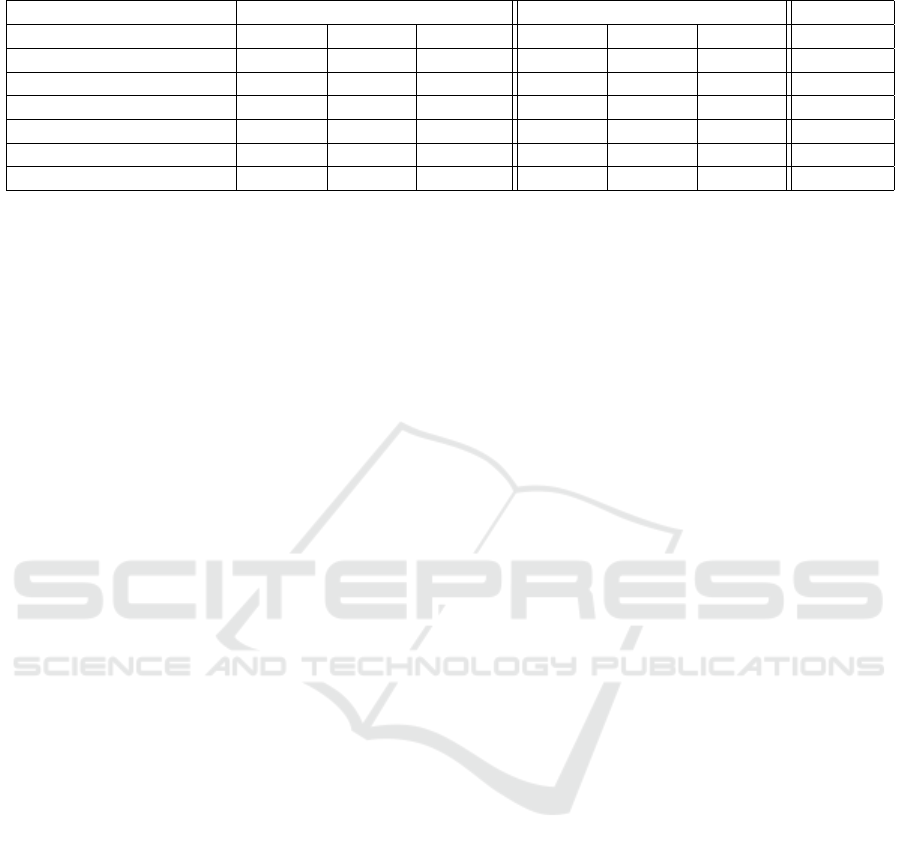
Table 4: Performance Comparison Results.
Using The Selected Feature Set Using The Full Feature Set Wilcoxon
Objective Average Min Max Average Min Max p-value
Mean flow-time 0.28238 0.18709 0.36727 0.31201 0.20618 0.41312 00.10%
Mean weighted flow-time 0.28278 0.19737 0.44225 0.29387 0.20499 0.43446 05.82%
Maximum flow-time 0.58983 0.47137 0.72148 0.67963 0.54417 0.83272 00.02%
Mean tardiness 0.28217 0.19048 0.38080 0.28607 0.20464 0.38240 35.70%
Mean weighted tardiness 0.30408 0.18893 0.47572 0.29339 0.20966 0.42190 16.97%
Maximum tardiness 0.55338 0.44895 0.70544 0.60615 0.41829 0.81957 00.19%
6 CONCLUSION AND FUTURE
WORK
This paper proposed an extension to Niching-GP Fea-
ture selection (NiSuFS) to be compatible with multi-
tree genetic programming for Dynamic Flexible Job
Shop Scheduling. NiSuFS was applied to DJSS in
(Mei et al., 2017) and the results proved its effective-
ness in improving rule generation. However, it was
not applied to DFJSS in other works. The results in
this paper showed that the extended NiSuFS can en-
hance the rule generation in multi-tree genetic pro-
gramming. The improvement was significant in 50%
of our tests and the generated rules were never sig-
nificantly worse than the baseline. For future work,
we plan to investigate the assumption that feature sig-
nificance can be measured on routing and sequenc-
ing separately despite the rule pair interaction. We
also plan to study information redundancy in features
and the applicability of feature reduction techniques
such as PCA. Since situation sampling for phenotype
measurement is completely random, different compo-
nents of the phenotype may be dependent, so pheno-
types with orthogonal components is worth exploring.
Moreover, other discriminative phenotype measure-
ment methods will be investigated in future work.
REFERENCES
Blackstone, J. H., Phillips, D. T., and Hogg, G. L. (1982).
A state-of-the-art survey of dispatching rules for man-
ufacturing job shop operations. In International Jour-
nal of Production Research, pages 27–45.
Brucker, P. and Schlie, R. (1990). Job-shop scheduling with
multi-purpose machines. Computing, 45:369–375.
Dauzere-Peres, S. and Paulli, J. (1997). An integrated ap-
proach for modeling and solving the general multipro-
cessor job-shop scheduling problem using tabu search.
In Ann. Oper. Res, pages 281–306.
Guyon, I. and Elisseeff, A. (2003). An introduction to vari-
able and feature selection. Journal of Machine Learn-
ing Research, 3:1157–1182.
Koza, J. (1992). Genetic programming: on the program-
ming of computers by means of natural selection. In
MIT press, volume volume 1.
Liu, H. and Yu, L. (2005). Toward integrating feature selec-
tion algorithms for classification and clustering. IEEE
Transactions on Knowledge and Data Engineering,
17(4):491–502.
Mei, Y., Nguyen, S., Xue, B., and Zhang, M. (2017).
An efficient feature selection algorithm for evolving
job shop scheduling rules with genetic programming.
IEEE Transactions on Emerging Topics in Computa-
tional Intelligence, 1(5):339–353.
Mei, Y., Zhang, M., and Nyugen, S. (2016). Feature selec-
tion in evolving job shop dispatching rules with ge-
netic programming. In GECCO.
Nguyen, S., Zhang, M., Johnston, M., and Tan, K. (2013).
A computational study of representations in genetic
programming to evolve dispatching rules for the job
shop scheduling problem. IEEE Transactions on Evo-
lutionary Computation, 17(5):621–639.
Yska, D., Mei, Y., and Zhang, M. (2018). Genetic pro-
gramming hyper-heuristic with cooperative coevolu-
tion for dynamic flexible job shop scheduling. In Pro-
ceedings of the European Conference on Genetic Pro-
gramming, pages 306–321. Springer.
Zhang, F., Mei, Y., and Zhang, M. (2018). Genetic pro-
gramming with multi-tree representation for dynamic
flexible job shop scheduling. In Australasian Joint
Conference on Artificial Intelligence, pages 472–484.
Springer.
ECTA 2019 - 11th International Conference on Evolutionary Computation Theory and Applications
146