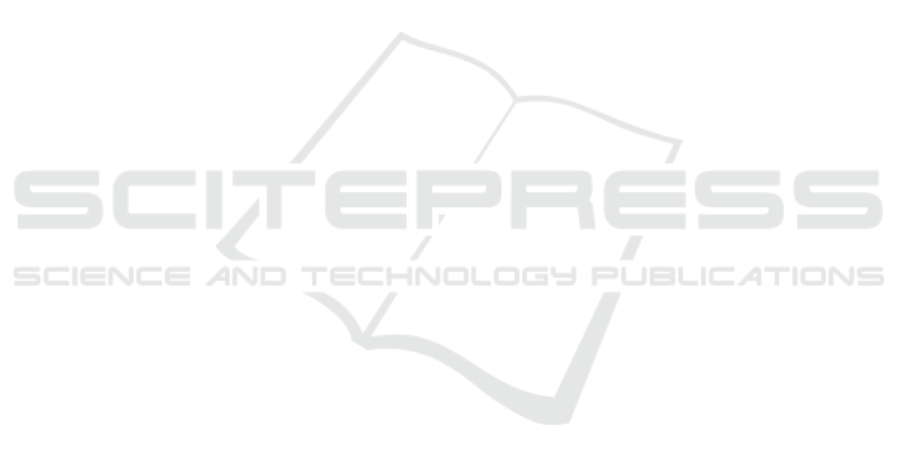
sentence-level location and context in the linked
literature where the relation originates from. These
detailed results are important for the bioinformatics
researchers who want to grasp an overall
comprehension of their interested entities and
relations.
5 CONCLUSIONS
In this article, focusing on the problem that current
“gene-mutation-disease” semantic types lack fine-
grained classification and corresponding relation
signal words, we propose a text-mining-assisted
semantic type construction approach for automatic
relation extraction from biomedical literature. We
eventually construct a semantic type with 5 layers and
16 categories as well as a corresponding signal word
vocabulary list with 58 commonly-used relation
words. Through coverage and guiding performance
test, even using the old-fashioned dictionary-based
methods, our semantic type is proved not only to have
good performance on coverage evaluation, but also
have great potential in assisting knowledge detection
and discovery from literature. In future works, we
will continue to study deep learning-based solutions
to extract “gene-mutation-disease” relations.
ACKNOWLEDGEMENTS
This Research was funded by National Key R&D
Program of China (2016YFC0901900).
Thanks to Doctor Jiao Li and her team at
Chinese Academy of Medical Sciences for the
guidance in biomedical ontology construction.
REFERENCES
Aljamel, A., Osman, T. and Acampora, G., 2015,
November. Domain-specific relation extraction: Using
distant supervision machine learning. In Knowledge
Discovery, Knowledge Engineering and Knowledge
Management (IC3K), 2015 7th International Joint
Conference on (Vol. 1, pp. 92-103). IEEE.
Allahyari, M., Pouriyeh, S., Assefi, M., Safaei, S., Trippe,
E.D., Gutierrez, J.B. and Kochut, K., 2017. A brief
survey of text mining: Classification, clustering and
extraction techniques. arXiv preprint
arXiv:1707.02919.
Bautista-Zambrana, M.R., 2015. Methodologies to build
ontologies for terminological purposes. Procedia-
Social and Behavioral Sciences, 173, pp.264-269.
Beheshti, M.S.H. and Ejei, F., 2015. Designing and
Implementing Basic Sciences Ontology Based on
Concepts and Relationships of Relevant Thesauri.
Iranian journal of Information Processing &
Management, 30(3), pp.677-696.
Burger, J.D., Doughty, E., Khare, R., Wei, C.H., Mishra,
R., Aberdeen, J., Tresner-Kirsch, D., Wellner, B.,
Kann, M.G., Lu, Z. and Hirschman, L., 2014. Hybrid
curation of gene-mutation relations combining
automated extraction and crowdsourcing. Database,
2014.
Christensen, J., Soderland, S. and Etzioni, O., 2011, June.
An analysis of open information extraction based on
semantic role labeling. In Proceedings of the sixth
international conference on Knowledge capture (pp.
113-120). ACM.
Degbelo, A., 2017, September. A Snapshot of Ontology
Evaluation Criteria and Strategies. In Proceedings of
the 13th International Conference on Semantic Systems
(pp. 1-8). ACM.
Dingerdissen, H.M., Torcivia-Rodriguez, J., Hu, Y., Chang,
T.C., Mazumder, R. and Kahsay, R., 2017. BioMuta
and BioXpress: mutation and expression
knowledgebases for cancer biomarker discovery.
Nucleic acids research, 46(D1), pp.D1128-D1136.
Fernández-López, M., Gómez-Pérez, A. and Suárez-
Figueroa, M.C., 2013. Methodological guidelines for
reusing general ontologies. Data & Knowledge
Engineering, 86, pp.242-275.
Landrum, M.J., Lee, J.M., Riley, G.R., Jang, W.,
Rubinstein, W.S., Church, D.M. and Maglott, D.R.,
2013. ClinVar: public archive of relationships among
sequence variation and human phenotype. Nucleic
acids research, 42(D1), pp.D980-D985.
Loper, E. and Bird, S., 2002, July. NLTK: The natural
language toolkit. In Proceedings of the ACL-02
Workshop on Effective tools and methodologies for
teaching natural language processing and
computational linguistics-Volume 1 (pp. 63-70).
Association for Computational Linguistics.
McCray, A.T., 1989, November. The UMLS Semantic
Network. In Proceedings. Symposium on Computer
Applications in Medical Care (pp. 503-507). American
Medical Informatics Association.
Miller, G.A. and Fellbaum, C., 2007. WordNet then and
now. Language Resources and Evaluation, 41(2),
pp.209-214.
Pal, H., 2016. Demonyms and compound relational nouns
in nominal open ie. In Proceedings of the 5th Workshop
on Automated Knowledge Base Construction (pp. 35-
39).
Piñero, J., Bravo, À., Queralt-Rosinach, N., Gutiérrez-
Sacristán, A., Deu-Pons, J., Centeno, E., Garcia-Garcia,
J., Sanz, F. and Furlong, L.I., 2016. DisGeNET: a
comprehensive platform integrating information on
human disease-associated genes and variants. Nucleic
acids research, p.gkw943.
Rather, N.N., Patel, C.O. and Khan, S.A., 2017. Using deep
learning towards biomedical knowledge discovery. Int.
J. Math. Sci. Comput.(IJMSC), 3(2), pp.1-10.
Construct Semantic Type of “Gene-mutation-disease” Relation by Computer-aided Curation from Biomedical Literature
129