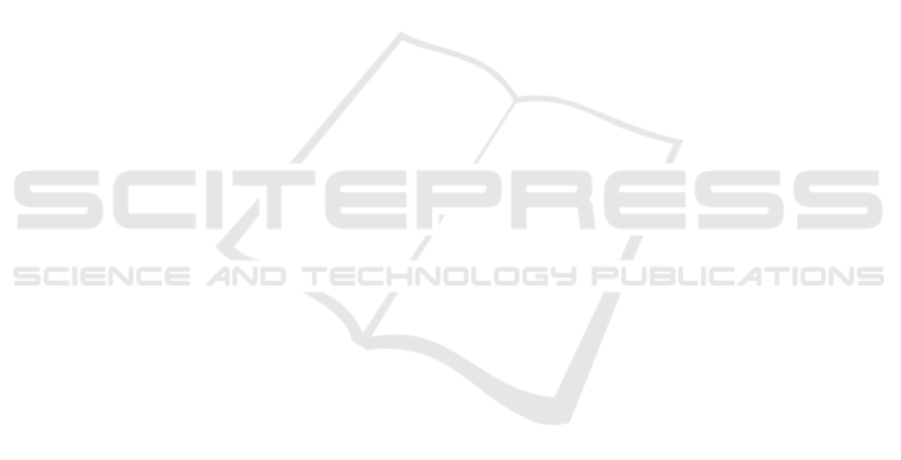
REFERENCES
B
¨
ack, T. and Sch
¨
utz, M. (1996). Intelligent mutation rate
control in canonical genetic algorithm. Proc. of the
International Symposium on Methodology for Intelli-
gent systems, pages 158–167.
Benedetti, A., Farina, M., and Gobbi, M. (2006). Evolu-
tionary multiobjective industrial design: the case of a
racing care tire-suspension system. IEEE Transaction
on evolutionary Computation, 10(3):230–244.
Beume, N., Naujoks, B., and Emmerich, M. (2007). Mul-
tiobjective selection based on dominated hypervol-
ume. European Journal of Operational Research,
18(3):1653–1669.
Boolong, K., Chaiyaratana, N., and Maneeratana, K.
(2010). Improved compressed-objective genetic algo-
rithm: COGA-II. International Conference on Evolu-
tionary Computation (ICEC), 1:95–103.
Deb, K. and Jain, H. (2014). An evolutionary many-
objective optimization algorithm using reference-
point-based nondominated sorting approach, Part i:
Solving problems with box constraints. IEEE transac-
tions on Evolutionary Computation, 18(4):577–601.
Deb, K., Pratap, A., Agarwal, S., and Meyarivan, T. (2002).
A fast and elitist multiobjective genetic algorithm:
NSGA-II. IEEE Transactions on Evolutionary Com-
putation, 6(2):182–197.
Diaz-Manriquez, A., Toscano, G., Barron-Zambrano, J.,
and et al. (2016). A review of surrogate assisted multi-
objective evolutionary algorithms. Computational In-
telligence and Neuroscience.
Guruprasad, K., Reddy, B., and Pandit, M. (1990). Corre-
lation between stability of a protein and its dipeptide
composition: A novel approach for predicting in vivo
stability of a protein from its primary structure. Pro-
tein Engineering, 4(2):155–161.
He, Z., Yen, G., and Zhang, J. (2014). Fuzzy-based
pareto optimality for many-objective evolutionary al-
gorithms. IEEE Transactions on Evolutionary Com-
putation, 18(2):269–285.
Hopp, T. and Woods, K. (1983). A computer program for
predicting protein antigenic determinants. Mol. Im-
munol., 20(4):483–489.
Ishihuchi, H., Akedo, N., and Ohyanagi, H. (2011). Be-
havior of emo algorithms on many-objective opti-
mization problems with correlated objectives. IEEE
Congress on Evolutionary Computation (CEC 2011),
pages 1465–1472.
Krause, T., R
¨
ockendorf, N., El-Sourani, N., and et al.
