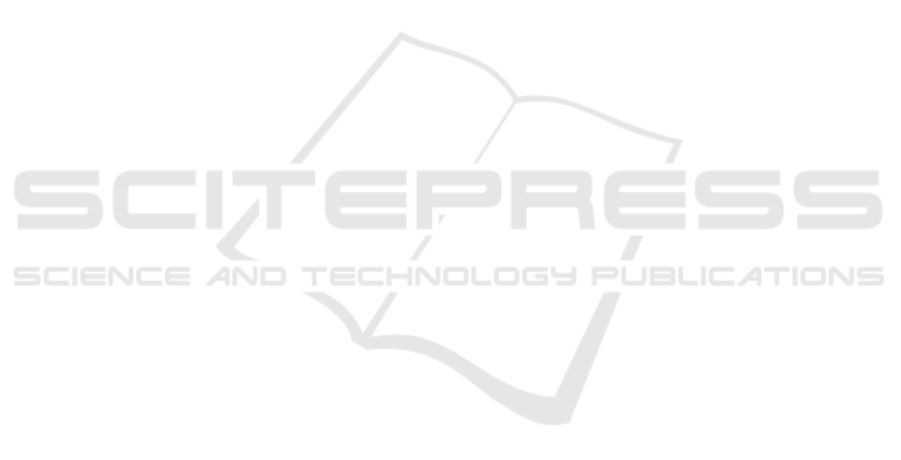
tives sequence) when primitives sequence is proned to
errors. In most cases, with less than 20% of average
detection/classification R-CNN errors, the mining al-
gorithm is able to find more than 70% of motifs. This
can be considered as efficient from a musicologist vie-
wpoint to target the major motifs but we believe that
these performances can still be improved.
In our future work, we will try to use the gap con-
straint of CSMA in order to see how we can reduce
the impact of errors on the extracted motifs.
ACKNOWLEDGEMENTS
The funding for this project was provided by a grant
from la R
´
egion Rh
ˆ
one Alpes. We acknowledge My-
lene Pardoen, the musicologist of the project for her
sound advices and expert recommendations.
REFERENCES
Benammar, R., Largeron, C., Eglin, V., and Pardoen, M.
(2017). Discovering motifs with variants in music
databases. In Intern. Symposium on Intelligent Data
Analysis, pages 14–26. Springer.
Calvo-Zaragoza, J. and Oncina, J. (2014). Recognition of
pen-based music notation: the homus dataset. In 22nd
Intern. Conf. on Pattern Recognition (ICPR), pages
3038–3043. IEEE.
Calvo-Zaragoza, J., Pertusa, A., and Oncina, J. (2017a).
Staff-line detection and removal using a convolutio-
nal neural network. Machine Vision and Applications,
pages 1–10.
Calvo-Zaragoza, J., Vigliensoni, G., and Fujinaga, I.
(2017b). Pixel-wise binarization of musical docu-
ments with convolutional neural networks. In Fif-
teenth IAPR Intern. Conf. on Machine Vision Appli-
cations (MVA), pages 362–365. IEEE.
Chen, L., Wang, S., Fan, W., Sun, J., and Satoshi, N. (2015).
Reconstruction combined training for convolutional
neural networks on character recognition. In Intern.
Conf. on Document Analysis and Recognition, pages
431–435. IEEE.
Forn
´
es, A., Dutta, A., Gordo, A., and Llad
´
os, J. (2012).
Cvc-muscima: a ground truth of handwritten music
score images for writer identification and staff remo-
val. Intern. Conf. on Document Analysis and Recog-
nition, pages 1–9.
Haji
ˇ
c, jr., J. and Pecina, P. (2017). In Search of a Dataset for
Handwritten Optical Music Recognition: Introducing
MUSCIMA++. CoRR.
Lee, K. C., Phon-Amnuaisuk, S., and Ting, C. Y. (2010).
Handwritten music notation recognition using hmma
non-gestural approach. In Intern. Conf.on Information
Retrieval & Knowledge Management,(CAMP), 2010,
pages 255–259. IEEE.
Lee, S., Son, S. J., Oh, J., and Kwak, N. (2016). Handwrit-
ten music symbol classification using deep convoluti-
onal neural networks. In Intern. Conf. on Information
Science and Security (ICISS), pages 1–5. IEEE.
Louloudis, G., Gatos, B., Stamatopoulos, N., and Papand-
reou, A. (2013). Icdar 2013 competition on writer
identification. In Intern. Conf. on Document Analy-
sis and Recognition, pages 1397–1401. IEEE.
Mitobe, Y., Miyao, H., and Maruyama, M. (2004). A fast
hmm algorithm based on stroke lengths for on-line re-
cognition of handwritten music scores. In Ninth In-
tern. Workshop on Frontiers in Handwriting Recogni-
tion, 2004. IWFHR-9, pages 521–526. IEEE.
Navarro, G. (2001). A guided tour to approximate
string matching. ACM computing surveys (CSUR),
33(1):31–88.
Pacha, A., Choi, K.-Y., Co
¨
uasnon, B., Ricquebourg, Y., Za-
nibbi, R., and Eidenberger, H. (2018a). Handwritten
music object detection: Open issues and baseline re-
sults. In Inter. Workshop on Document Analysis Sys-
tems.
Pacha, A., Haji
ˇ
c, J., and Calvo-Zaragoza, J. (2018b). A
baseline for general music object detection with deep
learning. Applied Sciences.
Pardoen, M. (2012). Projet Le Magasin de Musique
BIS. http://ladehis.ehess.fr/index.php?592. Accessed:
2018-11-14.
Rebelo, A., Capela, G., and Cardoso, J. S. (2010). Opti-
cal recognition of music symbols. Inter. journal on
document analysis and recognition, 13(1):19–31.
Ronneberger, O., Fischer, P., and Brox, T. (2015). U-net:
Convolutional networks for biomedical image seg-
mentation. In MICCAI, pages 234–241. Springer.
Szegedy, C., Ioffe, S., Vanhoucke, V., and Alemi, A. A.
(2017). Inception-v4, inception-resnet and the im-
pact of residual connections on learning. In AAAI,
volume 4, page 12.
Tard
´
on, L. J., Sammartino, S., Barbancho, I., G
´
omez,
V., and Oliver, A. (2009). Optical music recogni-
tion for scores written in white mensural notation.
EURASIP Journal on Image and Video Processing,
2009(1):843401.
Visani, M., Kieu, V. C., Forn
´
es, A., and Journet, N. (2013).
Icdar 2013 music scores competition: Staff removal.
In Intern. Conf. on Document Analysis and Recogni-
tion, pages 1407–1411. IEEE.
Wen, C., Rebelo, A., Zhang, J., and Cardoso, J. (2015). A
new optical music recognition system based on com-
bined neural network. Pattern Recognition Letters,
58:1–7.
Extraction of Musical Motifs from Handwritten Music Score Images
435