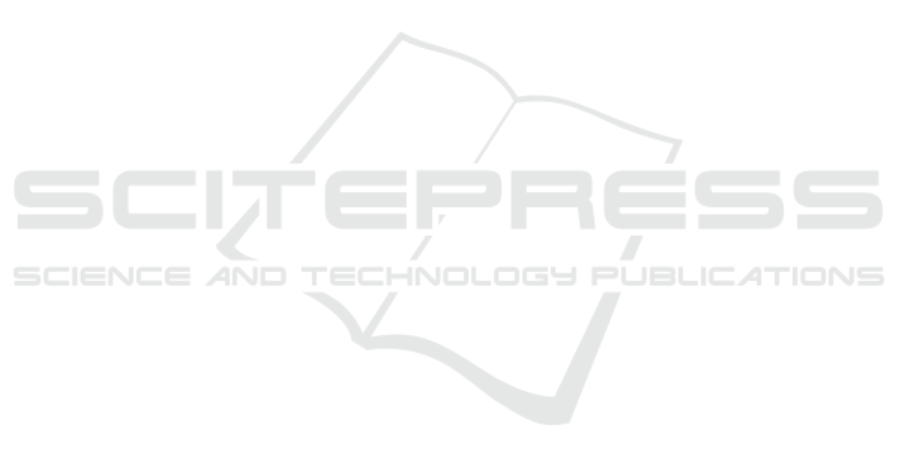
Castelli, M., Silva, S., and Vanneschi, L. (2015a). A
c++ framework for geometric semantic genetic pro-
gramming. Genetic Programming and Evolvable Ma-
chines, 16(1):73–81.
Castelli, M., Trujillo, L., and Vanneschi, L. (2015b). Energy
consumption forecasting using semantic-based ge-
netic programming with local search optimizer. Com-
putational intelligence and neuroscience, 2015:57.
Castelli, M., Trujillo, L., Vanneschi, L., Silva, S., et al.
(2015c). Geometric semantic genetic programming
with local search. In Proceedings of the 2015 An-
nual Conference on Genetic and Evolutionary Com-
putation, pages 999–1006. ACM.
Castelli, M., Vanneschi, L., and De Felice, M. (2015d).
Forecasting short-term electricity consumption using
a semantics-based genetic programming framework:
The south italy case. Energy Economics, 47:37–41.
Castelli, M., Vanneschi, L., Manzoni, L., and Popovi
ˇ
c, A.
(2016a). Semantic genetic programming for fast and
accurate data knowledge discovery. Swarm and Evo-
lutionary Computation, 26:1–7.
Castelli, M., Vanneschi, L., and Popovi
ˇ
c, A. (2015e). Pre-
dicting burned areas of forest fires: an artificial intel-
ligence approach. Fire ecology, 11(1):106–118.
Castelli, M., Vanneschi, L., and Popovi
ˇ
c, A. (2016b). Pa-
rameter evaluation of geometric semantic genetic pro-
gramming in pharmacokinetics. International Journal
of Bio-Inspired Computation, 8(1):42–50.
Castelli, M., Vanneschi, L., and Silva, S. (2014). Prediction
of the unified parkinson’s disease rating scale assess-
ment using a genetic programming system with ge-
ometric semantic genetic operators. Expert Systems
with Applications, 41(10):4608–4616.
Chang, C.-C. and Lin, C.-J. (2011). LIBSVM: A library for
support vector machines. ACM Transactions on Intel-
ligent Systems and Technology, 2:27:1–27:27. Soft-
ware available at http://www.csie.ntu.edu.tw/
˜
cjlin/libsvm.
Cortes, C. and Vapnik, V. (1995). Support-vector networks.
Machine Learning, 20(3):273–297.
Dua, D. and Karra Taniskidou, E. (2017). UCI machine
learning repository.
Keerthi, S. S. and Lin, C.-J. (2003). Asymptotic behaviors
of support vector machines with gaussian kernel. Neu-
ral Computation, 15(7):1667–1689.
Koza, J. R. (1992). Genetic Programming: On the Pro-
gramming of Computers by Means of Natural Selec-
tion. MIT Press, Cambridge, MA, USA.
Lin, H.-T. and Lin, C.-J. (2003). A study on sigmoid ker-
nels for svm and the training of non-psd kernels by
smo-type methods. Technical report, Department of
Computer Science, National Taiwan University.
Moraglio, A., Krawiec, K., and Johnson, C. (2012). Ge-
ometric semantic genetic programming. In Coello,
C., Cutello, V., Deb, K., Forrest, S., Nicosia, G., and
Pavone, M., editors, Parallel Problem Solving from
Nature - PPSN XII, volume 7491 of Lecture Notes in
Computer Science, pages 21–31. Springer Berlin Hei-
delberg.
Ruberto, S., Vanneschi, L., Castelli, M., and Silva, S.
(2014). Esagp–a semantic gp framework based on
alignment in the error space. In European Conference
on Genetic Programming, pages 150–161. Springer.
Vanneschi, L., Bakurov, I., and Castelli, M. (2017). An ini-
tialization technique for geometric semantic gp based
on demes evolution and despeciation. In Evolutionary
Computation (CEC), 2017 IEEE Congress on, pages
113–120. IEEE.
Vanneschi, L. and Castelli, M. (2019). Multilayer percep-
trons. In Ranganathan, S., Gribskov, M., Nakai, K.,
and Sch
¨
onbach, C., editors, Encyclopedia of Bioinfor-
matics and Computational Biology, pages 612 – 620.
Academic Press, Oxford.
Vanneschi, L., Silva, S., Castelli, M., and Manzoni, L.
(2014). Geometric semantic genetic programming for
real life applications. In Genetic programming theory
and practice xi, pages 191–209. Springer.
Vanschoren, J., van Rijn, J. N., Bischl, B., and Torgo,
L. (2013). Openml: Networked science in machine
learning. SIGKDD Explorations, 15(2):49–60.
ECTA 2019 - 11th International Conference on Evolutionary Computation Theory and Applications
48