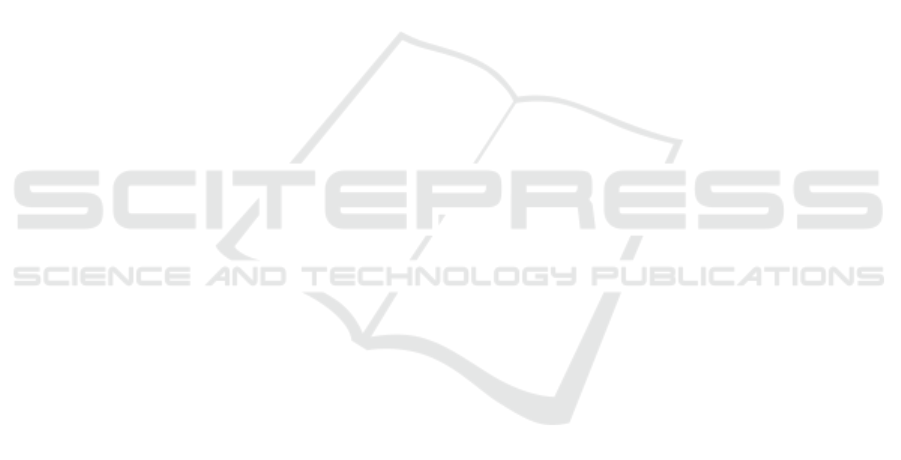
capabilities, whereas others, e.g., architecture,
construction, engineering and inspection services
have poor metrics. We believe that further research
should investigate the content of poor metric groups
and suggest possible solutions or find relevant content
within the tender documentation. We also noticed a
basic lack of data that could be used as dependent
target variables in this research area, which is why the
predictive power of the model is rather low. We
consider it entirely appropriate to consider open
public procurement procedures with one bid as cases
with great indication of corruption. A system based
on such models would be an excellent tool for experts
in the area of public procurement monitoring. For
example, due to the prevention of suspicious
activities at the very beginning of the tender
procedure, we can talk about an early warning
system. Further work should focus on including
additional indicators to enhance model accuracy as
well as applying neural networks, deep learning and
other machine-learning algorithms to achieve better
results.
REFERENCES
Ahmed, HA., Esraa, H., Abdul, A., 2017. Comparative
Study of Five Text Classification Algorithms with their
Improvements, International Journal of Applied
Engineering Research.
Azmi, KS., Rahman, AA., 2015. E-Procurement: A Tool to
Mitigate Public Procurement Fraud in Malaysia,
Electronic Journal of e-Government.
Bird, S., Klein, E., Loper, E., 2009. Natural language
processing with Python: analyzing text with the natural
language toolkit. O'Reilly Media, Inc.
Budak, J., 2016. Korupcija u javnoj nabavi: trebamo li novi
model istraživanja za Hrvatsku?. Ekonomski pregled.
Carvalho, RN., Sales, LJ., Da, Rocha, HA., Mendes, GL.,
2014. Using bayesian networks to identify and prevent
split purchases in Brazil, InProceedings of the Eleventh
UAI Conference on Bayesian Modeling Applications
Workshop.
Chapman, P., Clinton, J., Kerber, R., Khabaza, T., Reinartz,
T., Shearer, C., Wirth, R., 2016. CRISP-DM 1.0 Step-
by-step data mining guide, SPSS, CRISPMWP-1104.
Charron, N., Dahlström, C., Fazekas, M., Lapuente, V.,
2016. Careers, Connections, and Corruption Risks:
Investigating the Impact of Bureaucratic Meritocracy
on Public Procurement Processes. The Journal of
Politics.
Congcong, L., Jie, W., Lei, W., Luanyun, H., Peng, G.,
2014. Comparison of Classification Algorithms and
Training Sample Sizes in Urban Land Classification
with Landsat Thematic Mapper Imagery. Remote Sens.
Corruption Perceptions Index, Available at:
https://www.transparency.org/news/feature/corruption
_perceptions_index_2017, (Accessed: 20 October
2018)
Deepika, S., 2012. Stemming Algorithms: A Comparative
Study and their Analysis. International Journal of
Applied Information Systems.
Dhurandhar, A., Ravi, R., Graves, B., Maniachari, G., Ettl,
M., 2015. Robust system for identifying procurement
fraud. In Proceedings of the twenty seventh conference
on innovative applications in artificial intelligence
(IAAI-15), 3896–3903.
Diaz, G., 2016. Stopwords Croatia, Available at:
https://github.com/stopwords-iso/stopwords-hr,
(Accessed: 15 October 2018).
Directorate for the public procurement system, 2017.
Statistical report for 2017 year, Available at:
http://www.javnanabava.hr/userdocsimages/Statisticko
_izvjesce_JN-2017.pdf, (Accessed: 15 October 2018).
Domingos, SL., Carvalho, RN., Carvalho, RS., Ramos,
GN., 2016. Identifying IT purchases anomalies in the
Brazilian government procurement system using deep
learning. Machine Learning and Applications
(ICMLA).
Efstathios, K., Charalambos, S., Yannis, M., 2007. Data
mining techniques for the detection of fraudulent
financial statements. Expert Systems with Applications.
Eman, Y., 2015. Sentiment Analysis and Text Mining for
Social Media Microblogs using Open Source Tools: An
Empirical Study. International Journal of Computer
Applications.
European Commission, 2017. European semester thematic
factsheet public procurement, Available at:
https://ec.europa.eu/info/sites/info/files/file_import/eur
opean-semester_thematic-factsheet_public-
procurement_en_0.pdf, (Accessed: 08 October 2018).
Fazekas, M., Kocsis, G., 2017. Uncovering high-level
corruption: Cross-national objective corruption risk
indicators using public procurement data. British
Journal of Political Science.
Fazekas, M., Tóth, IJ., King, LP., 2016. An Objective
Corruption Risk Index Using Public Procurement Data.
European Journal on Criminal Policy and Research.
Ferwerda, J., Deleanu, I., Unger, B., 2016. Corruption in
Public Procurement: Finding the Right Indicators.
European Journal on Criminal Policy and Research.
Fissette, M., 2017 Text mining to detect indications of fraud
in annual reports worldwide. Dissertation, University of
Twente.
Gupta, V., Lehal, GS., 2009. A Survey of Text Mining
Techniques and Applications. Journal of Emerging
Technologies in Web Intelligence.
Gupta, R., Gill, NS., 2012. Financial Statement Fraud
Detection using Text Mining. International Journal of
Advanced Computer Science and Applications.
Harrington, P., 2012. Machine learning in action. NY:
Manning Publications Co., Shelter Island.
Kotsiantis, S., Koumanako, E., Tzelepis, D., Tampakas, V.,
2007. Forecasting Fraudulent Financial Statements
using Data Mining. International Journal of
Computational Intelligence.
Prediction of Public Procurement Corruption Indices using Machine Learning Methods
339