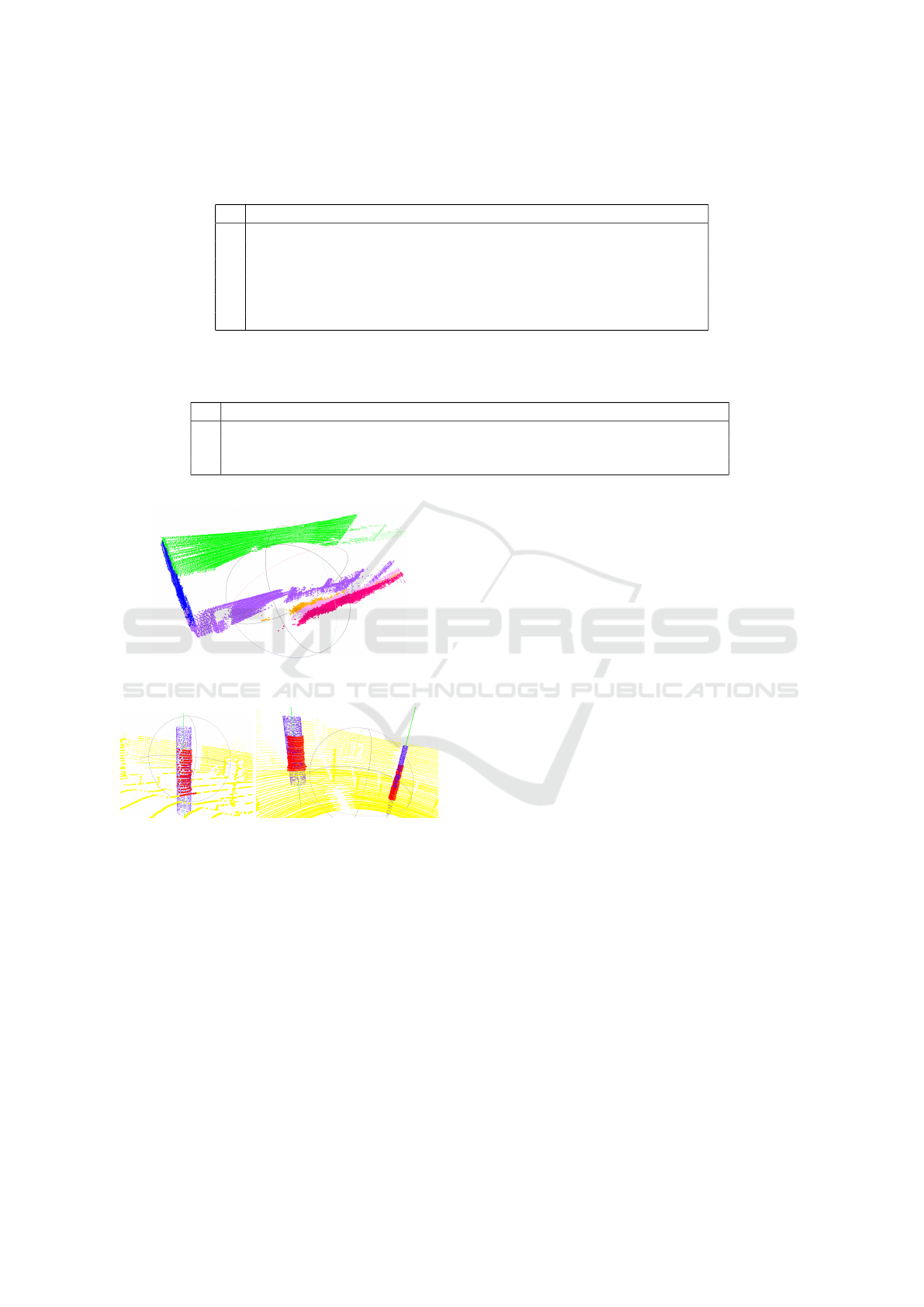
Table 3: Parameters of the detected planes in Figure 10. N denotes the number of points for which the plane fitting is carried
out. Vectors P
0
and n denote a point of the plane, and the plane normal. Five out of the six plane are quasi parallel, the blue
one is perpendicular to the others.
