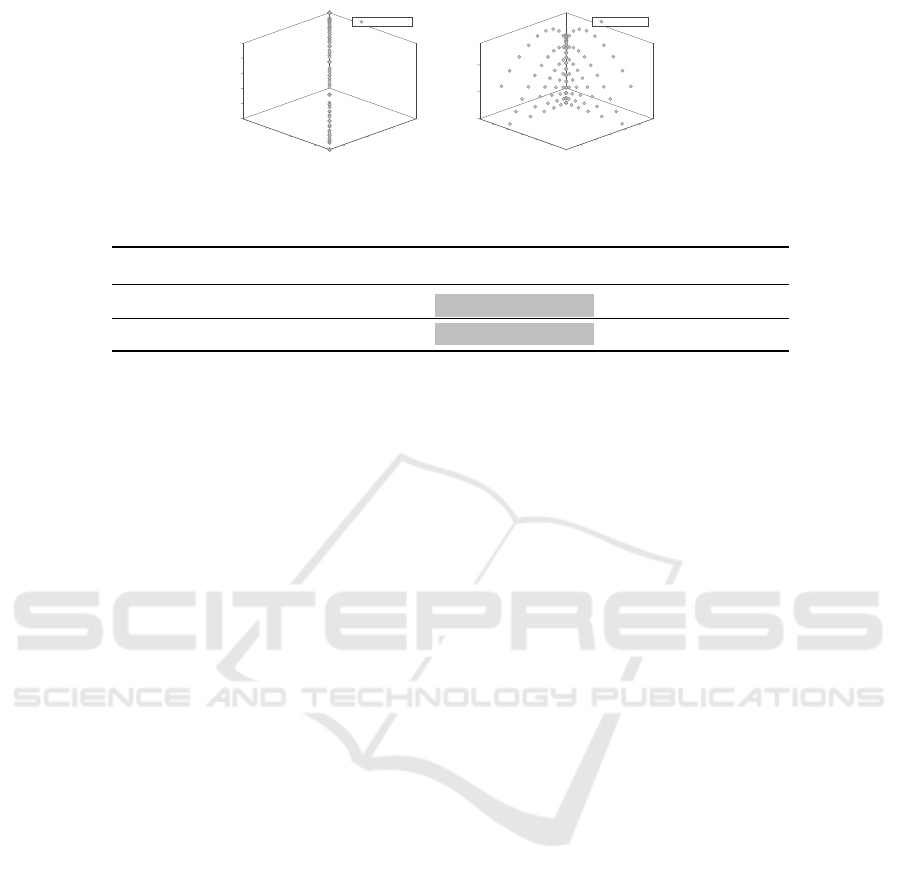
0
0.2
0.4
0.20.2
DTLZ5
f
3
0.6
f
1
f
2
0.8
0.40.4
1
0.60.6
MOEA/D-ABC
0
0.5
0.5
0.5
1
1
DTLZ5
f
3
f
1
f
2
1
1.5
1.5
2
2
2.5
2.5
MOEA/D-PBI
Figure 3: Obtained solutions by MOEA/D-ABC, and MOEA/D-PBI for DTLZ5.
Table 4: IGD values for MOEA/D-ABC, and MOEA/D-PBI on DTLZ5 and DTLZ6.
Problem N m D FEs MOEA/D-ABC MOEA/D-PBI
DTLZ5
200 3 12 100000 1.1250e-2 (2.06e-5) 2.2605e-2 (1.95e-5)
DTLZ6
200 3 12 100000 1.1319e-2 (7.43e-6) 2.2632e-2 (4.78e-6)
Deb, K. and Jain, H. (2014). An evolutionary many-
objective optimization algorithm using reference-
point-based nondominated sorting approach, part i:
solving problems with box constraints. IEEE Transac-
tions on Evolutionary Computation, 18(4):577–601.
Deb, K. and Kalyanmoy, D. (2001). Multi-Objective Opti-
mization Using Evolutionary Algorithms. John Wiley
& Sons, Inc., New York, NY, USA.
Deb, K., Pratap, A., Agarwal, S., and Meyarivan, T. (2002).
A fast and elitist multiobjective genetic algorithm:
Nsga-ii. IEEE Transactions on Evolutionary Compu-
tation, 6(2):182–197.
Deb, K., Thiele, L., Laumanns, M., and Zitzler, E.
(2001). Scalable test problems for evolutionary multi-
objective optimization. Technical report, Computer
Engineering and Networks Laboratory (TIK), Swiss
Federal Institute of Technology (ETH.
Karaboga, D. (2005). An idea based on honey bee swarm
for numerical optimization.
Ke, L., Zhang, Q., and Battiti, R. (2013). Moea/d-aco: A
multiobjective evolutionary algorithm using decom-
position and antcolony. IEEE transactions on cyber-
netics, 43(6):1845–1859.
Li, H. and Landa-Silva, D. (2011). An adaptive evolution-
ary multi-objective approach based on simulated an-
nealing. Evolutionary Computation, 19(4):561–595.
Li, H. and Zhang, Q. (2009). Multiobjective optimization
problems with complicated pareto sets, moea/d and
nsga-ii. IEEE Transactions on Evolutionary Compu-
tation, 13(2):284–302.
Qi, Y., Ma, X., Liu, F., Jiao, L., Sun, J., and Wu, J. (2014).
Moea/d with adaptive weight adjustment. Evolution-
ary computation, 22(2):231–264.
Reyes-Sierra, M., Coello, C. C., et al. (2006). Multi-
objective particle swarm optimizers: A survey of the
state-of-the-art. International journal of computa-
tional intelligence research, 2(3):287–308.
Sato, H. (2015). Analysis of inverted pbi and compari-
son with other scalarizing functions in decomposition
based moeas. Journal of Heuristics, 21(6):819–849.
Tian, Y., Cheng, R., Zhang, X., and Jin, Y. (2017). Platemo:
A matlab platform for evolutionary multi-objective
optimization [educational forum]. IEEE Computa-
tional Intelligence Magazine, 12:73–87.
Trivedi, A., Srinivasan, D., Sanyal, K., and Ghosh, A.
(2017). A survey of multiobjective evolutionary al-
gorithms based on decomposition. IEEE Transactions
on Evolutionary Computation, 21(3):440–462.
Veldhuizen, D. A. V. and Lamont, G. B. (1998). Multiob-
jective evolutionary algorithm research: A history and
analysis.
Yuan, Y., Xu, H., and Wang, B. (2014). An improved nsga-
iii procedure for evolutionary many-objective opti-
mization. In Proceedings of the 2014 Annual Con-
ference on Genetic and Evolutionary Computation,
GECCO ’14, pages 661–668, New York, NY, USA.
ACM.
Yuan, Y., Xu, H., Wang, B., Zhang, B., and Yao,
X. (2015). Balancing convergence and diversity
in decomposition-based many-objective optimizers.
IEEE Transactions on Evolutionary Computation,
20(2):180–198.
Zhang, Q. and Li, H. (2007). Moea/d: A multiob-
jective evolutionary algorithm based on decomposi-
tion. IEEE Transactions on evolutionary computation,
11(6):712–731.
Zhang, Q., Zhu, W., Liao, B., Chen, X., and Cai, L. (2018).
A modified pbi approach for multi-objective optimiza-
tion with complex pareto fronts. Swarm and Evolu-
tionary Computation, 40:216–237.
Zitzler, E., Deb, K., and Thiele, L. (2000). Comparison
of multiobjective evolutionary algorithms: Empirical
results. Evol. Comput., 8(2):173–195.
A Multiobjective Artificial Bee Colony Algorithm based on Decomposition
195