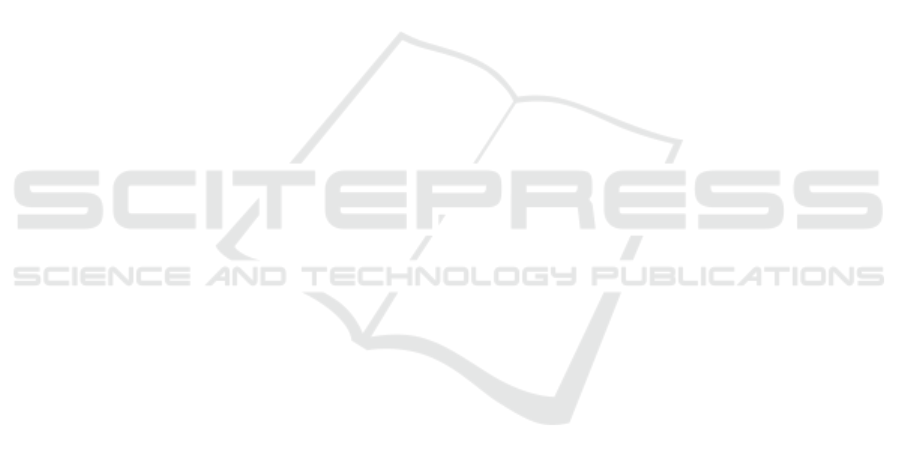
and trends in colorectal cancer incidence and morta-
lity. Gut, 66(4):683–691.
Axyonov, S., Liang, J., Kostin, K., Zamyatin, A. V., et al.
(2016). Advanced pattern recognition and deep lear-
ning for colon polyp detection.
Bernal, J., Histace, A., Masana, M., Angermann, Q.,
S
´
anchez-Montes, C., Rodriguez, C., Hammami, M.,
Garcia-Rodriguez, A., C
´
ordova, H., Romain, O., et al.
(2018). Polyp detection benchmark in colonoscopy vi-
deos using gtcreator: A novel fully configurable tool
for easy and fast annotation of image databases. In
Proceedings of 32nd CARS conference.
Bernal, J., Tajkbaksh, N., S
´
anchez, F. J., Matuszewski, B. J.,
Chen, H., Yu, L., Angermann, Q., Romain, O., Ru-
stad, B., Balasingham, I., et al. (2017). Comparative
validation of polyp detection methods in video colo-
noscopy: results from the miccai 2015 endoscopic vi-
sion challenge. IEEE transactions on medical ima-
ging, 36(6):1231–1249.
Corley, D. A., Jensen, C. D., Marks, A. R., Zhao, W. K.,
Lee, J. K., Doubeni, C. A., Zauber, A. G., de Boer,
J., Fireman, B. H., Schottinger, J. E., et al. (2014).
Adenoma detection rate and risk of colorectal can-
cer and death. New england journal of medicine,
370(14):1298–1306.
Felzenszwalb, P. F., Girshick, R. B., McAllester, D., and
Ramanan, D. (2010). Object detection with discri-
minatively trained part-based models. IEEE tran-
sactions on pattern analysis and machine intelligence,
32(9):1627–1645.
He, K., Zhang, X., Ren, S., and Sun, J. (2016). Deep resi-
dual learning for image recognition. In Proceedings of
the IEEE conference on computer vision and pattern
recognition, pages 770–778.
Hewett, D. G., Kaltenbach, T., Sano, Y., Tanaka, S., Saun-
ders, B. P., Ponchon, T., Soetikno, R., and Rex,
D. K. (2012). Validation of a simple classification sy-
stem for endoscopic diagnosis of small colorectal po-
lyps using narrow-band imaging. Gastroenterology,
143(3):599–607.
Hoang Ngan Le, T., Zheng, Y., Zhu, C., Luu, K., and Savvi-
des, M. (2016). Multiple scale faster-rcnn approach to
driver’s cell-phone usage and hands on steering wheel
detection. In Proceedings of the IEEE Conference on
Computer Vision and Pattern Recognition Workshops,
pages 46–53.
Ignjatovic, A., East, J. E., Suzuki, N., Vance, M., Guent-
her, T., and Saunders, B. P. (2009). Optical diagno-
sis of small colorectal polyps at routine colonoscopy
(detect inspect characterise resect and discard; discard
trial): a prospective cohort study. The lancet oncology,
10(12):1171–1178.
Jiang, H. and Learned-Miller, E. (2017). Face detection
with the faster r-cnn. In Automatic Face & Gesture
Recognition (FG 2017), 2017 12th IEEE International
Conference on, pages 650–657. IEEE.
Lieberman, D. A., Rex, D. K., Winawer, S. J., Giardiello,
F. M., Johnson, D. A., and Levin, T. R. (2012). Gui-
delines for colonoscopy surveillance after screening
and polypectomy: a consensus update by the us multi-
society task force on colorectal cancer. Gastroentero-
logy, 143(3):844–857.
Park, S. Y. and Sargent, D. (2016). Colonoscopic polyp
detection using convolutional neural networks. In
Medical Imaging 2016: Computer-Aided Diagnosis,
volume 9785, page 978528. International Society for
Optics and Photonics.
Ren, S., He, K., Girshick, R., and Sun, J. (2015). Faster
r-cnn: Towards real-time object detection with region
proposal networks. In Advances in neural information
processing systems, pages 91–99.
Ribeiro, E., Uhl, A., Wimmer, G., and H
¨
afner, M. (2016).
Exploring deep learning and transfer learning for co-
lonic polyp classification. Computational and mathe-
matical methods in medicine, 2016.
Wong, S. C., Gatt, A., Stamatescu, V., and McDonnell,
M. D. (2016). Understanding data augmentation
for classification: when to warp? arXiv preprint
arXiv:1609.08764.
Zhang, R., Zheng, Y., Mak, T. W. C., Yu, R., Wong, S. H.,
Lau, J. Y., and Poon, C. C. (2017). Automatic de-
tection and classification of colorectal polyps by trans-
ferring low-level cnn features from nonmedical dom-
ain. IEEE J. Biomedical and Health Informatics,
21(1):41–47.
Polyp Detection in Gastrointestinal Images using Faster Regional Convolutional Neural Network
631