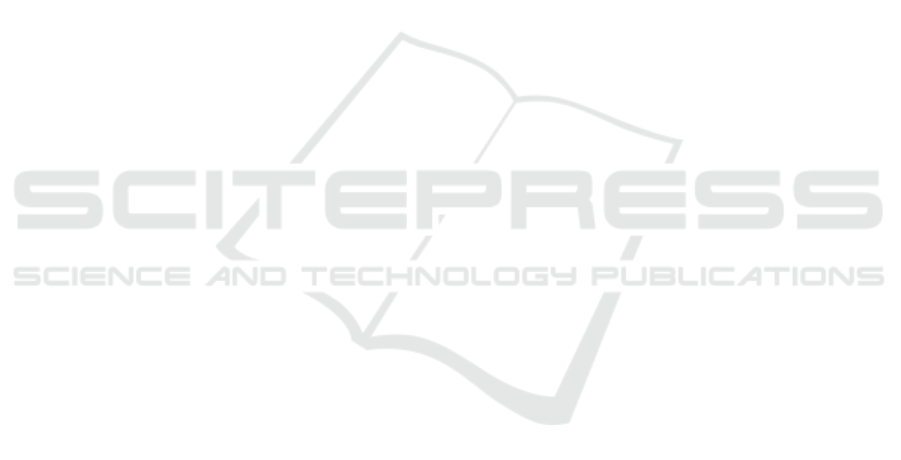
level adaboost-guided active shape model. Medical
Physics, 43(5).
Ino, F., Ooyama, K., and Hagihara, K. (2005). A data dis-
tributed parallel algorithm for nonrigid image regis-
tration. Parallel Computing, 31:19–43.
Ito, M. and Ino, F. (2018). An automated method for
generating training sets for deep learning based im-
age registration. In Proceedings of the 11th Inter-
national Joint Conference on Biomedical Engineering
Systems and Technologies (BIOSTEC 2018) - Volume
2: BIOIMAGING, Funchal, Madeira, Portugal, Jan-
uary 19-21, 2018., pages 140–147.
Ji
ˇ
r
´
ık, M., Ryba, T., Svobodov
´
a, M., M
´
ırka, H., and
Li
ˇ
ska, V. (2014). LISA - liver surgery ana-
lyzer software development. In Proceedings of the
11th World Congress on Computational Mechanics
(WCCM 2014), Barcelona.
Keustermans, J., Vandermeulen, D., and Suetens, P. (2012).
Integrating statistical shape models into a graph cut
framework for tooth segmentation. In Wang, F., Shen,
D., Yan, P., and Suzuki, K., editors, Machine Learn-
ing in Medical Imaging, pages 242–249, Berlin, Hei-
delberg. Springer Berlin Heidelberg.
Kodym, O. and
ˇ
Span
ˇ
el, M. (2018). Semi-automatic CT im-
age segmentation using random forests learned from
partial annotations. In Proceedings of the 11th In-
ternational Joint Conference on Biomedical Engineer-
ing Systems and Technologies - Volume 2: BIOIMAG-
ING,, pages 124–131. INSTICC, SciTePress.
Kr
ˇ
cah, M., Sz
´
ekely, G., and Blanc, R. (2011). Fully auto-
matic and fast segmentation of the femur bone from
3D-CT images with no shape prior. In 2011 IEEE In-
ternational Symposium on Biomedical Imaging: From
Nano to Macro, pages 2087–2090.
Liu, F., Zhou, Z., Jang, H., Samsonov, A., Zhao, G., and
Kijowski, R. (2018). Deep convolutional neural net-
work and 3d deformable approach for tissue segmen-
tation in musculoskeletal magnetic resonance imag-
ing. Magnetic resonance in medicine, 79(4):2379–
2391.
Loh, W.-Y. (2011). Classification and regression trees.
Wiley Interdisciplinary Reviews: Data Mining and
Knowledge Discovery, 1(1):14–23.
Milletari, F., Navab, N., and Ahmadi, S.-A. (2016). V-
net: Fully convolutional neural networks for volumet-
ric medical image segmentation. 2016 Fourth Inter-
national Conference on 3D Vision (3DV), pages 565–
571.
Ojalammi, A. and Malinen, J. (2017). Automated segmen-
tation of upper airways from MRI: Vocal tract geom-
etry extraction. In Proceedings of the 10th Interna-
tional Joint Conference on Biomedical Engineering
Systems and Technologies, volume 2, pages 77–84.
SciTePress.
Pinheiro, M. and Alves, J. L. (2015). A new level-set-
based protocol for accurate bone segmentation from
CT imaging. IEEE Access, 3:1894–1906.
Prasoon, A., Petersen, K., Igel, C., Lauze, F., Dam, E., and
Nielsen, M. (2013). Deep feature learning for knee
cartilage segmentation using a triplanar convolutional
neural network. In International conference on medi-
cal image computing and computer-assisted interven-
tion, pages 246–253. Springer.
Rathnayaka, K., Sahama, T., Schuetz, M. A., and Schmutz,
B. (2011). Effects of CT image segmentation meth-
ods on the accuracy of long bone 3D reconstructions.
Medical engineering & physics, 33(2):226–233.
Rohlfing, T. and Maurer, C. R. (2003). Nonrigid im-
age registration in shared-memory multiprocessor en-
vironments with application to brains, breasts, and
bees. IEEE Transactions on Information Technology
in Biomedicine, 7(1):16–25.
Ronneberger, O., Fischer, P., and Brox, T. (2015). U-net:
Convolutional networks for biomedical image seg-
mentation. In International Conference on Medical
image computing and computer-assisted intervention,
pages 234–241. Springer.
Tetsworth, K. D., Block, S., and Glatt, V. (2017). Putting
3D modelling and 3D printing into practice: virtual
surgery and preoperative planning to reconstruct com-
plex post-traumatic skeletal deformities and defects.
In SICOT-J.
Tomasi, C. and Manduchi, R. (1998). Bilateral filtering for
gray and color images. In Computer Vision, 1998.
Sixth International Conference on, pages 839–846.
IEEE.
Virz
`
ı, A., Marret, J. ., Muller, C. O., Berteloot, L., Boddaert,
N., Sarnacki, S., and Bloch, I. (2017). A new method
based on template registration and deformable models
for pelvic bones semi-automatic segmentation in pe-
diatric MRI. In 2017 IEEE 14th International Sympo-
sium on Biomedical Imaging (ISBI 2017), pages 323–
326.
Xi, T., Schreurs, R., Heerink, W. J., Berg
´
e, S. J.,
and Maal, T. J. J. (2014). A novel region-
growing based semi-automatic segmentation protocol
for three-dimensional condylar reconstruction using
cone beam computed tomography (CBCT). In PloS
one.
Xue, Y., Xu, T., Zhang, H., Long, L. R., and Huang, X.
(2018). Segan: Adversarial network with multi-scale
l 1 loss for medical image segmentation. Neuroinfor-
matics, pages 1–10.
Yokota, F., Okada, T., Takao, M., Sugano, N., Tada, Y.,
Tomiyama, N., and Sato, Y. (2013). Automated CT
segmentation of diseased hip using hierarchical and
conditional statistical shape models. In Mori, K.,
Sakuma, I., Sato, Y., Barillot, C., and Navab, N.,
editors, Medical Image Computing and Computer-
Assisted Intervention – MICCAI 2013, pages 190–
197, Berlin, Heidelberg. Springer Berlin Heidelberg.
BIOIMAGING 2019 - 6th International Conference on Bioimaging
160