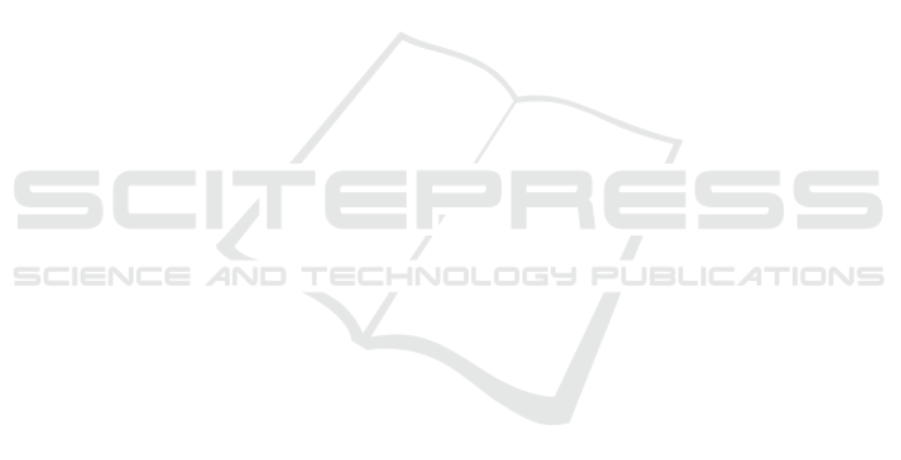
Choudhary, K., Deng, F., and Aviran, S. (2017). Compar-
ative and integrative analysis of RNA structural pro-
filing data: current practices and emerging questions.
Quantitative Biology, 5(1):3–24.
Eggenhofer, F., Tafer, H., Stadler, P. F., and Hofacker, I. L.
(2011). RNApredator: fast accessibility-based pre-
diction of sRNA targets. Nucleic Acids Res, 39(Web
Server issue):W149–54.
Fukunaga, T. and Hamada, M. (2017). RIblast: an ul-
trafast RNARNA interaction prediction system based
on a seed-and-extension approach. Bioinformatics,
33(17):2666–2674.
Hoe, C.-H., Raabe, C. A., Rozhdestvensky, T. S., and
Tang, T.-H. (2013). Bacterial sRNAs: Regulation in
stress. International Journal of Medical Microbiol-
ogy, 303(5):217 – 229.
Kolb, F. A., Malmgren, C., Westhof, E., Ehresmann, C.,
Ehresmann, B., Wagner, E. G., and Romby, P. (2000).
An unusual structure formed by antisense-target RNA
binding involves an extended kissing complex with
a four-way junction and a side-by-side helical align-
ment. RNA, 6(3):311–324.
K
¨
unne, T., Swarts, D. C., and Brouns, S. J. (2014). Plant-
ing the seed: target recognition of short guide RNAs.
Trends in Microbiology, 22(2):74 – 83.
Lalaouna, D., Simoneau-Roy, M., Lafontaine, D., and
Mass, E. (2013). Regulatory RNAs and target mRNA
decay in prokaryotes. Biochimica et Biophysica Acta
(BBA) - Gene Regulatory Mechanisms, 1829(6):742 –
747. RNA Decay Mechanisms.
Li, W., Ying, X., Lu, Q., and Chen, L. (2012). Predicting
sRNAs and their targets in bacteria. Genomics, Pro-
teomics & Bioinformatics, 10(5):276 – 284.
Lorenz, R., Bernhart, S. H., H
¨
oner zu Siederdissen, C.,
Tafer, H., Flamm, C., Stadler, P. F., and Hofacker,
I. L. (2011). ViennaRNA Package 2.0. Algorithms
for Molecular Biology, 6(1):26.
Lott, S. C., Sch
¨
afer, R. A., Mann, M., Backofen, R., Hess,
W. R., Voss, B., and Georg, J. (2018). GLASSgo -
automated and reliable detection of sRNA homologs
from a single input sequences. Frontiers in Genetics,
9:124.
