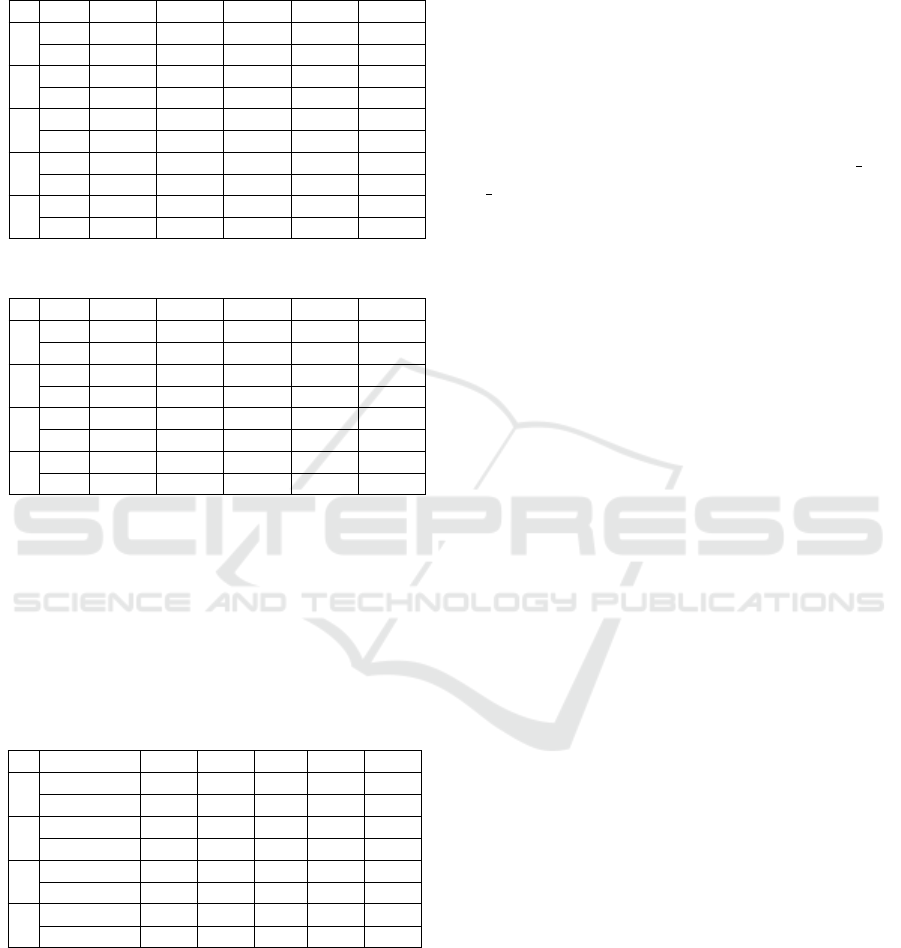
Ted}. Furthermore, we need to seek a diagnosis for
each patient p
i
, for i = 1, 2, 3, 4.
Table 2: Symptoms characteristic for the diagnoses.
Op VF Ma Ty SP CP
T
3T 1 (1,0) (1,0) (0.7,0.3) (0.3,0.7) (0.2,0.8)
2?T 1 (0.4,0.6) (0.5,0.5) (0.3,0.7) (0.1,0.9) (0.1,0.9)
H
3T 1 (0.5,0.5) (0.4,0.6) (0.9,0.1) (0.6,0.4) (0.2,0.8)
2?T 1 (0.3,0.7) (0.2,0.8) (0.5,0.5) (0.2,0.8) (0,1)
SPa
3T 1 (0.3,0.7) (0.1,0.9) (0.3,0.7) (1,0) (0.2,0.8)
2?T 1 (0.1,0.9) (0,1) (0.2,0.8) (0.5,0.5) (0.2,0.8)
C
3T 1 (0.7,0.3) (1,0) (0.4,0.6) (0.3,0.7) (0.2,0.8)
2?T 1 (0.4,0.6) (0.5,0.5) (0.2,0.8) (0.2,0.8) (0.2,0.8)
CPa
3T 1 (0.3,0.7) (0.2,0.8) (0.1,0.9) (0.3,0.7) (0.9,0.1)
2?T 1 (0.1,0.9) (0.1,0.9) (0.1,0.9) (0.2,0.8) (0.5,0.5)
Table 3: Symptoms characteristic for the patient.
