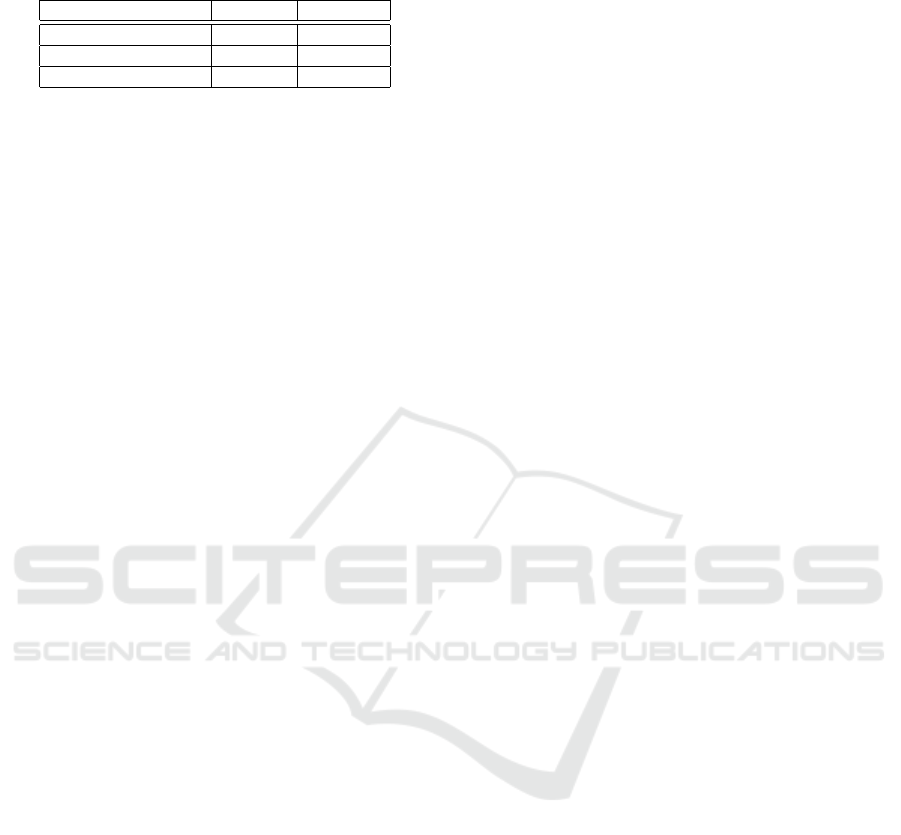
Table 6: Processing time of the decision stage for one 128×
128 testing image of the Outex-TC-00013 dataset.
Descriptor RSCCM EOCLBP
Feature computation 933 ms 3 000 ms
Classification 3 ms 3 ms
Total 936 ms 3 003 ms
6 CONCLUSION
In this paper, we have proposed a compact color tex-
ture representation based on the combination of tex-
ture features extracted from various configurations of
descriptors in multiple color spaces. This representa-
tion takes into account different color and spatial pro-
perties of the textures to be analyzed and overcomes
the difficulty of a prior parameter settings. In addi-
tion, a novel family of features computed from histo-
grams of LBP has been proposed in this paper.
Compared to others approaches, experiments car-
ried out on three benchmark texture databases give
competitive results that are very promising for future
work. The proposed approach should be improved by
using a filter model for feature selection rather than
the wrapper model chosen in this paper. Since filter
model is classifier-independent, it should greatly re-
duce the execution time of the learning stage. For the
decision stage, it would be interesting to apply more
performing classifiers like SVM.
Finally, in order to increase the classification accu-
racies, we plan to extend our approach to the com-
bination of texture features extracted from manifold
descriptors (RSCCM, EOCLBP and others) with dif-
ferent configurations and several color spaces.
REFERENCES
Alvarez, S. and Vanrell, M. (2012). Texton theory revisited:
A bag-of-words approach to combine textons. Pattern
Recognit., 45(12):4312–4325.
