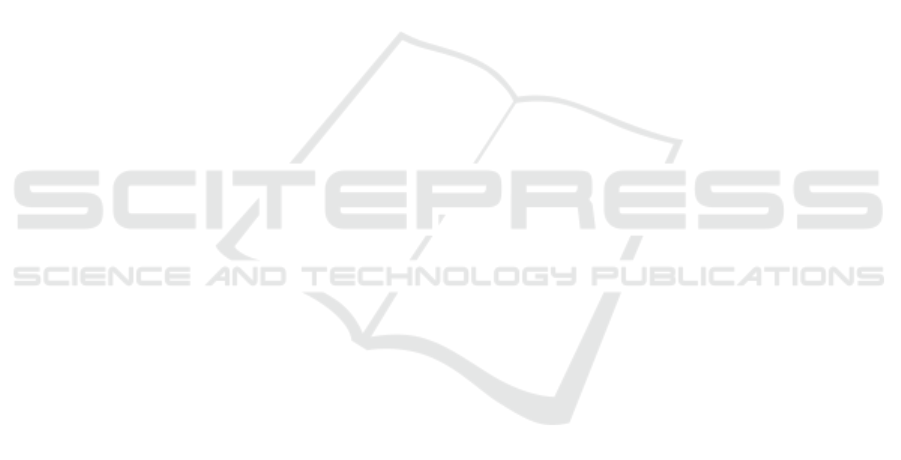
International Conference on Business Process Man-
agement, pages 3–14. Springer.
de Medeiros, A. K. A. (2006). Genetic process mining. PhD
thesis, Eindhoven university of technology.
Durillo, J. J. and Nebro, A. J. (2011). jmetal: A java frame-
work for multi-objective optimization. Advances in
Engineering Software, 42(10):760–771.
Dyer, D. W. (2010). Watchmaker framework for evolution-
ary computation version 0.7.1.
Emmerich, M. T. and Deutz, A. H. (2018). A tutorial on
multiobjective optimization: fundamentals and evolu-
tionary methods. Natural computing, 17(3):585–609.
Gadhvi, B., Savsani, V., and Patel, V. (2016). Multi-
objective optimization of vehicle passive suspension
system using nsga-ii, spea2 and pesa-ii. Procedia
Technology, 23:361–368.
G
¨
unther, C. W. and Van Der Aalst, W. M. (2007).
Fuzzy mining–adaptive process simplification based
on multi-perspective metrics. In International con-
ference on business process management, pages 328–
343. Springer.
Gupta, E. (2014). Process mining a comparative study. In-
ternational Journal of Advanced Research in Com-
puter and Communications Engineering, 3(11):5.
Ishibuchi, H., Imada, R., Setoguchi, Y., and Nojima, Y.
(2017). Hypervolume subset selection for triangular
and inverted triangular pareto fronts of three-objective
problems. In Proceedings of the 14th ACM/SIGEVO
Conference on Foundations of Genetic Algorithms,
pages 95–110. ACM.
Jin, Y. (2012). Advanced fuzzy systems design and applica-
tions, volume 112. Physica.
Kalyanmoy, D., Pratap, A., Agarwal, S., and Meyarivan, T.
(2002). A fast and elitist multiobjective genetic al-
gorithm: Nsga-ii. IEEE Transactions on evolutionary
computation, 6(2):182–197.
Knowles, J. D. and Corne, D. W. (2000). Approximating
the nondominated front using the pareto archived evo-
lution strategy. Evolutionary computation, 8(2):149–
172.
Knowles, J. D., Thiele, L., and Zitzler, E. (2006). A tutorial
on the performance assessment of stochastic multiob-
jective optimizers. TIK-Report, 214.
Li, M., Yang, S., and Liu, X. (2014). Diversity compari-
son of pareto front approximations in many-objective
optimization. IEEE Transactions on Cybernetics,
44(12):2568–2584.
