradski,
G., Konolige, K., and Navab, N. (2012). Model based
training, detection and pose estimation of texture-less
3d objects in heavily cluttered scenes. In Asian confe-
rence on computer vision (ACCV), pages 548–562.
Johnson, A. E. and Hebert, M. (1999). Using spin images
for efficient object recognition in cluttered 3d scenes.
IEEE Transactions on Pattern Analysis and Machine
Intelligence, 21(5):433–449.
Jones, D. R. (2001). A taxonomy of global optimization
methods based on response surfaces. Journal of global
optimization, 21(4):345–383.
Jørgensen, T. B., Iversen, T. M., Lindvig, A. P., and Kr
¨
uger,
N. (2018). Simulation-based optimization of camera
placement in the context of industrial pose estimation.
In International conference on Computer Vision The-
ory and Applications (VISAPP).
Kehl, W., Manhardt, F., Tombari, F., Ilic, S., and Navab, N.
(2017). Ssd-6d: Making rgb-based 3d detection and
6d pose estimation great again. In Proceedings of the
International Conference on Computer Vision (ICCV
2017), Venice, Italy, pages 22–29.
Li, M. and Hashimoto, K. (2018). Accurate object pose
estimation using depth only. Sensors, 18(4):1045.
Lowe, D. G. (1999). Object recognition from local scale-
invariant features. In International conference on
Computer Vision (ICCV), volume 2, pages 1150–
1157.
Oshima, M. and Shirai, Y. (1983). Object recognition using
three-dimensional information. IEEE Transactions on
Pattern Analysis and Machine Intelligence, (4):353–
361.
Qi, C. R., Su, H., Mo, K., and Guibas, L. J. (2017). Pointnet:
Deep learning on point sets for 3d classification and
segmentation. International Conference on Computer
Vision and Pattern Recognition (CVPR), 1(2):4.
Rad, M. and Lepetit, V. (2017). Bb8: A scalable, accu-
rate, robust to partial occlusion method for predicting
the 3d poses of challenging objects without using
depth. In International Conference on Computer Vi-
sion (ICCV).
Rasmussen, C. E. (2004). Gaussian processes in machine
learning. In Advanced lectures on machine learning,
pages 63–71. Springer.
Rios, L. M. and Sahinidis, N. V. (2013). Derivative-free op-
timization: a review of algorithms and comparison of
software implementations. Journal of Global Optimi-
zation, 56(3):1247–1293.
Rusu, R. B., Blodow, N., and Beetz, M. (2009). Fast point
feature histograms (fpfh) for 3d registration. In In-
ternational Conference on Robotics and Automation
(ICRA), pages 3212–3217.
Salti, S., Tombari, F., and Di Stefano, L. (2014). Shot: Uni-
que signatures of histograms for surface and texture
description. Computer Vision and Image Understan-
ding, 125:251–264.
Snoek, J., Larochelle, H., and Adams, R. P. (2012). Practi-
cal bayesian optimization of machine learning algo-
rithms. In Advances in Neural Information Processing
Systems (NIPS), pages 2951–2959.
Tejani, A., Tang, D., Kouskouridas, R., and Kim, T.-K.
(2014). Latent-class hough forests for 3d object de-
tection and pose estimation. In European Conference
on Computer Vision (ECCV), pages 462–477.
Tekin, B., Sinha, S. N., and Fua, P. (2018). Real time se-
amless single shot 6d object pose prediction. In Inter-
national Conference on Computer Vision and Pattern
Recognition (CVPR).
Tombari, F., Salti, S., and Di Stefano, L. (2010). Unique
shape context for 3d data description. In Proceedings
of the ACM workshop on 3D object retrieval, pages
57–62.
Zhang, Z. (2012). Microsoft kinect sensor and its effect.
IEEE multimedia, 19(2):4–10.
VISAPP 2019 - 14th International Conference on Computer Vision Theory and Applications
142