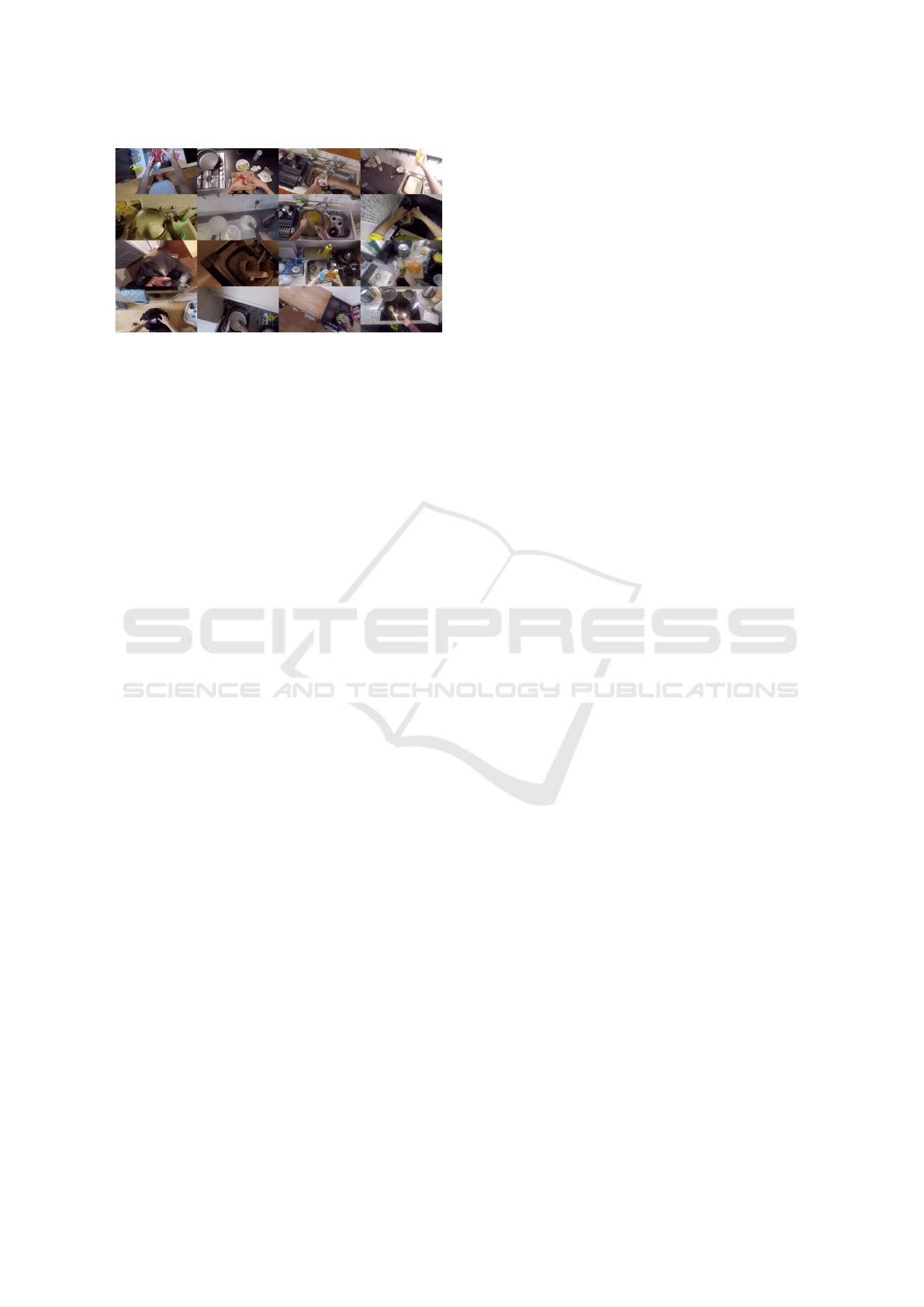
Figure 1: Sample frames from EPIC-Kitchens.
blems in fine-grained object interactions. A few of
these opportunities are highlighted here.