Marler, R. T. and Arora, J. S. (2004). Survey of
multi-objective optimization methods for engineer-
ing. Structural and multidisciplinary optimization,
26(6):369–395.
Marler, R. T. and Arora, J. S. (2010). The weighted
sum method for multi-objective optimization: new in-
sights. Structural and multidisciplinary optimization,
41(6):853–862.
Riquelme-Granada, N., Von L
¨
ucken, C., and Baran, B.
(2015). Performance metrics in multi-objective op-
timization. In 2015 Latin American Computing Con-
ference (CLEI), pages 1–11. IEEE.
Schott, J. R. (1995). Fault Tolerant Design Using Single and
Multicriteria Genetic Algorithm Optimization. PhD
thesis, AIR FORCE INST OF TECH WPAFB AFB
OH.
Tamaki, H., Kita, H., and Kobayashi, S. (1996). Multi-
objective optimization by genetic algorithms: A re-
view. In Proceedings of IEEE international confer-
ence on evolutionary computation, pages 517–522.
Tan, K. C., Lee, T. H., and Khor, E. F. (2002). Evolution-
ary algorithms for multi-objective optimization: Per-
formance assessments and comparisons. Artificial in-
telligence review, 17(4):251–290.
Tiwari, A., Turner, C. J., and Majeed, B. (2008). A re-
view of business process mining: state-of-the-art and
future trends. Business Process Management Journal,
14(1):5–22.
Tsai, C.-Y., Jen, H.-Y., and Chen, I.-C. (2010). Time-
interval process model discovery and validation—a
genetic process mining approach. Applied Intelli-
gence, 33:54–66.
Van der Aalst, W. M., De Medeiros, A. A., and Weijters, A.
(2005). Genetic process mining. In International con-
ference on application and theory of petri nets, pages
48–69. Springer.
van der Aalst, W. M., Reijers, H. A., Weijters, A. J., van
Dongen, B. F., De Medeiros, A. A., Song, M., and
Verbeek, H. (2007). Business process mining: An in-
dustrial application. Information Systems, 32(5):713–
732.
van der Aalst, W. M. P. (2011). Process Mining: Discov-
ery, Conformance and Enhancement of Business Pro-
cesses. Springer, 1st edition.
van der Aalst, W. M. P., Hee, K., Ter, A., Sidorova, N.,
M. W. Verbeek, H., Voorhoeve, M., and Wynn, M.
(2011). Soundness of workflow nets: Classification,
decidability, and analysis. Formal Aspects of Comput-
ing, 23:333–363.
van der Aalst, W. M. P., Weijters, A., and M
˘
arus¸ter, L.
(2004). Workflow mining: Discovering process mod-
els from event logs. IEEE Transactions on Knowledge
and Data Engineering, 16(9):1128–1142.
Van Veldhuizen, D. A. (1999). Multiobjective evolutionary
algorithms: classifications, analyses, and new inno-
vations. PhD thesis, AIR FORCE INST OF TECH
WPAFB OH SCHOOL OF ENGINEERING.
Verbeek, H. (2016). 9th international workshop on business
process intelligence. Last accessed 25 January 2017.
Verbeek, H., Buijs, J. C., Van Dongen, B. F., and Van
Der Aalst, W. M. (2010). Xes, xesame, and prom 6.
In International Conference on Advanced Information
Systems Engineering, pages 60–75. Springer.
Weijters, A., van Der Aalst, W. M., and De Medeiros, A. A.
(2006). Process mining with the heuristics miner-
algorithm. Technische Universiteit Eindhoven, Tech.
Rep. WP, 166:1–34.
Zitzler, E., Laumanns, M., and Thiele, L. (2000). Improv-
ing the strength pareto evolutionary algorithm. EU-
ROGEN 2001, Evolutionary Methods for Design, Op-
timization and Control with Applications to Industrial
Problems, pages 95–100.
KDIR 2019 - 11th International Conference on Knowledge Discovery and Information Retrieval
100