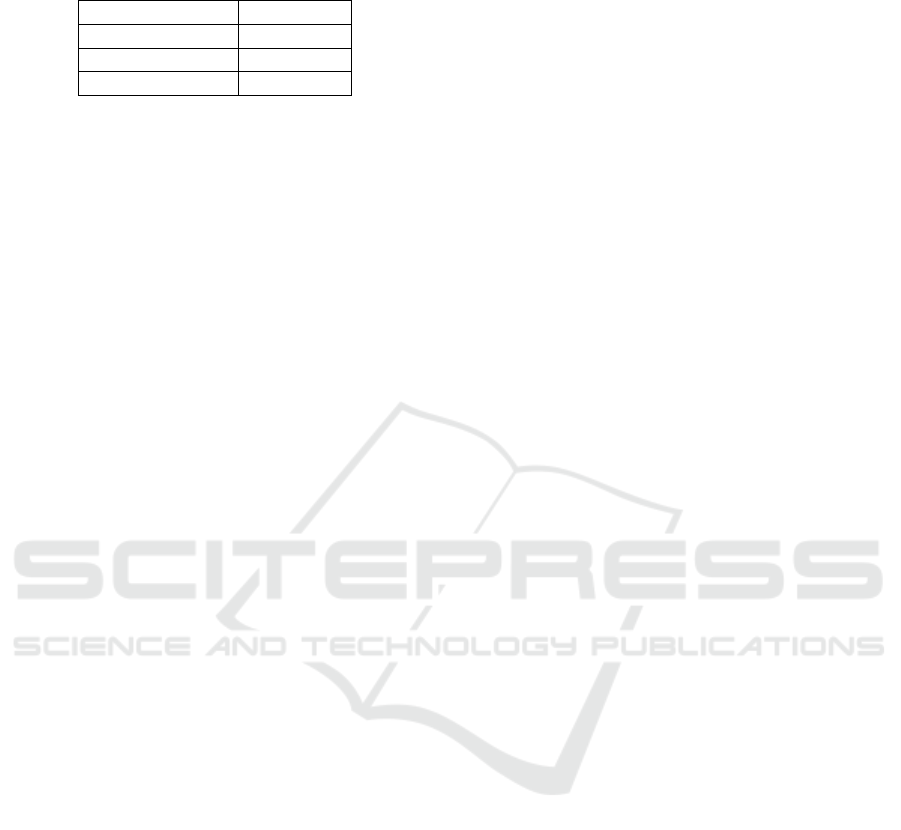
Table 6: Mean relative Uncertainty of Manufacturing costs
u
MC,rel
noted as µ ± 2σ.
mould standard u
MC,rel
/ %
E350 10.4 ± 3.9
P360 14.0 ± 1.5
V360 13.0 ± 2.3
for determining the number of mould nests could on
average be reduced on average by a factor of 4.
6 CONCLUSIONS
To recap, the initial CAD data is too complex and our
database too small to be processed directly to a learn-
ing approach. These kind of databases are quite com-
mon for production processes in Industry 4.0 scenar-
ios, especially in SMEs. With our work, we introduce
a framework on how to deal with such use cases. The
starting point is to reduce the CAD data to a lower di-
mensional feature space using expert knowledge. De-
pending on the number of features that are suggested
by the expert, we process using feature selection and
reduction. To reduce the complexity of the regres-
sion task even further, we proposed the use of a price
model with just some missing factors. We were able
to show that using a random forest model about 500
data records are sufficient to develop a price predic-
tion which meets the requirements. Results that do
not meet the requirements are easy to spot as outliers.
These still require the expert to perform a price pre-
diction by hand. It is reasonable to assume that the
number of outliers will decrease over time the system
is used because the database will increase. Indeed,
the methodology comprises nine distinct steps, where
we have evaluated different approaches. One aspect
that comes along with the smaller data sets is that in
these application cases expert knowledge needs to be
combined with machine learning techniques in many
steps like the generation of the data or the building of
the model for the estimation. But unlike expert sys-
tems, the result is a self-learning method which is able
to improve itself without consuming additional time
from the experts. This illustrates that small databases
even with a high variety, which comes along with
small batches in Industry 4.0, is a challenge that can
be mastered using the presented framework.
REFERENCES
Burrows, S., Stein, B., Frochte, J., Wiesner, D., and M
¨
uller,
K. (2011). Simulation data mining for supporting
bridge design. In Proceedings of the Ninth Aus-
tralasian Data Mining Conference-Volume 121, pages
163–170. Australian Computer Society, Inc.
Chougule, R. and Ravi, B. (2006). Casting cost estimation
in an integrated product and process design environ-
ment. International Journal of Computer Integrated
Manufacturing, 19(7):676–688.
Denkena, B., Lorenzen, L.-E., and Sch
¨
urmeyer, J. (2009).
Rule-based quotation costing of pressure die casting
moulds. Production Engineering, 3(1):87–94.
Kuschicke, F., Thiele, T., Meisen, T., and Jeschke, S.
(2017). A data-based method for industrial big data
project prioritization. In Proc. of the Int. Confer-
ence on Big Data and Internet of Thing, BDIOT2017,
pages 6–10, New York, NY, USA. ACM.
Li, P. and Niggemann, O. (2018). A data provenance based
architecture to enhance the reliability of data analy-
sis for industry 4.0. In 2018 IEEE 23rd Int. Confer-
ence on Emerging Technologies and Factory Automa-
tion (ETFA), volume 1, pages 1375–1382. IEEE.
Mukherjee, N., Ravi, B., et al. (2005). An integrated frame-
work for die and mold cost estimation using design
features and tooling parameters. The international
journal of advanced manufacturing technology, 26(9-
10):1138–1149.
Pedregosa, F., Varoquaux, G., Gramfort, A., Michel, V.,
Thirion, B., Grisel, O., Blondel, M., Prettenhofer,
P., Weiss, R., Dubourg, V., Vanderplas, J., Passos,
A., Cournapeau, D., Brucher, M., Perrot, M., and
Duchesnay, E. (2011). Scikit-learn: Machine learning
in Python. Journal of Machine Learning Research,
12:2825–2830.
Saltelli, A., Ratto, M., Andres, T., Campolongo, F., Cari-
boni, J., Gatelli, D., Saisana, M., and Tarantola, S.
(2008). Global sensitivity analysis: the primer. John
Wiley & Sons.
Wang, H., Ruan, X.-Y., and Zhou, X. (2003). Research on
injection mould intelligent cost estimation system and
key technologies. The International Journal of Ad-
vanced Manufacturing Technology, 21(3):215–222.
Windmann, S. and Niggemann, O. (2015). Data-driven as-
sistance functions for industrial automation systems.
In Journal of Physics: Conference Series, volume
659, page 012045. IOP Publishing.
Zhang, C. and Chen, T. (2001). Efficient feature extraction
for 2d/3d objects in mesh representation. In Proceed-
ings 2001 International Conference on Image Pro-
cessing (Cat. No. 01CH37205), volume 3, pages 935–
938. IEEE.
Case Study on Model-based Application of Machine Learning using Small CAD Databases for Cost Estimation
265