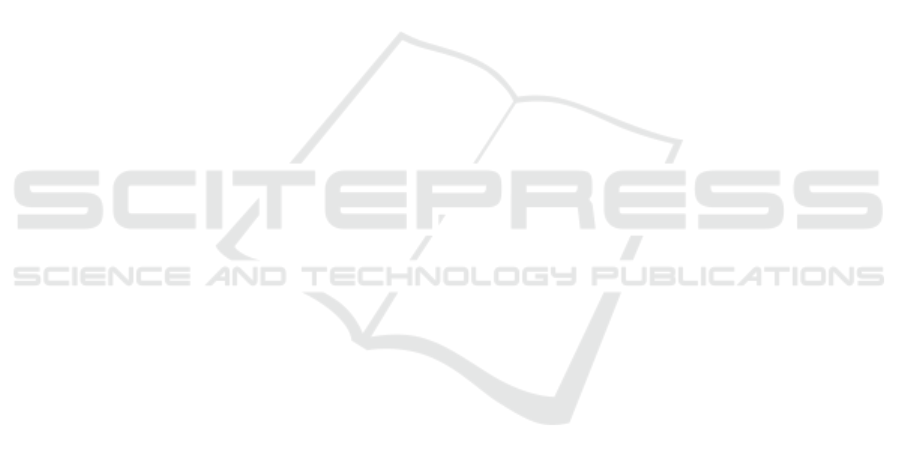
REFERENCES
Attias, H. (1999). Inferring parameters and structure of la-
tent variable models by variational bayes. In Proceed-
ings of the Fifteenth conference on Uncertainty in ar-
tificial intelligence, pages 21–30. Morgan Kaufmann
Publishers Inc.
Bishop, C. M. (2006). Pattern Recognition and Machine
Learning. Springer New York.
Chen, S. S. and Gopalakrishnan, P. S. (1998). Clustering via
the bayesian information criterion with applications in
speech recognition. In Acoustics, Speech and Signal
Processing, 1998. Proceedings of the 1998 IEEE In-
ternational Conference on, volume 2, pages 645–648.
IEEE.
Dempster, A. P., Laird, N. M., and Rubin, D. B. (1977).
Maximum likelihood from incomplete data via the
EM algorithm. Journal of the royal statistical soci-
ety. Series B (methodological), pages 1–38.
Diggle, P. J. (2013). Statistical analysis of spatial and
spatio-temporal point patterns. CRC Press.
Ester, M., Kriegel, H.-P., Sander, J., and Xu, X. (1996).
A density-based algorithm for discovering clusters in
large spatial databases with noise. pages 226–231.
AAAI Press.
EUMETSAT (2018). Metop design - IASI.
Greenspan, H., Goldberger, J., and Ridel, L. (2001). A con-
tinuous probabilistic framework for image matching.
Comput. Vis. Image Underst., 84(3):384–406.
Jin, H., Leung, K.-S., Wong, M.-L., and Xu, Z.-B. (2005a).
Scalable model-based cluster analysis using clustering
features. Pattern Recognition, 38(5):637–649.
Jin, H., Wong, M.-L., and Leung, K.-S. (2005b). Scal-
able model-based clustering for large databases based
on data summarization. IEEE Transactions on Pat-
tern Analysis and Machine Intelligence, 27(11):1710–
1719.
Kalnis, P., Mamoulis, N., and Bakiras, S. (2005). On
discovering moving clusters in spatio-temporal data.
In International Symposium on Spatial and Temporal
Databases, pages 364–381. Springer.
Kollios, G., Gunopulos, D., Koudas, N., and Berchtold,
S. (2003). Efficient biased sampling for approxi-
mate clustering and outlier detection in large data sets.
IEEE Transactions on Knowledge and Data Engineer-
ing, 15(5):1170–1187.
Kullback, S. (1997). Information theory and statistics.
Courier Corporation.
Li, Y., Han, J., and Yang, J. (2004). Clustering moving
objects. In Proceedings of the tenth ACM SIGKDD
international conference on Knowledge discovery and
data mining, pages 617–622. ACM.
Maciag, P. S. (2017). A survey on data mining methods for
clustering complex spatiotemporal data. In Interna-
tional Conference: Beyond Databases, Architectures
and Structures, pages 115–126. Springer.
Maimon, O. Z. H., editor (2010). Data mining and knowl-
edge discovery handbook. Springer, New York, 2. ed.
edition.
McLachlan, G. and Peel, D. (2004). Finite mixture models.
John Wiley & Sons.
McLachlan, G. J. and Basford, K. E. (1988). Mixture
models: Inference and applications to clustering, vol-
ume 84. Marcel Dekker.
Paci, L. and Finazzi, F. (2018). Dynamic model-based clus-
tering for spatio-temporal data. Statistics and Com-
puting, 28(2):359–374.
Rey, S. J. and Anselin, L. (2007). PySAL: A Python Li-
brary of Spatial Analytical Methods. The Review of
Regional Studies, 37(1):5–27.
Schneider, M., Borger, C., Wiegele, A., Hase, F., Garc
´
ıa,
O. E., Sep
´
ulveda, E., and Werner, M. (2017). Mu-
sica metop/iasi {H
2
O,δD} pair retrieval simulations
for validating tropospheric moisture pathways in at-
mospheric models. Atmospheric Measurement Tech-
niques, 10(2):507–525.
Trevor, H., Robert, T., and JH, F. (2009). The elements of
statistical learning: data mining, inference, and pre-
diction. Springer.
Zhang, T., Ramakrishnan, R., and Livny, M. (1996). Birch:
an efficient data clustering method for very large
databases. In ACM Sigmod Record, volume 25, pages
103–114. ACM.
KDIR 2019 - 11th International Conference on Knowledge Discovery and Information Retrieval
54