Bello-Cerezo, R., Bianconi, F., Fern
´
andez, A., Gonz
´
alez,
E., and Mar
´
ıa, F. D. (2016). Experimental comparison
of color spaces for material classification. J. Electron.
Imag., 25(6):061406.
Bianconi, F., Harvey, R., Southam, P., and Fernandez, A.
(2011). Theoretical and experimental comparison of
different approaches for color texture classification. J.
Electron. Imag., 20(4):043006.
Cernadas, E., Fern
´
andez-Delgado, M., Gonz
´
alez-Rufino,
E., and Carri
´
on, P. (2017). Influence of normalization
and color space to color texture classification. Pattern
Recognit., 61:120–138.
Cusano, C., Napoletano, P., and Schettini, R. (2016). Com-
bining multiple features for color texture classifica-
tion. J. Electron. Imag., 25(6):061410.
Guo, J.-M., Prasetyo, H., Lee, H., and Yao, C.-C. (2016).
Image retrieval using indexed histogram of void-and-
cluster block truncation coding. Signal Processing,
123:143–156.
Kalakech, M., Porebski, A., Vandenbroucke, N., and Ha-
mad, D. (2018). Unsupervised local binary pattern
histogram selection scores for color texture classifica-
tion. J. Imaging, 4(10):1–17.
Khan, F. S., Anwer, R. M., van de Weijer, J., Felsberg, M.,
and Laaksonen, J. (2015). Compact color-texture des-
cription for texture classification. Pattern Recognit.
Lett., 51:16–22.
Ledoux, A., Losson, O., and Macaire, L. (2016). Color
local binary patterns: compact descriptors for texture
classification. J. Electron. Imag., 25(6):061404.
Liu, L., Fieguth, J. C. P., Zhao, G., Chellappa, R., and Pie-
tik
¨
ainen, M. (2018). From BoW to CNN: Two decades
of texture representation for texture classification. Int.
J. Comput. Vis., pages 1–36.
Liu, P., Guo, J., Chamnongthai, K., and Prasetyo, H. (2017).
Fusion of color histogram and LBP-based features for
texture image retrieval and classification. Inf. Sci.,
390:95–111.
M
¨
aenp
¨
a
¨
a, T. and Pietik
¨
ainen, M. (2004). Classification with
color and texture: jointly or separately? Pattern Re-
cognit., 37(8):1629–1640.
Palm, C. (2004). Color texture classification by inte-
grative co-occurrence matrices. Pattern Recognit.,
37(5):965–976.
Pietik
¨
ainen, M., Zhao, G., Hadid, A., and Ahonen, T.
(2011). Computer Vision Using Local Binary Pat-
terns. Number 40 in Computational Imaging and Vi-
sion. Springer.
Porebski, A., Hoang, V. T., Vandenbroucke, N., and Hamad,
D. (2018). Multi-color space local binary pattern-
based feature selection for texture classification. J.
Electron. Imag., 27(1):011010.
Porebski, A., Vandenbroucke, N., and Hamad, D. (2013a).
LBP histogram selection for supervised color texture
classification. In IEEE Int. Conf. on Image Proces-
sing, pages 3239–3243, Melbourne, Australia.
Porebski, A., Vandenbroucke, N., and Macaire, L. (2013b).
Supervised texture classification: color space or tex-
ture feature selection? Pattern Anal. Applic., 16(1):1–
18.
Porebski, A., Vandenbroucke, N., Macaire, L., and Hamad,
D. (2014). A new benchmark image test suite for
evaluating color texture classification schemes. Mul-
timed. Tools Appl., 70(1):543–556.
Qazi, I., Alata, O., Burie, J., Moussa, A., and Fernandez-
Maloigne, C. (2011). Choice of a pertinent color
space for color texture characterization using parame-
tric spectral analysis. Pattern Recognit., 44(1):16–31.
Compact Color Texture Representation by Feature Selection in Multiple Color Spaces
443