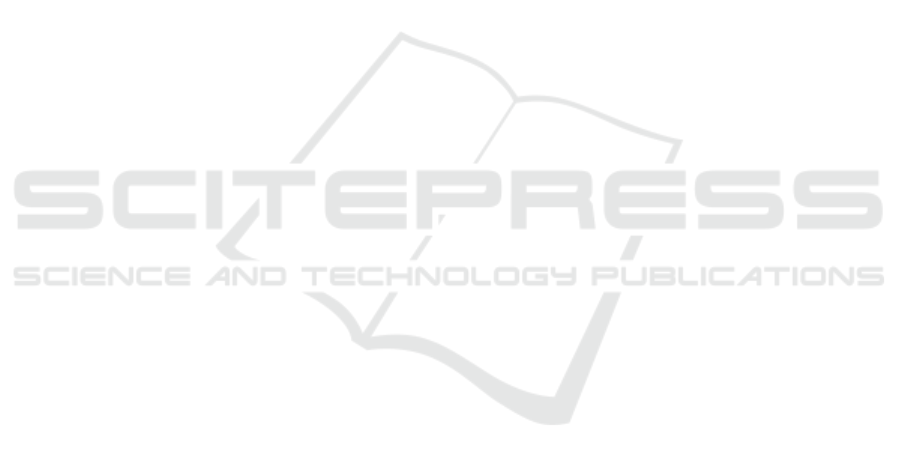
learn scaled and communicate with humans in natu-
ral language (Kelly, 2015) in the Knowledge Stairway
4.0. “Augmented Intelligence” refers to the extension
of the competence of experts by a system (Davenport
and Kirby, 2016), “Cognitive Computing” to the in-
tegration of knowledge from different sources (North
and Maier, 2018). Assist 4.0 is the leader in network-
ing machines; the other models do not refer to this
criterion.
Seidenst
¨
ucker states with regard to data pro-
tection that a maximum of data security must be
achieved (Seidenst
¨
ucker, 2017). The Assist 4.0 con-
cept is the only model that presents possibilities for
full data protection. The other models do not consider
data protection.
5 CONCLUSION
The present work contributes to the overall question
of how well current and well-established knowledge
management models are able to handle the new chal-
lenges and requirements introduced by Industry 4.0
and the ongoing digital transition in the industrial sec-
tor. It does that by analyzing two main research ques-
tions (see Section 1).
The question regarding the newly induced chal-
lenges (cf. RQ1) has been answered through a liter-
ature study that revealed that in addition to the al-
ready well-established knowledge processing tasks,
smart factories introduce new aspects such as human-
machine-, machine-machine-communication as well
as data protection and privacy.
The extent to which those requirements are sup-
ported by current knowledge management models
has been analyzed in the second part of this work
(cf. RQ2). The analysis revealed that the aspects of
human-machine-, machine-machine-communication
as well as data protection and privacy are only selec-
tively supported by current knowledge management
models and initiatives.
These results also corroborate the fact that in In-
dustry 4.0, humans and machines (both hardware and
software) need to be considered as equitable partners
that both participate in the knowledge creation and
processing life-cycle. This perspective needs to be
taken into consideration by future knowledge man-
agement models and it must be complemented by ini-
tiatives to establish an organization-wide continuous
learning culture. Future works might built upon the
validation of those findings and propose models that
integrate the previously mentioned aspects in more
Industry 4.0 compliant ways.
REFERENCES
Aamodt, A. and Nyg
˚
ard, M. (1995). Different Roles
and Mutual Dependencies of Data, Information, and
Knowledge-An AI Perspective on their Integration.
Data Knowl. Eng., 16(3):191–222.
Brandl, P., Aschbacher, H., and H
¨
osch, S. (2015). Mobiles
Wissensmanagement in der Industrie 4.0. In Mensch
und Computer 2015 - Workshopband, Stuttgart, Ger-
many, September 6-9, 2015, pages 225–232.
C Feng, S., Bernstein, W., Hedberg, T., and Barnard Feeney,
A. (2017). Toward knowledge management for smart
manufacturing. Journal of Computing and Informa-
tion Science in Engineering, 17.
Davenport, T. H. and Kirby, J. (2016). Only humans need
apply: winners and losers in the age of smart ma-
chines. Harper Business New York, NY.
European Commission, editor (2013). Factories of the Fu-
ture: Multi-annual Roadmap for the contractual PPP
under Horizon 2020. Publications Office of the Euro-
pean Union, Luxembourg.
Frey-Luxemburger, M. (2014). Ans
¨
atze und Modelle
des Wissensmanagements, pages 39–70. Springer
Fachmedien Wiesbaden, Wiesbaden.
Karagiannis, D. (2003). Wissensmanagement: Einige
konzepte & technologien. In Informationswirtschaft:
Ein Sektor mit Zukunft, pages 191–206.
Kelly, J. E. (2015). Computing, cognition and the future of
knowing. Whitepaper, IBM Reseach, page 2.
Kim, D.-W. and Yoon, S. W. (2018). Special issue on smart
automation and manufacturing. International Journal
Computer Integrated Manufacturing, 31(8):675–676.
Lechler, A. and Schlechtendahl, J. (2016). Steuerung aus
der Cloud. Handbuch Industrie 4.0: Produktion, Au-
tomatisierung und Logistik, pages 1–14.
Lehner, F. (2003). Information Sharing und Wissensaus-
tausch in Unternehmen. In Geyer-Schulz, A. and
Taudes, A., editors, Informationswirtschaft: Ein Sek-
tor mit Zukunft, volume 33 of LNI, pages 301–319.
GI.
North, K. (2016). Die Wissenstreppe, pages 33–65.
Springer Fachmedien Wiesbaden, Wiesbaden.
North, K. and Maier, R. (2018). Wissen 4.0 – Wissens-
management im digitalen Wandel. HMD Praxis der
Wirtschaftsinformatik, 55(4):665–681.
North, K., Reinhardt, K., and Sieber-Suter, B. (2018). Was
ist Kompetenz?, pages 35–110. Springer Fachmedien
Wiesbaden, Wiesbaden.
Probst, G. J. B. and Romhardt, K. (1997). Bausteine
des Wissensmanagements — ein praxisorientierter
Ansatz, pages 129–143. Gabler Verlag, Wiesbaden.
Reinmann-Rothmeier, G. (2001). Wissen managen: Das
M
¨
unchener Modell. Forschungsbericht Nr. 131 –
LMU M
¨
unchen: Lehrstuhl f
¨
ur Empirische P
¨
adagogik
und P
¨
adagogische Psychologie.
Rowley, J. (2007). The wisdom hierarchy: representations
of the DIKW hierarchy. Journal of information sci-
ence, 33(2):163–180.
Knowledge Management Models for the Smart Factory: A Comparative Analysis of Current Approaches
403