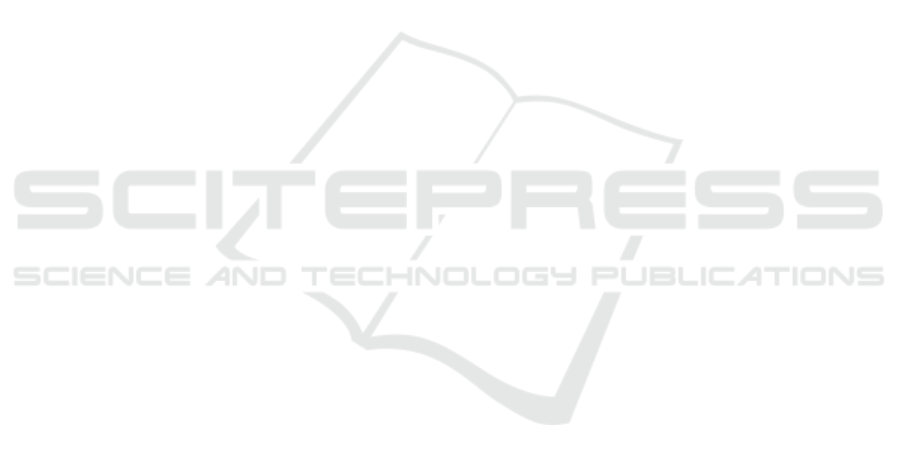
approaches for managing driving context in intelligent
transportation. In KEOD, pages 302–309.
Huet, R., Courty, N., and Lef
`
evre, S. (2016). A new penal-
isation term for image retrieval in clique neural net-
works. In European Symposium on Artificial Neural
Networks, Computational Intelligence and Machine
Learning (ESANN).
Huynh, T., Gao, Y., Kang, J., Wang, L., Zhang, P., Lian,
J., and Shen, D. (2016). Estimating ct image from
mri data using structured random forest and auto-
context model. IEEE transactions on medical imag-
ing, 35(1):174.
Japkowicz, N., Hanson, S. J., and Gluck, M. A. (2000).
Nonlinear autoassociation is not equivalent to pca.
Neural computation, 12(3):531–545.
Jia, F., Lei, Y., Lin, J., Zhou, X., and Lu, N. (2016). Deep
neural networks: A promising tool for fault character-
istic mining and intelligent diagnosis of rotating ma-
chinery with massive data. Mechanical Systems and
Signal Processing, 72:303–315.
Jiang, G., Xie, P., He, H., and Yan, J. (2018). Wind turbine
fault detection using a denoising autoencoder with
temporal information. IEEE/ASME Transactions on
Mechatronics, 23(1):89–100.
Jing, L., Wang, T., Zhao, M., and Wang, P. (2017). An adap-
tive multi-sensor data fusion method based on deep
convolutional neural networks for fault diagnosis of
planetary gearbox. Sensors, 17(2):414.
Lee, C.-C. (2014). Gender classification using m-estimator
based radial basis function neural network. In Signal
Processing and Multimedia Applications (SIGMAP),
2014 International Conference on, pages 302–306.
IEEE.
Liu, Z., Tang, B., He, X., Qiu, Q., and Liu, F. (2017).
Class-specific random forest with cross-correlation
constraints for spectral–spatial hyperspectral image
classification. IEEE Geoscience and Remote Sensing
Letters, 14(2):257–261.
Madani, P. and Vlajic, N. (2018). Robustness of deep
autoencoder in intrusion detection under adversarial
contamination. In Proceedings of the 5th Annual Sym-
posium and Bootcamp on Hot Topics in the Science of
Security, page 1. ACM.
Mart
´
ınez-Rego, D., Fontenla-Romero, O., and Alonso-
Betanzos, A. (2011). Power wind mill fault detection
via one-class ν-svm vibration signal analysis. In Neu-
ral Networks (IJCNN), The 2011 International Joint
Conference on, pages 511–518. IEEE.
Mishra, K. M. and Huhtala, K. (2019). Fault detection of el-
evator systems using multilayer perceptron neural net-
work. In 24th IEEE Conference on Emerging Tech-
nologies and Factory Automation (ETFA). IEEE.
Mohamed, R., El-Makky, N. M., and Nagi, K. (2017). Hy-
bqa: Hybrid deep relation extraction for question an-
swering on freebase. In KEOD, pages 128–136.
Seppala, J., Koivisto, H., and Koivo, H. (1998). Modeling
elevator dynamics using neural networks. In Neural
Networks Proceedings, 1998. IEEE World Congress
on Computational Intelligence. The 1998 IEEE Inter-
national Joint Conference on, volume 3, pages 2419–
2424. IEEE.
Sun, W., Shao, S., Zhao, R., Yan, R., Zhang, X., and Chen,
X. (2016). A sparse auto-encoder-based deep neural
network approach for induction motor faults classifi-
cation. Measurement, 89:171–178.
Vincent, P., Larochelle, H., Bengio, Y., and Manzagol, P.-
A. (2008). Extracting and composing robust features
with denoising autoencoders. In Proceedings of the
25th international conference on Machine learning,
pages 1096–1103. ACM.
Wang, P., He, W., and Yan, W. (2009). Fault diagnosis of
elevator braking system based on wavelet packet algo-
rithm and fuzzy neural network. In Electronic Mea-
surement & Instruments, 2009. ICEMI’09. 9th Inter-
national Conference on, pages 4–1028. IEEE.
Xia, M., Li, T., Xu, L., Liu, L., and de Silva, C. W.
(2018). Fault diagnosis for rotating machinery us-
ing multiple sensors and convolutional neural net-
works. IEEE/ASME Transactions on Mechatronics,
23(1):101–110.
Yang, Z.-X. and Zhang, P.-B. (2016). Elm meets rae-elm:
A hybrid intelligent model for multiple fault diagnosis
and remaining useful life predication of rotating ma-
chinery. In Neural Networks (IJCNN), 2016 Interna-
tional Joint Conference on, pages 2321–2328. IEEE.
Zhang, R., Peng, Z., Wu, L., Yao, B., and Guan, Y. (2017).
Fault diagnosis from raw sensor data using deep neu-
ral networks considering temporal coherence. Sen-
sors, 17(3):549.
KEOD 2019 - 11th International Conference on Knowledge Engineering and Ontology Development
342