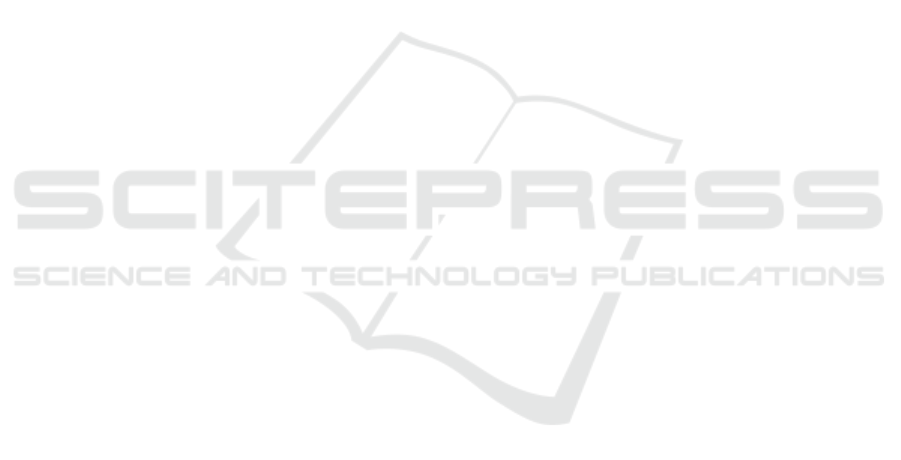
will be proposed to improve the recommendation for
a cold-start scenario.
ACKNOWLEDGEMENTS
The authors are grateful for the support given
by S
˜
ao Paulo Research Foundation (FAPESP).
Grant #2014/04851-8, and the support given by
Ita
´
u Unibanco S.A. trough the Ita
´
u Scholarship
Program, at the Centro de Ci
ˆ
encia de Dados (C
2
D),
Universidade de S
˜
ao Paulo, Brazil.
REFERENCES
Al-Hassan, M., Lu, H., and Lu, J. (2015). A
semantic enhanced hybrid recommendation approach:
A case study of e-Government tourism service
recommendation system. Decis. Support Syst.,
72:97–109.
Contratres, F. G., Alves-Souza, S. N., Filgueira, L.
V. L., and DeSouza, L. S. (2018). Sentiment
analysis of social network data for cold-start relief
in recommender systems. In New Contributions in
Information Systems and Technologies. Advances in
Intelligent Systems and Computing. in press.
Deng, S., Huang, L., and Xu, G. (2014). Social
network-based service recommendation with trust
enhancement. Expert Systems with Applications,
41(18):8075–8084.
Desrosiers, C. and Karypis, G. (2011). A Comprehensive
Survey of Neighborhood-based Recommendation
Methods, chapter 4. Springer US.
Gonzalez-Camacho, L. A. and Alves-Souza, S. N.
(2018). Social network data to alleviate
cold-start in recommender system: A systematic
review. Information Processing & Management,
54(4):529–544.
Guo, J., Zhu, Y., Li, A., Wang, Q., and Han, W. (2016). A
Social Influence Approach for Group User Modeling
in Group Recommendation Systems. IEEE Intelligent
Systems, 31(5):40–48.
Hu, Y., Volinsky, C., and Koren, Y. (2008). Collaborative
filtering for implicit feedback datasets. Proceedings
- IEEE International Conference on Data Mining,
ICDM.
Koren, Y. and Bell, R. (2011). Advances in Collaborative
Filtering, chapter 5. Springer US.
Koren, Y., Bell, R., and Volinsky, C. (2009). Matrix
factorization techniques for recommender systems.
Computer, 42(8):30–37.
Krejcie, R. V. and Morgan, D. W. (1970). Determining
Sample Size for Research Activities . Educational and
Psychological Measurement, 30(3).
Lalwani, D., Somayajulu, D. V. L. N., and Krishna, P. R.
(2015). A community driven social recommendation
system. Proc. - 2015 IEEE Int. Conf. Big Data, IEEE
Big Data 2015, pages 821–826.
Lian, D., Ge, Y., Zhang, F., Yuan, N. J., Xie, X., Zhou,
T., and Rui, Y. (2016). Content-aware collaborative
filtering for location recommendation based on human
mobility data. Proc. - IEEE Int. Conf. Data Mining,
ICDM, 2016-Janua:261–270.
Masthoff, J. (2011). Group Recommender Systems:
Combining Individual Models, chapter 21. Springer
US.
Masthoff, J. and Gatt, A. (2006). In pursuit of satisfaction
and the prevention of embarrassment: affective state
in group recommender systems. User Modeling and
User-Adapted Interaction, 16(3-4):281–319.
Prando, A., Contratres, F., Alves-Souza, S., and deSouza,
L. (2017). Content-based recommender system using
social networks for cold-start users. In Proceedings of
the 9th International Joint Conference on Knowledge
Discovery,Knowledge Engineering and Knowledge
Management. in press.
Quirino, G. Z., Mals, N. P., Groterhorst, V. M., De Souza,
S. N., and De Souza, L. S. (2015). Meneduca
- Social school network to support the educational
environment. In Proceedings - 2015 41st Latin
American Computing Conference, CLEI 2015.
Resnick, P. and Varian, H. R. (1997). Recommender
systems. Communications of the ACM, 40(3):56–58.
Ricci, F., Rokach, L., and Shapira, B. (2011). Recommender
Systems Handbook. Springer US.
Wang, Z. and Lu, H. (2014). Online recommender
system based on social network regularization.
8834:487–494.
Zhang, Y., Chen, W., and Yin, Z. (2013). Collaborative
filtering with social regularization for TV program
recommendation. Knowledge-Based Syst.,
54:310–317.
Zhao, W. X., Li, S., He, Y., Chang, E. Y., Wen, J. R.,
and Li, X. (2016). Connecting Social Media to
E-Commerce: Cold-Start Product Recommendation
Using Microblogging Information. IEEE Trans.
Knowl. Data Eng., 28:1147–1159.
Zhu, H. and Huberman, B. A. (2014). To Switch or
Not To Switch: Understanding Social Influence in
Online Choices. American Behavioral Scientist,
58(10):1329–1344.
Social Tracks: Recommender System for Multiple Individuals using Social Influence
371