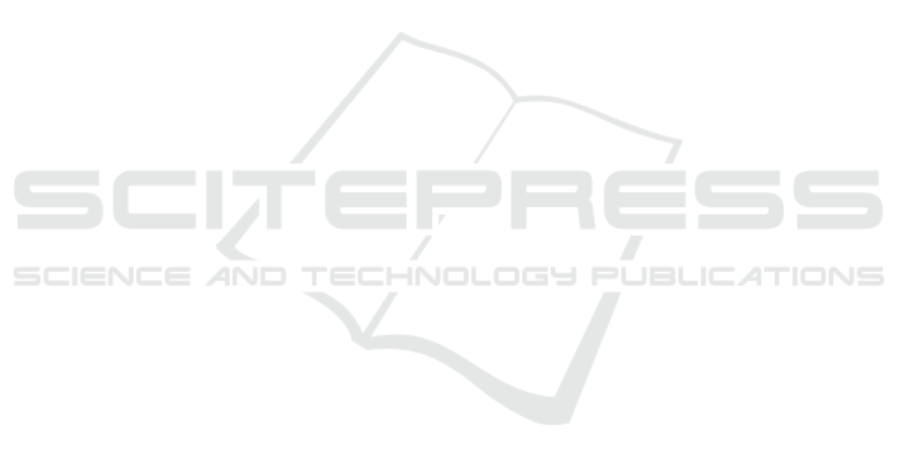
Krishnan, A., Zbilut, J. P., Tomita, M., and Giuliani, A.
(2008). Proteins as networks: usefulness of graph the-
ory in protein science. Current Protein and Peptide
Science, 9(1):28–38.
Livi, L., Del Vescovo, G., Rizzi, A., and Frattale Mas-
cioli, F. M. (2014). Building pattern recognition
applications with the spare library. arXiv preprint
arXiv:1410.5263.
Livi, L. and Sadeghian, A. (2016). Granular comput-
ing, computational intelligence, and the analysis of
non-geometric input spaces. Granular Computing,
1(1):13–20.
Martino, A., Giuliani, A., and Rizzi, A. (2018a). Gran-
ular computing techniques for bioinformatics pat-
tern recognition problems in non-metric spaces. In
Pedrycz, W. and Chen, S.-M., editors, Computational
Intelligence for Pattern Recognition, pages 53–81.
Springer International Publishing, Cham.
Martino, A., Rizzi, A., and Frattale Mascioli, F. M. (2017).
Efficient approaches for solving the large-scale k-
medoids problem. In Proceedings of the 9th Inter-
national Joint Conference on Computational Intelli-
gence - Volume 1: IJCCI,, pages 338–347. INSTICC,
SciTePress.
Martino, A., Rizzi, A., and Frattale Mascioli, F. M. (2018b).
Distance matrix pre-caching and distributed computa-
tion of internal validation indices in k-medoids clus-
tering. In 2018 International Joint Conference on
Neural Networks (IJCNN), pages 1–8.
Martino, A., Rizzi, A., and Frattale Mascioli, F. M.
(2019). Efficient approaches for solving the large-
scale k-medoids problem: Towards structured data.
In Sabourin, C., Merelo, J. J., Madani, K., and War-
wick, K., editors, Computational Intelligence: 9th In-
ternational Joint Conference, IJCCI 2017 Funchal-
Madeira, Portugal, November 1-3, 2017 Revised Se-
lected Papers, pages 199–219. Springer International
Publishing, Cham.
Neuhaus, M. and Bunke, H. (2007). Bridging the gap be-
tween graph edit distance and kernel machines, vol-
ume 68. World Scientific.
Pedrycz, W. (2005). Knowledge-based clustering: from
data to information granules. John Wiley & Sons.
Pedrycz, W. (2010). Human centricity in computing with
fuzzy sets: an interpretability quest for higher order
granular constructs. Journal of Ambient Intelligence
and Humanized Computing, 1(1):65–74.