color N P
0
n
1. green 31 743 (1.881, 0.999, -0.191) (-0.098, -0.995, 0,013)
2. magenta 10 328 (-2.486, 0.998, -1.071) (-0.059, -0.959, 0.055)
3. purple 9007 (-1.394, 0.996, 0.7974) (-0.235, -0.961, -0.148)
4. rose 4076 (-2.203, 0.989, -1.2377) (0.021, -0.940, 0.340)
5. blue 5698 (0.459, -0.041, 5.0901) (0.893, -0.449, 0.013)
6. orange 2428 (-2.086, 0.976, -0.572) (-0.143, 0.867, 0.478)
Table 4: Parameters of the detected cylinders in Figure 11. N denotes the number of the points of a cylinder. Vectors C and
W denote a point of the axis and the direction of the axis. r and h are the radius and the height value of the cylinder, finally,
E denotes the fitting error given in Euclidean distance.
N C W r h E
1. 660 (5.560, 0.029, -0.432) (0.000,0.000, 1.000) 0.127 2.257 0.001
2. 782 (6.203, 7.609, -0.174) (-0.024,0.074, 0.996) 0.559 2.464 0.002
3. 263 (6.687, 16.955, 0.340) (0.000,0.078, 0.996) 0.487 2.352 0.006
Figure 10: Fitted planes of an outdoor test in case of high
noise because of mirroring.
Figure 11: Fitted cylinders of some outdoor measurements.
ACKNOWLEDGEMENTS
EFOP-3.6.3-VEKOP-16-2017-00001: Talent Man-
agement in Autonomous Vehicle Control Technolo-
gies – The Project is supported by the Hungarian
Government and co-financed by the European Social
Fund.
REFERENCES
Awrangjeb, M. and Fraser, C. S. (2014). Automatic seg-
mentation of raw LIDAR data for extraction of build-
ing roofs. Remote Sensing, 6(5):3716–3751.
Bj
¨
orck,
˚
A. (1996). Numerical Methods for Least Squares
Problems. Siam.
Eberly, D. Fitting 3D Data with a Cylinder. http://
www.geometrictools.com/Documentation/Cylinder
Fitting.pdf. Online; accessed at 5th November 2018.
Eberly, D. Least Squares Fitting of Segments by Line or
Plane. https://www.geometrictools.com/Documentati
on/FitSegmentsByLineOrPlane.pdf. Online; accessed
at 5th November 2018.
Eberly, D. Least Squares Fitting on Data. http://www.geo
metrictools.com/Documentation/LeastSquaresFitt
ing.pdf. Online; accessed at 5th November 2018.
Fischler, M. and Bolles, R. (1981). RANdom SAmpling
Consensus: a paradigm for model fitting with appli-
cation to image analysis and automated cartography.
Commun. Assoc. Comp. Mach., 24:358–367.
Grant, W. S., Voorhies, R., and Itti, L. (2013). Finding
planes in lidar point clouds for real-time registration.
In International Conference on Intelligent Robots and
Systems, pages 4347–4354.
Hartley, R. and Zisserman, A. (2003). Multiple view geom-
etry in computer vision. Cambridge University Press.
Nurunnabi, A. and andoderik Lindenbergh, Y. S. (2017).
Robust cylinder fitting in three-dimensional point
cloud dara. In The International Archives of the Pho-
togrammetry, Remote Sensing and Spatial Informa-
tion Sciences, pages 63–70.
Pereira, M., Silva, D., Santos, V. M. F., and Dias, P. (2016).
Self calibration of multiple lidars and cameras on au-
tonomous vehicles. Robotics and Autonomous Sys-
tems, 83:326–337.
Pratt, V. (1987). Direct Least-squares Fitting of Algebraic
Surfaces. In Proceedings of the 14th Annual Con-
ference on Computer Graphics and Interactive Tech-
niques, SIGGRAPH ’87, pages 145–152.
Tsai, A., Hsu, C., Hong, I.-C., and Liu, W.-K. (2010). Plane
and boundary extraction from lidar data using cluster-
ing and convex hull projection. In IAPRS, pages 175–
179.
VISAPP 2019 - 14th International Conference on Computer Vision Theory and Applications
628