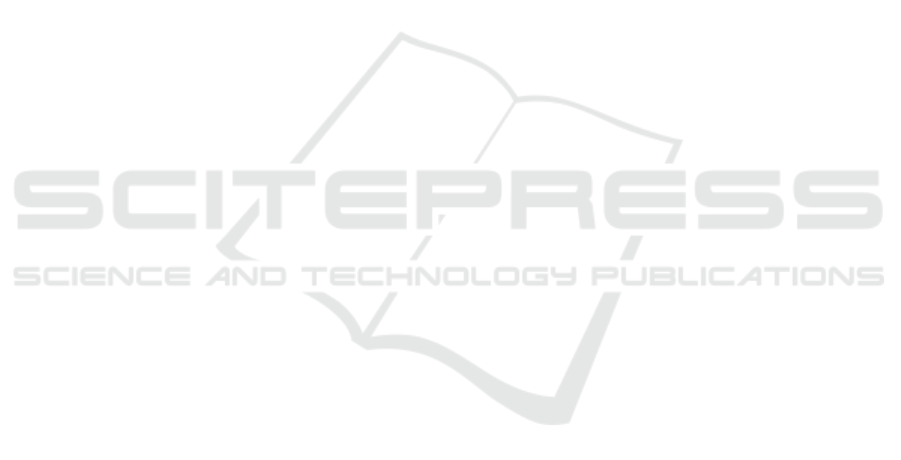
Fernandez-Tobias, I., Braunhofer, M., Elahi, M., Ricci, F.
& Cantador, I. 2016. Alleviating the new user problem
in collaborative filtering by exploiting personality
information. User Modeling and User-Adapted
Interaction, 26, 221-255.
Flach, P. 2012. Machine learning: the art and science of
algorithms that make sense of data. Cambridge
University Press.
Frank, E., Hall, M. A. & Witten, I. H. 2016. The WEKA
workbench. Data mining: Practical machine learning
tools and techniques, 4.
Gope, J. & Jain, S. K. 2017. A Survey on Solving Cold Start
Problem in Recommender Systems.
Harper, F. M. & Konstan, J. A. 2016. The movielens
datasets: History and context. Acm transactions on
interactive intelligent systems (tiis), 5, 19.
Isinkaye, F. O., Folajimi, Y. O. & Ojokoh, B. A. 2015.
Recommendation systems: Principles, methods and
evaluation. Egyptian Informatics Journal, 16, 261-273.
Karimi, R., Freudenthaler, C., Nanopoulos, A. & Schmidt-
Thieme, L. 2011. Towards Optimal Active Learning for
Matrix Factorization in Recommender Systems. 2011
23rd IEEE International Conference on Tools with
Artificial Intelligence (ICTAI). IEEE.
Karimi, R., Freudenthaler, C., Nanopoulos, A. & Schmidt-
Thieme, L. 2015. Comparing Prediction Models for
Active Learning in Recommender Systems.
Comparing Prediction Models for Active Learning in
Recommender Systems, 2015. 171-180.
Katarya, R. & Verma, O. P. 2016. A collaborative
recommender system enhanced with particle swarm
optimization technique. Multimedia Tools and
Applications, 75, 9225-9239.
Kim, H. M., Ghiasi, B., Spear, M., Laskowski, M. & Li, J.
2017. Online serendipity: The case for curated
recommender systems. Business Horizons, 60, 613-
620.
Kotkov, D., Konstan, J. A., Zhao, Q. & Veijalainen, J. 2018.
Investigating serendipity in recommender systems
based on real user feedback. Proceedings of the 33rd
Annual ACM Symposium on Applied Computing,
2018. ACM, 1341-1350.
Liao, C.-L. & Lee, S.-J. 2016. A clustering based approach
to improving the efficiency of collaborative filtering
recommendation. Electronic Commerce Research and
Applications, 18, 1-9.
Lu, J., Wu, D. S., Mao, M. S., Wang, W. & Zhang, G. Q.
2015. Recommender system application developments:
A survey. Decision Support Systems, 74, 12-32.
Lucas, J. P., Segrera, S. & Moreno, M. N. 2012. Making
use of associative classifiers in order to alleviate typical
drawbacks in recommender systems. Expert Systems
with Applications, 39, 1273-1283.
Minkov, E., Charrow, B., Ledlie, J., Teller, S. & Jaakkola,
T. 2010. Collaborative future event recommendation.
Proceedings of the 19th ACM international conference
on Information and knowledge management, 2010.
ACM, 819-828.
Mishra, R., Kumar, P. & Bhasker, B. 2015. A web
recommendation system considering sequential
information. Decision Support Systems, 75, 1-10.
Ntoutsi, E., Stefanidis, K., Rausch, K. & Kriegel, H. P.
2014. Strength lies in differences: Diversifying friends
for recommendations through subspace clustering.
In Proceedings of the 23rd ACM International
Conference on Conference on Information and
Knowledge Management, 2014. ACM, 729-738.
Pereira, A. L. V. & Hruschka, E. R. 2015. Simultaneous co-
clustering and learning to address the cold start problem
in recommender systems. Knowledge-Based Systems,
82, 11-19.
Pujari, A. K., Rajesh, K. & Reddy, D. S. 2001. Clustering
techniques in data mining-A survey. IETE Journal of
Research, 47, 19-28.
Saha, T., Rangwala, H. & Domeniconi, C. 2015. Predicting
preference tags to improve item recommendation.
Proceedings of the 2015 SIAM International
Conference on Data Mining, 2015. SIAM, 864-872.
Soundarya, V., Kanimozhi, U. & Manjula, D. 2017.
Recommendation System for Criminal Behavioral
Analysis on Social Network using Genetic Weighted K-
Means Clustering. JCP, 12, 212-220.
Sridevi, M., Rao, R. R. & Rao, M. V. 2016. A survey on
recommender system. International Journal of
Computer Science and Information Security, 14, 265.
Sun, S. 2007. An improved random subspace method and
its application to EEG signal classification.
International Workshop on Multiple Classifier
Systems, 2007. Springer, 103-112.
Tsai, C.-H. 2016. A fuzzy-based personalized
recommender system for local businesses. Proceedings
of the 27th ACM Conference on Hypertext and Social
Media, 2016. ACM, 297-302.
Wang, X., Hoi, S. C. H., Liu, C. & Ester, M. 2017.
Interactive social recommendation. Information and
Knowledge Management 2017. ACM, 357-366.
Witten, I. H., Frank, E., Hall, M. A. & Pal, C. J. 2016. Data
Mining: Practical machine learning tools and
techniques, Morgan Kaufmann.
Zhang, S., Yao, L., Sun, A. & Tay, Y. 2019. Deep learning
based recommender system: A survey and new
perspectives. ACM Computing Surveys (CSUR), 52, 5.
Active Learning and User Segmentation for the Cold-start Problem in Recommendation Systems
123