(2018). Breeding cell penetrating peptides: Optimiza-
tion of cellular uptake by a function-driven evolution-
ary process. Bioconjug Chem.
Laumanns, M., Thiele, L., Deb, K., and Zitzler, E. (2002).
Combining convergence and diversity in evolutionary
multiobjective optimization. Evolutionary Computa-
tion, 10(3):263–282.
Lee, Z.-Z., Wang, Y., and Huang, P.-Q. (2018). And:
A many-objective evolutionary algorithm with angle-
based selection and shift-based density estimation. In-
formation Sciences, Elsevier.
Liu, H., Chen, L., Zhang, Q., and Deb, K. (2017). Adap-
tively allocating search effort in challenging many-
objective optimization problems. IEEE Transactions
on Evolutionary Computation, 22(3):433–448.
Lopez, E. and Coello, C. (2016). idg
+
-EMOA: A multi-
objective evolutionary algorithm based on igd
+
. IEEE
Congress on Evolutionary Computation (CEC), pages
996–1006.
Maneeratano, C., Boonlang, K., and Chaigaratana, N.
(2006). Compressed-objective genetic algorithm. Par-
allel Problem solving from Nature - PPSN IX, LNCS
4193:473–482.
Nebro, A. and Durillo, J. (2018). jmetal: Metaheuristic Al-
gorithms in Java.
Nicolotti, D., Giangreco, I., and Introcasa, A. (2011).
Strategies of multi-objective optimization in drug dis-
covery and development. Expert Opin Drug Discov.,
6(9).
Prlic, A., Yates, A., Spencer, E., and et al. (2018). BioJava:
an open-source framework for bioinformatics.
R
¨
ockendorf, N. and Borschbach, M. (2012). Molecular evo-
lution of peptide ligands with custom-tailored charac-
teristics. PLOS Computational Biology, 8(12).
Rosenthal, S. and Borschbach, M. (2017a). Average cuboid
volume as a convergence indicator and selection cri-
terion for multi-objective biochemical optimization.
EVOLVE - A Bridge between Probability, Set Oriented
Numerics and Evolutionary Computation VII.
Rosenthal, S. and Borschbach, M. (2017b). Design perspec-
tives of an evolutionary process for multi-objective
molecular optimization. Proc. of the 9th Interna-
tional Conference on Evolutionary Multi-Criterion
Optimization (EMO 2017), LNCS 10173, pages 529–
544.
Wang, R., Zhang, Q., and Zhang, T. (2016).
Decomposition-based algorithms using pareto
adaptive scalarization methods. IEEE Transactions
on Evolutionary Computation, 20(6):821–837.
Yang, S., Li, M., Liu, X., and Zheng, J. (2013). A grid-
based evolutionary algorithm for many- objective op-
timization. IEEE Transactions on Evolutionary Com-
putation, 17(5):721–736.
Yuan, Y., Xu, H., Wang, B., and Yao, X. (2016). Balanc-
ing convergence and diversity in decomposition-based
many-objective optimization. IEEE Transactions on
Evolutionary Computation, 20(2):180–198.
Zhang, Q. and Li, H. (2007). MOEA/D: A multi-objective
evolutionary algorithm based on decomposition. IEEE
Trans. on Evolutionary Computation, 11(6):712–731.
Zitzler, E. and K
¨
unzli, S. (2004). Indicator-based selection
in multi-objective search. Parallel Problem Solving
from Nature - PPSN VIII, LNCS 3242:832–842.
Zitzler, E., Laumanns, M., and Thiele, L. (2002). SPEA2:
improving the strength pareto evolutionary algorithm
for multi-objective optimization. Evolutionary Meth-
ods for Design, Optimisations and Control, pages 19–
26.
Zou, X., Chen, Y., Liu, M., and Kang, L. (2008). A new
evolutionary algorithm for solving many-objective op-
timization problems. IEEE Transactions on Sys-
tems, Man, and Cybernetics, Part B (Cybernetics),
38(5):1402–1412.
ECTA 2019 - 11th International Conference on Evolutionary Computation Theory and Applications
58