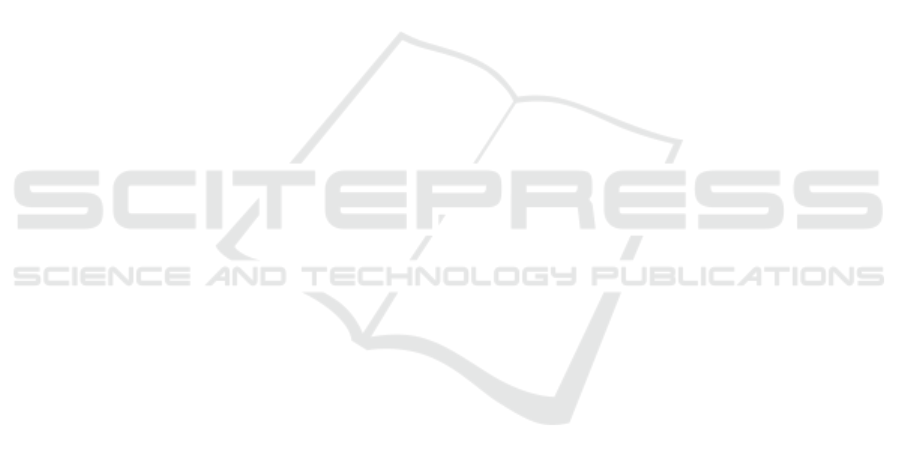
worldwide for 36 cancers in 185 countries. CA: a
cancer journal for clinicians, 68, 394-424.
Brockwell, P. J., Davis, R. A. & Calder, M. V. 2002.
Introduction to time series and forecasting, Springer.
Carlstein, E. 1992. Resampling techniques for stationary
time series: some recent developments. IMA
VOLUMES IN MATHEMATICS AND ITS
APPLICATIONS, 45, 75-75.
Chapman, W. W., Bridewell, W., Hanbury, P., Cooper, G.
F. & Buchanan, B. G. 2001. A simple algorithm for
identifying negated findings and diseases in discharge
summaries. Journal of biomedical informatics, 34, 301-
310.
Cuturi, M. & Blondel, M. Soft-DTW: a differentiable loss
function for time-series. Proceedings of the 34th
International Conference on Machine Learning-
Volume 70, 2017. JMLR. org, 894-903.
Cuturi, M., Vert, J.-P., Birkenes, O. & Matsui, T. A kernel
for time series based on global alignments. 2007 IEEE
International Conference on Acoustics, Speech and
Signal Processing-ICASSP'07, 2007. IEEE, II-413-II-
416.
De Bruijn, B., Cherry, C., Kiritchenko, S., Martin, J. & Zhu,
X. 2011. Machine-learned solutions for three stages of
clinical information extraction: the state of the art at
i2b2 2010. Journal of the American Medical
Informatics Association, 18, 557-562.
Díaz, N. P. C., López, M. J. M., Vázquez, J. M. & Álvarez,
V. P. 2012. A machine‐learning approach to negation
and speculation detection in clinical texts. Journal of
the American society for information science and
technology, 63, 1398-1410.
Elkin, P. L., Brown, S. H., Bauer, B. A., Husser, C. S.,
Carruth, W., Bergstrom, L. R. & Wahner-Roedler, D.
L. 2005. A controlled trial of automated classification
of negation from clinical notes. BMC medical
informatics and decision making, 5, 13.
Etzioni, R., Tsodikov, A., Mariotto, A., Szabo, A., Falcon,
S., Wegelin, J., Karnofski, K., Gulati, R., Penson, D. F.
& Feuer, E. 2008. Quantifying the role of PSA
screening in the US prostate cancer mortality decline.
Cancer Causes & Control, 19, 175-181.
Gillum, R. F. 2013. From papyrus to the electronic tablet: a
brief history of the clinical medical record with lessons
for the digital age. The American journal of medicine,
126, 853-857.
