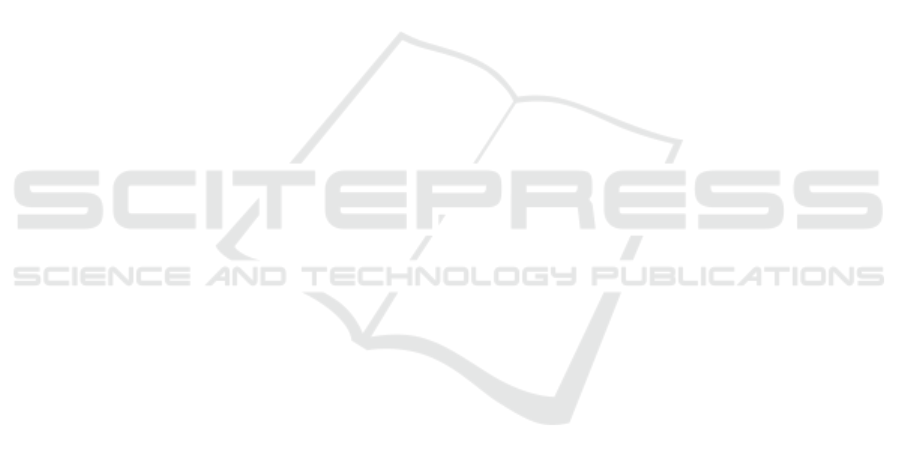
tive: accelerating cancer research by sharing data. The
oncologist, 20(5):464–e20.
Groth, P., Loizou, A., Gray, A. J., Goble, C., Harland, L.,
and Pettifer, S. (2014). Api-centric linked data inte-
gration: the open PHACTS discovery platform case
study. Web Semantics: Science, Services and Agents
on the World Wide Web, 29:12–18.
Jansen, C., Beier, M., Witt, M., Frey, S., and Krefting, D.
(2017). Towards reproducible research in a biomed-
ical collaboration platform following the FAIR guid-
ing principles. In Companion Proceedings of the10th
International Conference on Utility and Cloud Com-
puting, pages 3–8. ACM.
Lancaster, O., Beck, T., Atlan, D., Swertz, M., Thangavelu,
D., Veal, C., Dalgleish, R., and Brookes, A. J. (2015).
Cafe Variome: General-purpose software for making
genotype–phenotype data discoverable in restricted or
open access contexts. Human mutation, 36(10):957–
964.
Magazine, D.-L. (2011). The dataverse network
R
: an
open-source application for sharing, discovering and
preserving data. D-lib Magazine, 17(1/2).
Martin-Sanchez, F. and Verspoor, K. (2014). Big data in
medicine is driving big changes. Yearbook of medical
informatics, 9(1):14.
McQuilton, P., Gonzalez-Beltran, A., Rocca-Serra, P.,
Thurston, M., Lister, A., Maguire, E., and Sansone,
S.-A. (2016). Biosharing: curated and crowd-sourced
metadata standards, databases and data policies in the
life sciences. Database, 2016.
Mons, B., Neylon, C., Velterop, J., Dumontier, M.,
da Silva Santos, L. O. B., and Wilkinson, M. D.
(2017). Cloudy, increasingly FAIR; revisiting the
FAIR data guiding principles for the European
Open Science Cloud. Information Services & Use,
37(1):49–56.
Phan, J. H., Quo, C. F., Cheng, C., and Wang, M. D. (2012).
Multiscale integration of-omic, imaging, and clini-
cal data in biomedical informatics. IEEE reviews in
biomedical engineering, 5:74–87.
Press, G. (2016). Cleaning big data: Most time-consuming,
least enjoyable data science task, survey says. Forbes,
March, 23.
Prinz, F., Schlange, T., and Asadullah, K. (2011). Believe
it or not: how much can we rely on published data on
potential drug targets? Nature reviews Drug discov-
ery, 10(9):712.
Pundir, S., Martin, M. J., and O’Donovan, C. (2017).
Uniprot protein knowledgebase. Protein Bioinformat-
ics: From Protein Modifications and Networks to Pro-
teomics, pages 41–55.
Razick, S., Mo
ˇ
cnik, R., Thomas, L. F., Ryeng, E., Drabløs,
F., and Sætrom, P. (2014). The eGenVar data manage-
ment system-cataloguing and sharing sensitive data
and metadata for the life sciences. Database, 2014.
Silva, L. B., Trifan, A., and Oliveira, J. L. (2018). Montra:
An agile architecture for data publishing and discov-
ery. Computer methods and programs in biomedicine,
160:33–42.
Trifan, A. and Oliveira, J. L. (2018). A FAIR market-
place for biomedical data custodians and clinical re-
searchers. In 2018 IEEE 31st International Sympo-
sium on Computer-Based Medical Systems (CBMS),
pages 188–193. IEEE.
Vaccarino, A. L., Dharsee, M., Strother, S. C., Aldridge,
D., Arnott, S. R., Behan, B., Dafnas, C., Dong, F.,
Edgecombe, K., El-Badrawi, R., et al. (2018). Brain-
code: A secure neuroinformatics platform for man-
agement, federation, sharing and analysis of multi-
dimensional neuroscience data. Frontiers in neuroin-
formatics, 12:28.
Wen, C.-H., Ou, S.-M., Guo, X.-B., Liu, C.-F., Shen, Y.-
B., You, N., Cai, W.-H., Shen, W.-J., Wang, X.-Q.,
and Tan, H.-Z. (2017). B-CAN: a resource sharing
platform to improve the operation, visualization and
integrated analysis of TCGA breast cancer data. On-
cotarget, 8(65):108778.
Wilkinson, M. D., Dumontier, M., Aalbersberg, I. J., Apple-
ton, G., Axton, M., Baak, A., Blomberg, N., Boiten,
J.-W., da Silva Santos, L. B., Bourne, P. E., et al.
(2016). The FAIR Guiding Principles for scientific
data management and stewardship. Scientific data, 3.
Yamamoto, Y., Yamaguchi, A., and Splendiani, A. (2018).
YummyData: providing high-quality open life science
data. Database, 2018.
HEALTHINF 2019 - 12th International Conference on Health Informatics
166