Mann, M., Wright, P. R., and Backofen, R. (2017). In-
taRNA 2.0: enhanced and customizable prediction
of RNA-RNA interactions. Nucleic Acids Res.,
45(W1):W435–W439.
Miladi, M., Montaseri, S., Backofen, R., and Raden, M.
(2019). Integration of accessibility data from structure
probing into RNA-RNA interaction prediction. Bioin-
formatics, (epub ahead of print).
M
¨
uckstein, U., Tafer, H., Hackerm
¨
uller, J., Bernhart, S. H.,
Stadler, P. F., and Hofacker, I. L. (2006). Thermo-
dynamics of RNA–RNA binding. Bioinformatics,
22(10):1177–1182.
Nitzan, M., Rehani, R., and Margalit, H. (2017). Integra-
tion of bacterial small RNAs in regulatory networks.
Annual Review of Biophysics, 46(1):131–148.
Raden, M., Mohamed, M. M., Ali, S. M., and
Backofen, R. (2018). Interactive implementa-
tions of thermodynamics-based RNA structure and
RNA-RNA interaction prediction approaches for
example-driven teaching. PLOS Comput. Biol,
14(8):e1006341.
Richter, A. S. and Backofen, R. (2012). Accessibility
and conservation: General features of bacterial small
RNA-mRNA interactions? RNA Biol, 9(7):954–65.
Storz, G., Vogel, J., and Wassarman, K. (2011). Regula-
tion by small RNAs in bacteria: Expanding frontiers.
Molecular Cell, 43(6):880 – 891.
Tinoco Jr, I., Borer, P., Dengler, B., Levin, M., Uhlenbeck,
O., Crothers, D., and Bralla, J. (1973). Improved es-
timation of secondary structure in ribonucleic acids.
Nature New Biology, 246(150):40–41.
Tjaden, B., Goodwin, S. S., Opdyke, J. A., Guillier, M.,
Fu, D. X., Gottesman, S., and Storz, G. (2006). Tar-
get prediction for small, noncoding RNAs in bacteria.
Nucleic Acids Res., 34(9):2791–2802.
Turner, D. H. and Mathews, D. H. (2010). NNDB: the near-
est neighbor parameter database for predicting stabil-
ity of nucleic acid secondary structure. Nucleic Acids
Res, 38(Database issue):D280–2.
Umu, S. U. and Gardner, P. P. (2017). A comprehensive
benchmark of RNA-RNA interaction prediction tools
for all domains of life. Bioinformatics, 33(7):988–
996.
Wright, P. R., Georg, J., Mann, M., Sorescu, D. A., Richter,
A. S., Lott, S., Kleinkauf, R., Hess, W. R., and Back-
ofen, R. (2014). CopraRNA and IntaRNA: predicting
small RNA targets, networks and interaction domains.
Nucleic Acids Res., 42(Web Server issue):W119–23.
Wright, P. R., Mann, M., and Backofen, R. (2018). Struc-
ture and interaction prediction in prokaryotic RNA bi-
ology. Microbiol Spectrum, 6(2).
Wright, P. R., Richter, A. S., Papenfort, K., Mann, M.,
Vogel, J., Hess, W. R., Backofen, R., and Georg, J.
(2013). Comparative genomics boosts target predic-
tion for bacterial small RNAs. Proceedings of the Na-
tional Academy of Sciences, 110(37):E3487–96.
APPENDIX
A Benchmarking Workflow
The benchmark workflow used for the creation of the
prediction accuracy plots is shown in Figure 8. First,
for each sRNA query, optimal interactions with all
mRNA targets are predicted using INTARNA with a
certain parameter set. Then the resulting interactions
are sorted according to their energy values, from the
most favourable, i.e. those with the lowest values, to
the least favourable. Finally, we identify the rank of
each verified target (considered a supported predic-
tion). For each maximal rank value (later plotted on
the x-axis), we count the number of verified targets for
all sRNA queries that show a rank smaller or equal to
this.
This process is repeated for each benchmarked pa-
rameter set. The data collected is then plotted. The
BIOINFORMATICS 2019 - 10th International Conference on Bioinformatics Models, Methods and Algorithms
138