Op T H SPa C CPa
Al
2T 2 (0.8,0.2) (0.6,0.4) (0.2,0.8) (0.6,0.4) (0.9,0.1)
3!T 2 (0.9,0.1) (0.9,0.1) (0.5,0.5) (0.9,0.1) (0.5,0.5)
Bob
2T 2 (0,1) (0.4,0.6) (0.6,0.4) (0.1,0.9) (0.1,0.9)
3!T 2 (0.5,0.5) (0.6,0.4) (0.9,0.1) (0.5,0.5) (0.5,0.5)
Joe
2T 2 (0.8,0.2) (0.8,0.2) (0,1) (0.2,0.8) (0,1)
3!T 2 (0.9,0.1) (0.9,0.1) (0.5,0.5) (0.5,0.5) (0.5,0.5)
Ted
2T 2 (0.6,0.4) (0.5,0.5) (0.3,0.7) (0.7,0.3) (0.3,0.7)
3!T 2 (0.9,0.1) (0.6,0.4) (0.6,0.4) (0.8,0.2) (0.6,0.4)
We calculate the A-CC in Eq. (30) between Ta-
bles 2 and 3 deriving a diagnosis for each patient
p
i
, for i = 1,2,3,4. In the first step, the method is
performed applying the A-CC between the operators
3T 1 and 2T 2. And, in the second one, the method
uses the operators 2?T 1 and 3!T 2 deriving the re-
lated A-CC. All the results for the considered patients
are listed in Table 4.
Table 4: Resulting A-CC of symptoms for each patient.
A-CC VF Ma Ty SP CP
Al
(3T 1, 2T 2) 0,610 0,568 0,538 -0,255 -0,452
(2?T 1, 3!T 2) 0,642 0,555 0,441 -0,441 -0,448
Bob
(3T 1, 2T 2) -0,430 -0,472 0,029 0,646 -0,208
(2?T 1, 3!T 2) -0,381 -0,460 0 0,634 -0,103
Joe
(3T 1, 2T 2) 0,455 0,326 0,632 -0,158 -0,327
(2?T 1, 3!T 2) 0,361 0,238 0,562 -0,361 -0,488
Ted
(3T 1, 2T 2) 0,553 0,632 0,344 -0,363 -0,375
(2?T 1, 3!T 2) 0,544 0,614 0 -0,389 -0,189
Based on the arguments in Table 4, for both meth-
ods a proper diagnosis coincides as follows: Al suf-
fers from Viral fever, Bob from a stomach problem,
Joe from Typhoid, and Ted from Malaria. Addition-
ally, one can observe that in (Xu, 2006)), the diagno-
sis is the same in two patients (Bob and Joe) and it
is reverse in other two (Al and ted). Despite the A-
CC expression used in (Xu, 2006, Definition 3.1)) is
able to preserve the property on which any two IFSs
equals one iff these two IFSs are the same, it does not
consider the action of the IFIx π providing the mea-
sure of hesitance degree. The distinct methodologies
justified the difference in the results.
8 CONCLUSION
In this paper, the analytical expressions of A-CC were
considered to pairs of modal α-level operators K
1
2
and
L
1
2
, in particular, for !A and ?A, also including their
conjugate operators. Moreover, we present an appli-
cation of the A-CC with the modal operators of ne-
cessity, possibility, and related modal-level operators
?A and !A.
Further work intends to extend these studies of A-
IFSs to other fuzzy connectives frequently applied to
making decision based on fuzzy systems.
ACKNOWLEDGEMENTS
This work was supported by CAPES/Brasil - Finance
Code 001 and PqG-FAPERGS (17/2551-0001207-0)
and Pq-CNPq (310106/2016-8).
REFERENCES
Atanassov, K. (1983). Intuitionistic fuzzy sets. vii itkr ses-
sion. sofia. Centr. Sci.-Techn. Library of Bulg. Acad.
of Sci., 1697(84).
Atanassov, K. and Gargov, G. (1989). Interval valued intu-
itionistic fuzzy sets. Fuzzy Sets Systems, 31(3):343–
349.
Atanassov, K. T. (1986). Intuitionistic fuzzy sets. Fuzzy
Sets and Systems, pages 87–96.
Atanassov, K. T. (1999). Intuitionistic Fuzzy Sets: Theory
and Applications. Studies in Fuzziness and Soft Com-
puting. Physica-Verlag HD, Heidelberg, Germany,
Germany.
Bertei, A. and Reiser, R. (2018). Correlation coefficient
analysis performed on duality and conjugate modal-
level operators. In 2018 IEEE Intl. Conf. on Fuzzy
Systems, pages 1–8.
Bertei, A., Zanotelli, R., Cardoso, W., Reiser, R., Foss, L.,
and Bedregal, B. (2016). Correlation coefficient anal-
ysis based on fuzzy negations and representable auto-
morphisms. In 2016 IEEE Intl. Conf. on Fuzzy Sys-
tems, pages 127–132.
Bustince, H., Barrenechea, E., and Mohedano, V. (2004).
Intuicionistic fuzzy implication operators - an expres-
sion and main properties. Uncertainty, Fuzziness and
Knowledge-Based Systems, 12:387–406.
FCTA 2019 - 11th International Conference on Fuzzy Computation Theory and Applications
286