Pedrycz, W. (2013). Proximity-based clustering: a search
for structural consistency in data with semantic blocks
of features. IEEE Transactions on Fuzzy Systems,
21(5):978–982.
Pedrycz, W. (2016). Granular computing: analysis and de-
sign of intelligent systems. CRC press.
Pedrycz, W. and Homenda, W. (2013). Building the
fundamentals of granular computing: A principle
of justifiable granularity. Applied Soft Computing,
13(10):4209 – 4218.
P˛ekalska, E. and Duin, R. P. (2005). The dissimilarity rep-
resentation for pattern recognition: foundations and
applications.
Richiardi, J., Achard, S., Bunke, H., and Van De Ville, D.
(2013). Machine learning with brain graphs: predic-
tive modeling approaches for functional imaging in
systems neuroscience. IEEE Signal Processing Mag-
azine, 30(3):58–70.
Riesen, K. and Bunke, H. (2008). Iam graph database
repository for graph based pattern recognition and ma-
chine learning. In Joint IAPR International Work-
shops on Statistical Techniques in Pattern Recognition
(SPR) and Structural and Syntactic Pattern Recogni-
tion (SSPR), pages 287–297. Springer.
Theodoridis, S. and Koutroumbas, K. (2008). Pattern
Recognition. Academic Press, 4 edition.
Tran, H.-N., Cambria, E., and Hussain, A. (2016). Towards
gpu-based common-sense reasoning: Using fast sub-
graph matching. Cognitive Computation, 8(6):1074–
1086.
Tsai, W.-H. and Fu, K.-S. (1979). Error-correcting isomor-
phisms of attributed relational graphs for pattern anal-
ysis. IEEE Transactions on systems, man, and cyber-
netics, 9(12):757–768.
Vishwanathan, S. V. N., Schraudolph, N. N., Kondor, R.,
and Borgwardt, K. M. (2010). Graph kernels. Journal
of Machine Learning Research, 11(Apr):1201–1242.
Wang, F. and Sun, J. (2015). Survey on distance metric
learning and dimensionality reduction in data mining.
Data Mining and Knowledge Discovery, 29(2):534–
564.
Wang, G., Yang, J., and Xu, J. (2017). Granular
computing: from granularity optimization to multi-
granularity joint problem solving. Granular Comput-
ing, 2(3):105–120.
Weinshall, D., Jacobs, D. W., and Gdalyahu, Y. (1999).
Classification in non-metric spaces. In Kearns, M. J.,
Solla, S. A., and Cohn, D. A., editors, Advances
in Neural Information Processing Systems 11, pages
838–846. MIT Press.
Yang, J., Wang, G., and Zhang, Q. (2018). Knowledge
distance measure in multigranulation spaces of fuzzy
equivalence relations. Information Sciences, 448:18–
35.
Yao, Y. (2016). A triarchic theory of granular computing.
Granular Computing, 1(2):145–157.
Yao, Y. and Zhao, L. (2012). A measurement theory view
on the granularity of partitions. Information Sciences,
213:1–13.
Yao, Y.-Y. (2008). The rise of granular computing. Journal
of Chongqing University of Posts and Telecommuni-
cations (Natural Science Edition), 20(3):299–308.
Zadeh, L. A. (1979). Fuzzy sets and information granu-
larity. Advances in fuzzy set theory and applications,
11:3–18.
Zadeh, L. A. (1997). Toward a theory of fuzzy information
granulation and its centrality in human reasoning and
fuzzy logic. Fuzzy sets and systems, 90(2):111–127.
Zaharia, M., Chowdhury, M., Franklin, M. J., Shenker, S.,
and Stoica, I. (2010). Spark: Cluster computing with
working sets. In Proceedings of the 2nd USENIX
Conference on Hot Topics in Cloud Computing, Hot-
Cloud’10, pages 10–10. USENIX Association.
NCTA 2019 - 11th International Conference on Neural Computation Theory and Applications
402