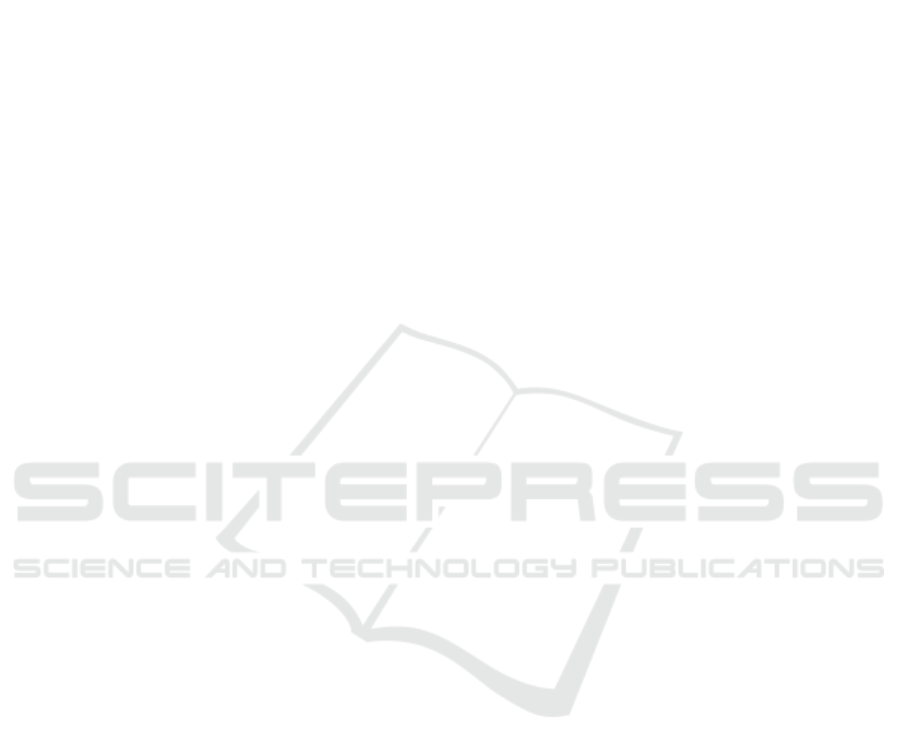
Causal Learning to Discover Supply Chain Vulnerability
Ying Zhao, Jacob Jones and Douglas MacKinnon
Naval Postgraduate School, Monterey, CA, U.S.A.
Keywords:
Causal Learning, Counterfactual Analysis, Cause and Effect, Supply Chain Vulnerability, Associations,
Correlations, Lexical Link Analysis, Data Mining.
Abstract:
This paper illustrates a methodology of causal learning using pair-wise associations discovered from data.
Taking advantage of a U.S. Department of Defense supply chain use case, this causal learning approach was
substantiated and demonstrated in the application of discovering supply chain vulnerabilities. By integrating
lexical link analysis, a data mining tool used to discover relationships in specific vocabularies or lexical terms
with pair-wise causal learning, supply chain vulnerabilities were recognized. Evaluation of results from this
methodology reveals supply chain opportunities, while exposing weaknesses to develop a more responsive and
efficient supply chain system.
1 INTRODUCTION
U.S. Department of Defense (DoD) supply chains are
large, complex, and deal with unpredictable demand
signals from a variety of sources. DoD supply chains
account for over 100,000 suppliers, 2,000 control-
ling systems, 19 maintenance depots, 25 distribution
depots, and over 30,000 customer sites, and manag-
ing an inventory of $92.6 billion in 2015 (Haraburda,
2016). Private sector supply chains focus on profit
margins and growth potential. However, the DoD’s
goal is to ensure that readiness of equipment and per-
sonnel are maintained at a rate sufficient to engage
enemy combatants in both peace and wartime (Wil-
hite et al., 2014). Keeping the warfighters at a high
state of readiness is the main objective and a ma-
jor factor in the design of the supply chains, while
cost is a constraining factor professionals must man-
age and minimize it (Haraburda, 2016; Wilhite et al.,
2014). Therefore, military supply chains measure
themselves using two metrics: response time, which
is how quickly the force can be equipped with the re-
quired item; and effectiveness, ensuring the right sup-
ply asset is given at the right time (Jones, 2018).
2 IMPORTANCE OF CAUSAL
LEARNING
A unique aspect to military supply chains is that they
support complex weapon systems. They involve cut-
ting edge technology throughout the long life cycles
of the weapon systems and must be ready for com-
bat at any given time. To achieve the highest readi-
ness level, DoD supply chain organizations have to
constantly reinvent themselves to improve the sup-
ply chains and logistic processes by utilizing new
technology, process, and concepts, such as big data
mining, machine learning, and artificial intelligence.
While applying the new technologies, decision mak-
ers are interested in discovering and understanding
the reasons and causes from data which can address
the gaps and vulnerabilities of the current systems,
and where and how to make necessary improvements.
This calls for systematic causal learning to discover
supply chain vulnerabilities. For example, lengthy
supply delivery time could be a vulnerability. To
strengthen supply chains, causes for those vulnerabil-
ities must be discovered.
3 DATA DESCRIPTION
This project analyzed MV-22 Osprey supply chain
data, a multi-mission, tiltrotor military aircraft with
both vertical takeoff and landing, and short takeoff
and landing capabilities. It is designed to combine
the functionality of a conventional helicopter with the
long-range, high-speed cruise performance of a tur-
boprop aircraft, necessary to conduct sea basing and
expeditionary operations (V-22, 2018).
To assess the MV-22 Osprey supply chain, this re-
Zhao, Y., Jones, J. and MacKinnon, D.
Causal Learning to Discover Supply Chain Vulnerability.
DOI: 10.5220/0008070503050309
In Proceedings of the 11th International Joint Conference on Knowledge Discovery, Knowledge Engineering and Knowledge Management (IC3K 2019), pages 305-309
ISBN: 978-989-758-382-7
Copyright
c
2019 by SCITEPRESS – Science and Technology Publications, Lda. All rights reserved
305