Gindl, S., Kaiser, K. & Miksch, S. 2008. Syntactical
negation detection in clinical practice guidelines.
Studies in health technology and informatics, 136, 187.
Goldin, I. & Chapman, W. W. Learning to detect negation
with ‘not’in medical texts. Proc Workshop on Text
Analysis and Search for Bioinformatics, ACM SIGIR,
2003.
Gordon, T., Castelli, W. P., Hjortland, M. C., Kannel, W.
B. & Dawber, T. R. 1977. High density lipoprotein as a
protective factor against coronary heart disease: the
Framingham Study. The American journal of medicine,
62, 707-714.
Gunter, T. D. & Terry, N. P. 2005. The emergence of
national electronic health record architectures in the
United States and Australia: models, costs, and
questions. Journal of medical Internet research, 7, e3.
Henry, J., Pylypchuk, Y., Searcy, T. & Patel, V. 2016.
Adoption of electronic health record systems among US
non-federal acute care hospitals: 2008-2015. ONC data
brief, 35, 1-9.
Honnibal, M. & Johnson, M. An improved non-monotonic
transition system for dependency parsing. Proceedings
of the 2015 Conference on Empirical Methods in
Natural Language Processing, 2015. 1373-1378.
Jain, N. L. & Friedman, C. Identification of findings
suspicious for breast cancer based on natural language
processing of mammogram reports. Proceedings of the
AMIA Annual Fall Symposium, 1997. AMIA, 829.
Košmelj, K. & Batagelj, V. 1990. Cross-sectional approach
for clustering time varying data. Journal of
Classification, 7, 99-109.
Kreindler, D. M. & Lumsden, C. J. 2016. The effects of the
irregular sample and missing data in time series
analysis. Nonlinear Dynamical Systems Analysis for the
Behavioral Sciences Using Real Data. CRC Press.
Lee, C. F., Lee, J. C. & Lee, A. C. 2000. Statistics for
business and financial economics, Springer.
Lee, E. K., Wang, Y., Hagen, M. S., Wei, X., DAVIS, R.
A. & Egan, B. M. Machine Learning: Multi-site
evidence-based best practice discovery. International
Workshop on Machine Learning, Optimization, and
Big Data, 2016. Springer, 1-15.
Lee, E. K., Wang, Y., Hagen, M. S., Li, Z., Wei, X.,
DAVIS, R. A. & EGAN, B. M. A Machine Learning
Framework for Multi-site Evidence-based Best Practice
Discovery. 2019 Caterpillar & INFORMS Innovative
Applications in Analytics Award 2
nd
place. To appear
in INFORMS Journal on Applied Analytics.
Lenart, M., Mascarenhas, N., Xiong, R. & FLOWER, A.
Identifying risk of progression for patients with Chronic
Kidney Disease using clustering models. 2016 IEEE
Systems and Information Engineering Design
Symposium (SIEDS), 2016. IEEE, 221-226.
Li, L., Chase, H. S., Patel, C. O., Friedman, C. & Weng, C.
Comparing ICD9-encoded diagnoses and NLP-
processed discharge summaries for clinical trials pre-
screening: a case study. AMIA Annual Symposium
Proceedings, 2008. American Medical Informatics
Association, 404.
Liao, T. W. 2007. A clustering procedure for exploratory
mining of vector time series. Pattern Recognition, 40,
2550-2562.
Long, W. Extracting diagnoses from discharge summaries.
AMIA annual symposium proceedings, 2005. AMIA,
470.
Marlin, B. M., Kale, D. C., Khemani, R. G. & Wetzel, R.
C. Unsupervised pattern discovery in electronic health
care data using probabilistic clustering models. 2012.
ACM, 389-398.
Marteau, P.-F. 2009. Time warp edit distance with stiffness
adjustment for time series matching. IEEE
Transactions on Pattern Analysis and Machine
Intelligence, 31, 306-318.
KDIR 2019 - 11th International Conference on Knowledge Discovery and Information Retrieval
320