• Overlapping Object Interactions: Defining the
temporal extent of an action is fundamentally an
ambiguous problem (Moltisanti et al., 2017; Si-
gurdsson et al., 2017). This is usually resolved
through multi-labels, i.e. allowing a time-segment
to belong to multiple classes of actions. Howe-
ver, actual understanding of interaction overlap-
ping requires an space of action labels that cap-
tures dependencies (e.g. filling a kettle requires
opening the tap). Models that capture and predict
overlapping interactions are needed for a finer-
understanding of object interactions.
• Object Interaction Completion/Incompletion:
Beyond classification and localisation, action
completion/incompletion is the problem of
identifying whether the action’s goal has been
successfully achieved, or merely attempted. This
is a novel fine-grained object interaction research
question proposed in (Heidarivincheh et al.,
2016). This work has been recently extended to
locating the moment of completion (Heidarivin-
cheh et al., 2018) - that is the moment in time
beyond which the action’s goal is believed to be
completed by a human observer.
• Skill Determination from Video: Even when an
interaction is successfully completed, further un-
derstanding of ‘how well’ the task was comple-
ted would offer knowledge beyond pure classifica-
tion. In this leading work (Doughty et al., 2018a),
a collection of video could be ordered by the skill
exhibited in each video, through deep pairwise
ranking. This method has been recently extended
to include rank-aware attention (Doughty et al.,
2018b) - that is a novel loss function capable of
attending to parts of the video that exhibit higher
skill as well as parts that demonstrate lower skill
including mistakes or hesitation.
• Anticipation and Forecasting: Predicting upco-
ming interactions has recently gathered additional
attention, triggered by the presence of first-person
datasets (Furnari et al., 2018; Rhinehart and Ki-
tani, 2017). Novel research on uncertainty in anti-
cipating actions (Furnari et al., 2018), or relating
forecasting to trajectory prediction (Rhinehart and
Kitani, 2017) have recently been proposed.
• Paired Interactions: One leading work has at-
tempted capturing both the action and its counter-
action (or reaction), both from a wearable ca-
mera (Yonetani et al., 2016). This is a very ex-
citing area of research, still under-explored.
5 CONCLUSION
Recent deep-learning research has only scratched the
surface of potentials for finer-grained understanding
of object interactions. As new hardware platforms
for first-person vision emerge (Microsoft’s Hololens,
Magic Leap, Samsung Gear, · · · ), applications of fine-
grained recognition will be endless.
REFERENCES
Alletto, S., Serra, G., Calderara, S., and Cucchiara, R.
(2015). Understanding social relationships in egocen-
tric vision. In Pattern Recognition.
Caba Heilbron, F., Escorcia, V., Ghanem, B., and Car-
los Niebles, J. (2015). Activitynet: A large-scale vi-
deo benchmark for human activity understanding. In
CVPR.
Carreira, J. and Zisserman, A. (2017). Quo vadis, action
recognition? a new model and the kinetics dataset. In
CVPR.
Damen, D., Doughty, H., Farinella, G. M., Fidler, S., Fur-
nari, A., Kazakos, E., Moltisanti, D., Munro, J., Per-
rett, T., Price, W., and Wray, M. (2018). Scaling
egocentric vision: The EPIC-KITCHENS Dataset. In
ECCV.
Damen, D., Leelasawassuk, T., Haines, O., Calway, A., and
Mayol-Cuevas, W. (2014). You-do, I-learn: Disco-
vering task relevant objects and their modes of inte-
raction from multi-user egocentric video. In BMVC.
De La Torre, F., Hodgins, J., Bargteil, A., Martin, X., Ma-
cey, J., Collado, A., and Beltran, P. (2008). Guide to
the Carnegie Mellon University Multimodal Activity
(CMU-MMAC) database. In Robotics Institute.
Doughty, H., Damen, D., and Mayol-Cuevas, W. (2018a).
Who’s Better? Who’s Best? Pairwise Deep Ranking
for Skill Determination. In CVPR.
Doughty, H., Mayol-Cuevas, W., and Damen, D. (2018b).
The Pros and Cons: Rank-aware temporal attention
for skill determination in long videos. In Arxiv.
Fathi, A., Hodgins, J., and Rehg, J. (2012a). Social inte-
ractions: A first-person perspective. In CVPR.
VISIGRAPP 2019 - 14th International Joint Conference on Computer Vision, Imaging and Computer Graphics Theory and Applications
12