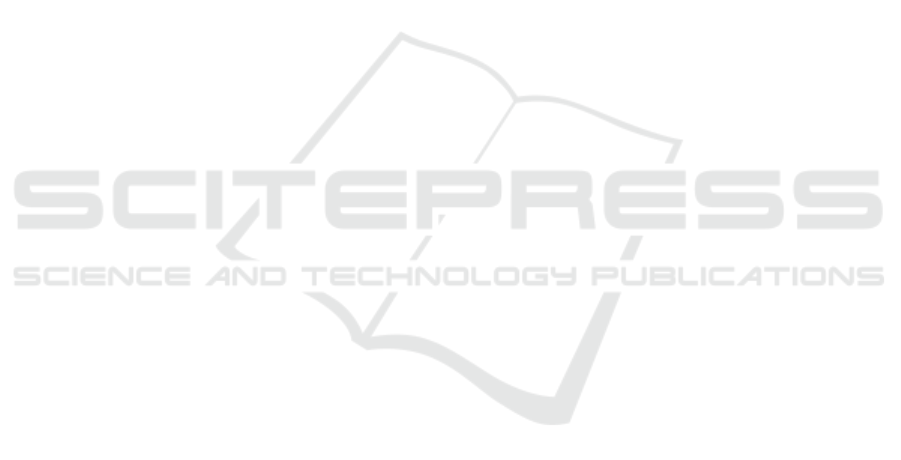
dence than the correct one is found, at least the trust of
this new knowledge base is higher than for the start-
ing point. Especially for an intermediate trust value
of around 60% to 75%, the improvement in trust is
noticeable. Probably the improvement is also better
for higher trust values p, if a better algorithm for the
optimization problem is used. An additional obser-
vation is, that the stability of values for large knowl-
edge bases is higher as compared to smaller knowl-
edge bases, i.e. the experimental results suffer from
high volatility for small datasets.
6 CONCLUSION
The paper presented a novel approach to deal with
probabilistic description logics. By not interpreting
each axiom separately, but a whole knowledge base
together, it enables results for cases, where other ap-
proaches would consider the knowledge base as in-
consistent. Also the computation time can be highly
reduced as compared to probabilistic description log-
ics by using optimization algorithms, which should be
subject to further research.
The disadvantage of the presented approach is,
that axioms can not be treated independently, i.e. the
whole knowledge base must be kept together. An-
other problem is that the probabilities of all axioms
are assumed to be independent of each other, i.e. there
is no space for redundancy. Nevertheless, the as-
sumption of having only one correct world instead
of assuming the existence of several possible worlds
seems more appropriate and realistic in many appli-
cation scenarios. Therefore a further development of
the presented idea seems useful.
For the evaluation, another interesting aspect
might be to consider also T-Box axioms to be uncer-
tain. Therefore another strategy for evaluation should
be developed, e.g. a different or separate treatment of
A-Box and T-Box axioms (Van Asch, 2013). For this
purpose, it might also be useful to change or param-
eterize formula (1) to determine the confidence of an
interpretation for a given knowledge base in a differ-
ent way.
ACKNOWLEDGEMENTS
We would like to thank the Deutsche Forschungs-
gemeinschaft (DFG) and the agence nationale de la
recherche (ANR) for the support of the TEXTELSEM
project, in which this work has been done.
REFERENCES
Baader, F., Calvanese, D., McGuinness, D., Patel-
Schneider, P., and Nardi, D. (2003). The description
logic handbook: Theory, implementation and applica-
tions. Cambridge university press.
Baader, F., Horrocks, I., and Sattler, U. (2005). Description
logics as ontology languages for the semantic web.
In Mechanizing mathematical reasoning, pages 228–
248. Springer.
Carvalho, R. N., Laskey, K. B., and Costa, P. C. (2017).
Pr-owl–a language for defining probabilistic ontolo-
gies. International Journal of Approximate Reason-
ing, 91:56–79.
Ding, Z., Peng, Y., and Pan, R. (2006). Bayesowl: Uncer-
tainty modeling in semantic web ontologies. In Soft
computing in ontologies and semantic web, pages 3–
29. Springer.
Dubois, D. and Prade, H. (2001). Possibility theory, prob-
ability theory and multiple-valued logics: A clarifi-
cation. Annals of mathematics and Artificial Intelli-
gence, 32(1-4):35–66.
Giugno, R. and Lukasiewicz, T. (2002). P-shoq (d): A prob-
abilistic extension of shoq (d) for probabilistic ontolo-
gies in the semantic web. In JELIA, volume 2, pages
86–97. Springer.
Klinov, P. (2008). Pronto: A non-monotonic probabilistic
description logic reasoner. In European Semantic Web
Conference, pages 822–826. Springer.
Koller, D., Levy, A., and Pfeffer, A. (1997). P-classic: A
tractable probablistic description logic. AAAI/IAAI,
1997(390-397):14.
Lukasiewicz, T. (2007). Probabilistic description logic pro-
grams. International Journal of Approximate Reason-
ing, 45(2):288–307.
Lukasiewicz, T. (2008). Expressive probabilistic descrip-
tion logics. Artificial Intelligence, 172(6-7):852–883.
Lukasiewicz, T. and Straccia, U. (2008). Managing uncer-
tainty and vagueness in description logics for the se-
mantic web. Web Semantics: Science, Services and
Agents on the World Wide Web, 6(4):291–308.
Niepert, M., Noessner, J., and Stuckenschmidt, H. (2011).
Log-linear description logics. In Twenty-Second Inter-
national Joint Conference on Artificial Intelligence.
Nilsson, N. J. (1986). Probabilistic logic. Artificial intelli-
gence, 28(1):71–87.
Riguzzi, F., Bellodi, E., Lamma, E., and Zese, R. (2015).
Probabilistic description logics under the distribution
semantics. Semantic Web, 6(5):477–501.
Van Asch, V. (2013). Macro-and micro-averaged evaluation
measures [[basic draft]]. Belgium: CLiPS, pages 1–
27.
Zolin, E. (2013). Complexity of reasoning in description
logics.
Axiom-based Probabilistic Description Logic
389