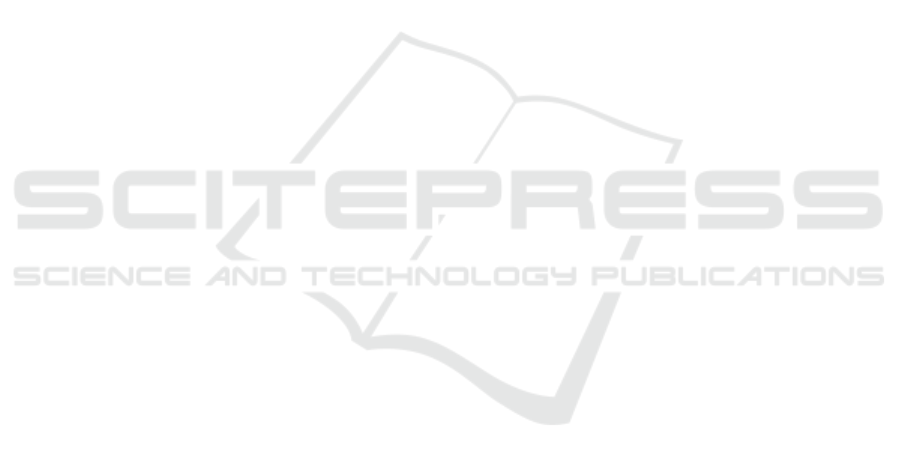
REFERENCES
Alarcon-Aguilar, F., Hernandez-Galicia, E., Campos-
Sepulveda, A., Xolalpa-Molina, S., Rivas-Vilchis, J.,
Vazquez-Carrillo, L., and Roman-Ramos, R. (2002).
Evaluation of the hypoglycemic effect of cucurbita fi-
cifolia bouch
´
e (cucurbitaceae) in different experimen-
tal models. Journal of Ethnopharmacology, 82(2-
3):185–189.
Berger, J. P., SinhaRoy, R., Pocai, A., Kelly, T. M., Scapin,
G., Gao, Y.-D., Pryor, K. A. D., Wu, J. K., Eiermann,
G. J., Xu, S. S., et al. (2018). A comparative study
of the binding properties, dipeptidyl peptidase-4 (dpp-
4) inhibitory activity and glucose-lowering efficacy of
the dpp-4 inhibitors alogliptin, linagliptin, saxagliptin,
sitagliptin and vildagliptin in mice. Endocrinology,
Diabetes & Metabolism, 1(1):e00002.
Biftu, T., Sinha-Roy, R., Chen, P., Qian, X., Feng, D.,
Kuethe, J. T., Scapin, G., Gao, Y. D., Yan, Y., Krueger,
D., et al. (2014). Omarigliptin (mk-3102): a novel
long-acting dpp-4 inhibitor for once-weekly treatment
of type 2 diabetes.
Davidson, J. A., Parente, E. B., and Gross, J. L. (2008). In-
cretin mimetics and dipeptidyl peptidase-4 inhibitors:
innovative treatment therapies for type 2 diabetes. Ar-
quivos Brasileiros de Endocrinologia & Metabologia,
52(6):1039–1049.
Ferreira, L., dos Santos, R., Oliva, G., and Andricopulo, A.
(2015). Molecular docking and structure-based drug
design strategies. Molecules, 20(7):13384–13421.
Hiramatsu, H., Kyono, K., Higashiyama, Y., Fukushima, C.,
Shima, H., Sugiyama, S., Inaka, K., Yamamoto, A.,
and Shimizu, R. (2003). The structure and function
of human dipeptidyl peptidase iv, possessing a unique
eight-bladed β-propeller fold. Biochemical and bio-
physical research communications, 302(4):849–854.
Johari, S., Sharmah, R., and Sinha, S. (2011). Ligand bind-
ing studies for dpp iv a target protein responsible for
diabetes mellitus type 2: Structural based approach for
drug designing. In Emerging Trends and Applications
in Computer Science (NCETACS), 2011 2nd National
Conference on, pages 1–4. IEEE.
Marbury, T., Huang, W.-C., Strange, P., and Lebovitz, H.
(1999). Repaglinide versus glyburide: a one-year
comparison trial1. Diabetes research and clinical
practice, 43(3):155–166.
Nachabe, L., ElHassan, B., AlMouhammad, D., and Genet,
M. G. (2017). Intelligent system for diabetes patients
monitoring and assistance. In Advances in Biomedical
Engineering (ICABME), 2017 Fourth International
Conference on, pages 1–4. IEEE.
Nauck, M. A., Kahle, M., Baranov, O., Deacon, C. F.,
and Holst, J. J. (2017). Addition of a dipeptidyl
peptidase-4 inhibitor, sitagliptin, to ongoing ther-
apy with the glucagon-like peptide-1 receptor ago-
nist liraglutide: A randomized controlled trial in pa-
tients with type 2 diabetes. Diabetes, Obesity and
Metabolism, 19(2):200–207.
PubChem (2005). ata deposited in or computed by pub-
chem. [Online; acesses November 27, 2018].
Richter, B., Bandeira-Echtler, E., Bergerhoff, K., and
Lerch, C. (2008). Dipeptidyl peptidase-4 (dpp-4)
inhibitors for type 2 diabetes mellitus. Cochrane
Database of Systematic Reviews, (2).
Rosenstock, J., Brazg, R., Andryuk, P. J., Lu, K., Stein,
P., Study, S., et al. (2006). Efficacy and safety of
the dipeptidyl peptidase-4 inhibitor sitagliptin added
to ongoing pioglitazone therapy in patients with
type 2 diabetes: a 24-week, multicenter, random-
ized, double-blind, placebo-controlled, parallel-group
study. Clinical therapeutics, 28(10):1556–1568.
Shityakov, S. and F
¨
orster, C. (2014). In silico predic-
tive model to determine vector-mediated transport
properties for the blood–brain barrier choline trans-
porter. Advances and applications in bioinformatics
and chemistry: AABC, 7:23.
Vignesh, N. A. and Amalarethinam, D. G. (2017). Rule
extraction for diagnosis of diabetes mellitus used for
enhancing regular covering technique. In Computing
and Communication Technologies (WCCCT), 2017
World Congress on, pages 111–114. IEEE.
BIOINFORMATICS 2019 - 10th International Conference on Bioinformatics Models, Methods and Algorithms
330