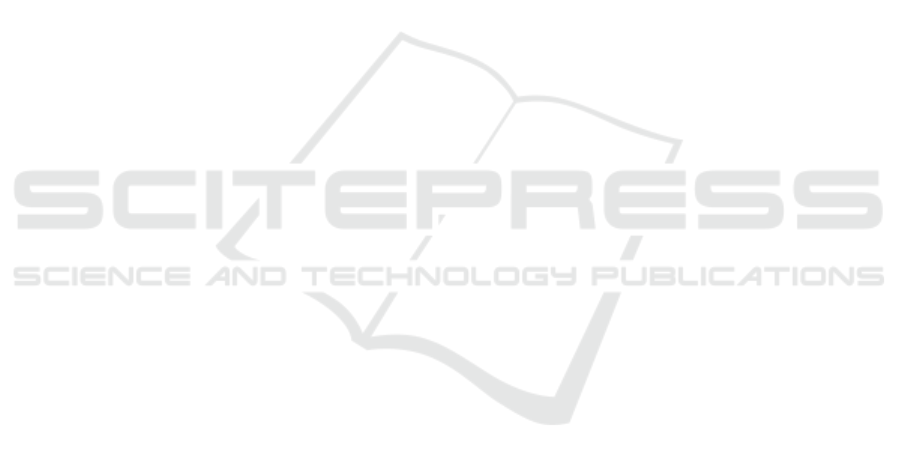
The resulting item information for the sample sets was
published as a report. This report was then evaluated
by experts of the art historical domain. The evalua-
tion had to verify the correctness of the interlinks as
well as the correctness of the order of the concept an-
notations. As already seen in the presented examples,
the interlinks contain incorrectly disambiguated con-
cepts. This not only created incorrect concept anno-
tations, but also influenced the relevancy scores. We
ignored the incorrect concepts from the artwork de-
scriptions and evaluated the remaining correct con-
cept annotations regarding the correctness of the or-
der. It showed that the correct concept annotations
provide a valid description of the details of the content
regarding the relevance. The concluded concepts au-
tomatically extend the asserted descriptions and help
non-experts to understand the details using the rele-
vancy scoring. They can be used as a basis for re-
trieval in knowledge-based recommender systems.
5 CONCLUSION
In this paper we presented an approach for relevancy
scoring based on the interlinking of vocabularies. It
is not dependent on instance data and does not have
drawbacks regarding the size of the collection. It
will work for small collections well as for large ones.
However, it is dependent on the vocabularies selected
for interlinking and the quality of the interlinks. To
help users to better understand the meaning of art-
works, the two vocabularies must have different se-
mantic expressivity, so the concluded information is
represented using a view that is understandable to
non-experts. The data model can be generated au-
tomatically by vocabulary interlinking and calculat-
ing information content scores for the interlinked con-
cepts. We performed a interdisciplinary evaluation to
verify the approach. It showed that the interlinking re-
sulted in false positive concept annotations that were
incorrectly disambiguated. We ignored these false
positives for the further evaluation and only consid-
ered the correct ones for relevancy scoring. It showed
that the order of the correct concept annotations for
the sample artworks was valid. However, the in-
correct interlinks influence the relevancy scores and
might result in a changed order for some artwork de-
scriptions regarding correct concepts. This, as well
as improving the disambiguation, has to be evaluated
in future work. The next step is the extension of the
implemented prototype for recommendations where
the options are given by the scored concept annota-
tions. We can then evaluate how these artwork de-
scriptions help visitors to navigate the museum col-
lections based on their interests by using the scored
concepts for retrieval and as tweaking options.
REFERENCES
Alistair Miles, S. B. (2009). Skos simple knowledge organi-
zation system reference. http://www.w3.org/TR/skos-
reference/. [Online; accessed 31-March-2015].
Berners-Lee, T., Hendler, J., Lassila, O., et al. (2001). The
semantic web. Scientific american, 284(5):28–37.
Burke, R. (2000). Knowledge-based recommender systems.
In Encyclopedia of Library and Information Systems,
page 2000. Marcel Dekker.
Burke, R. D., Hammond, K. J., and Young, B. C. (1997).
The findme approach to assisted browsing. IEEE
Expert: Intelligent Systems and Their Applications,
12:32–40.
Dijkshoorn, C., Jongma, L., Aroyo, L., van Ossenbruggen,
J., Schreiber, G., ter Weele, W., and Wielemaker, J.
(2014). The rijksmuseum collection as linked data.
Semantic Web, (Preprint):1–10.
Felfernig, A. and Burke, R. (2008). Constraint-based rec-
ommender systems: Technologies and research is-
sues. In Proceedings of the 10th International Confer-
ence on Electronic Commerce, ICEC ’08, pages 3:1–
3:10, New York, NY, USA. ACM.
Gicquel, P.-Y. and Lenne, D. (2013). Semantic and Con-
textual Proximities for Informal Learning: The Case
Study of Museum Visits. International Journal of
Computer Applications, 69(26):12134–8410.
Hollink, L., Schreiber, G., Wielemaker, J., and Wielinga, B.
(2003). Semantic annotation of image collections. In
Second International Conference for Knowledge Cap-
ture (KCAP2003) Workshop on Knowledge Markup
and Semantic Annotation.
Hyvönen, E., Ruotsalo, T., Haggström, T., Salminen,
M., Junnila, M., Virkkilä, M., Haaramo, M.,
Mäkelä, E., Kauppinen, T., and Viljanen, K. (2007).
CultureSampo–Finnish Culture on the Semantic Web:
The Vision and First Results, pages 33–58. LIT Ver-
lag, Berlin.
Petersen, T. (1990). Art & architecture thesaurus. Ox-
ford University Press. http://www.getty.edu/research/
tools/vocabulary/aat/.
Resnik, P. (1995). Using information content to evaluate se-
mantic similarity in a taxonomy. In Proceedings of the
14th International Joint Conference on Artificial In-
telligence - Volume 1, IJCAI’95, pages 448–453, San
Francisco, CA, USA. Morgan Kaufmann Publishers
Inc.
Van de Waal, H. (1974). Iconclass: an iconographic classi-
fication system, volume 2. North-Holland.
Relevancy Scoring for Knowledge-based Recommender Systems
239