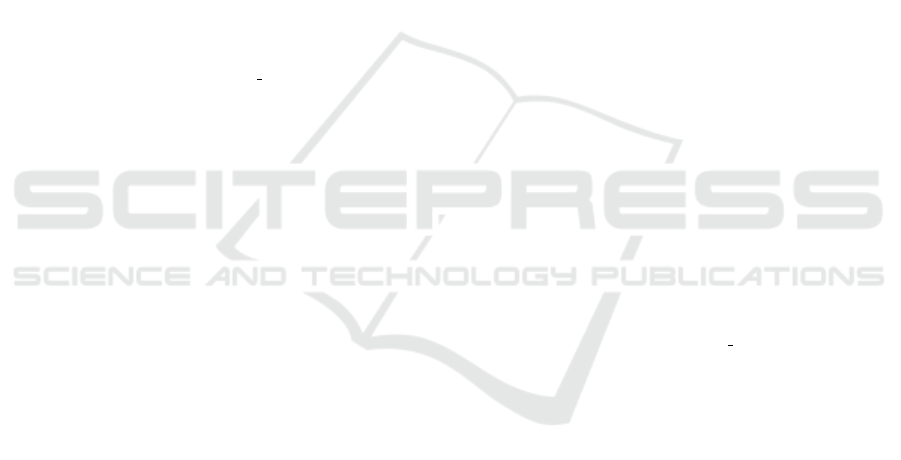
ACKNOWLEDGEMENT
This work was supported by the Slovenian Research
Agency Program P2-0098 and the European Union’s
Horizon 2020 research and innovation programme
[grant agreement No 769661].
REFERENCES
Alexander, M. and Anderson, J. (2012). The hansard cor-
pus, 1803-2003.
Beam, A. L., Kompa, B., Fried, I., Palmer, N. P., Shi, X.,
Cai, T., and Kohane, I. S. (2018). Clinical concept
embeddings learned from massive sources of medical
data. arXiv preprint arXiv:1804.01486.
Boag, W., Wacome, K., Naumann, T., and Rumshisky, A.
(2015). Cliner: A lightweight tool for clinical named
entity recognition. AMIA Joint Summits on Clinical
Research Informatics (poster).
Bodenreider, O. (2004). The unified medical language sys-
tem (umls): integrating biomedical terminology. Nu-
cleic acids research, 32(suppl 1):D267–D270.
Boulos, M. N. K., Yassine, A., Shirmohammadi, S., Nama-
hoot, C. S., and Br
¨
uckner, M. (2015). Towards an
“internet of food”: Food ontologies for the internet of
things. Future Internet, 7(4):372–392.
Caracciolo, C., Stellato, A., Rajbahndari, S., Morshed,
A., Johannsen, G., Jaques, Y., and Keizer, J. (2012).
Thesaurus maintenance, alignment and publication as
linked data: the agrovoc use case. International Jour-
nal of Metadata, Semantics and Ontologies, 7(1):65–
75.
C¸ elik, D. (2015). Foodwiki: Ontology-driven mobile safe
food consumption system. The Scientific World Jour-
nal, 2015.
Choi, E., Bahadori, M. T., Searles, E., Coffey, C., Thomp-
son, M., Bost, J., Tejedor-Sojo, J., and Sun, J. (2016).
Multi-layer representation learning for medical con-
cepts. In Proceedings of the 22nd ACM SIGKDD In-
ternational Conference on Knowledge Discovery and
Data Mining, pages 1495–1504. ACM.
Comeau, D. C., Do
˘
gan, R. I., Ciccarese, P., Cohen, K. B.,
Krallinger, M., Leitner, F., Lu, Z., Peng, Y., Rinaldi,
F., Torii, M., et al. (2013). Bioc: a minimalist ap-
proach to interoperability for biomedical text process-
ing. Database, 2013:bat064.
Donnelly, K. (2006). Snomed-ct: The advanced terminol-
ogy and coding system for ehealth. Studies in health
technology and informatics, 121:279.
(EFSA), E. F. S. A. (2015). The food classification and
description system foodex 2 (revision 2). EFSA Sup-
porting Publications, 12(5):804E.
Eftimov, T., Koro
ˇ
sec, P., and Korou
ˇ
si
´
c Seljak, B. (2017a).
Standfood: Standardization of foods using a semi-
automatic system for classifying and describing foods
according to FoodEx2. Nutrients, 9(6):542.
Eftimov, T., Korou
ˇ
si
´
c Seljak, B., and Koro
ˇ
sec, P. (2017b).
A rule-based named-entity recognition method for
knowledge extraction of evidence-based dietary rec-
ommendations. PloS One, 12(6):e0179488.
Gligic, L., Kormilitzin, A., Goldberg, P., and Nevado-
Holgado, A. (2019). Named entity recognition
in electronic health records using transfer learn-
ing bootstrapped neural networks. arXiv preprint
arXiv:1901.01592.
Griffiths, E. J., Dooley, D. M., Buttigieg, P. L., Hoehn-
dorf, R., Brinkman, F. S., and Hsiao, W. W. (2016).
Foodon: A global farm-to-fork food ontology. In
ICBO/BioCreative.
Groves, S. (2013). How allrecipes. com became the worlds
largest food/recipe site. roi of social media (blog).
Johnson, A. E., Pollard, T. J., Shen, L., Li-wei, H. L.,
Feng, M., Ghassemi, M., Moody, B., Szolovits, P.,
Celi, L. A., and Mark, R. G. (2016). Mimic-iii, a
freely accessible critical care database. Scientific data,
3:160035.
Jonquet, C., Shah, N., Youn, C., Callendar, C., Storey, M.-
A., and Musen, M. (2009). Ncbo annotator: seman-
tic annotation of biomedical data. In International
Semantic Web Conference, Poster and Demo session,
volume 110.
Kolchin, M. and Zamula, D. (2013). Food product ontol-
ogy: Initial implementation of a vocabulary for de-
scribing food products. In Proceeding of the 14th
Conference of Open Innovations Association FRUCT,
Helsinki, Finland, pages 11–15.
Miotto, R., Li, L., Kidd, B. A., and Dudley, J. T. (2016).
Deep patient: an unsupervised representation to pre-
dict the future of patients from the electronic health
records. Scientific reports, 6:26094.
Noy, N. F., Shah, N. H., Whetzel, P. L., Dai, B., Dorf, M.,
Griffith, N., Jonquet, C., Rubin, D. L., Storey, M.-A.,
Chute, C. G., et al. (2009). Bioportal: ontologies and
integrated data resources at the click of a mouse. Nu-
cleic acids research, 37(suppl 2):W170–W173.
Popovski, G., Kochev, S., Korou
ˇ
si
´
c Seljak, B., and Efti-
mov, T. (2019). Foodie: A rule-based named-entity
recognition method for food information extraction.
In Proceedings of the 8th International Conference
on Pattern Recognition Applications and Methods,
(ICPRAM 2019), pages 915–922.
Rayson, P., Archer, D., Piao, S., and McEnery, A. (2004).
The ucrel semantic analysis system.
Snae, C. and Br
¨
uckner, M. (2008). Foods: a food-oriented
ontology-driven system. In Digital Ecosystems and
Technologies, 2008. DEST 2008. 2nd IEEE Interna-
tional Conference on, pages 168–176. IEEE.
Wang, Q., Zhou, Y., Ruan, T., Gao, D., Xia, Y., and He,
P. (2019). Incorporating dictionaries into deep neu-
ral networks for the chinese clinical named entity
recognition. Journal of biomedical informatics, page
103133.
KEOD 2019 - 11th International Conference on Knowledge Engineering and Ontology Development
202