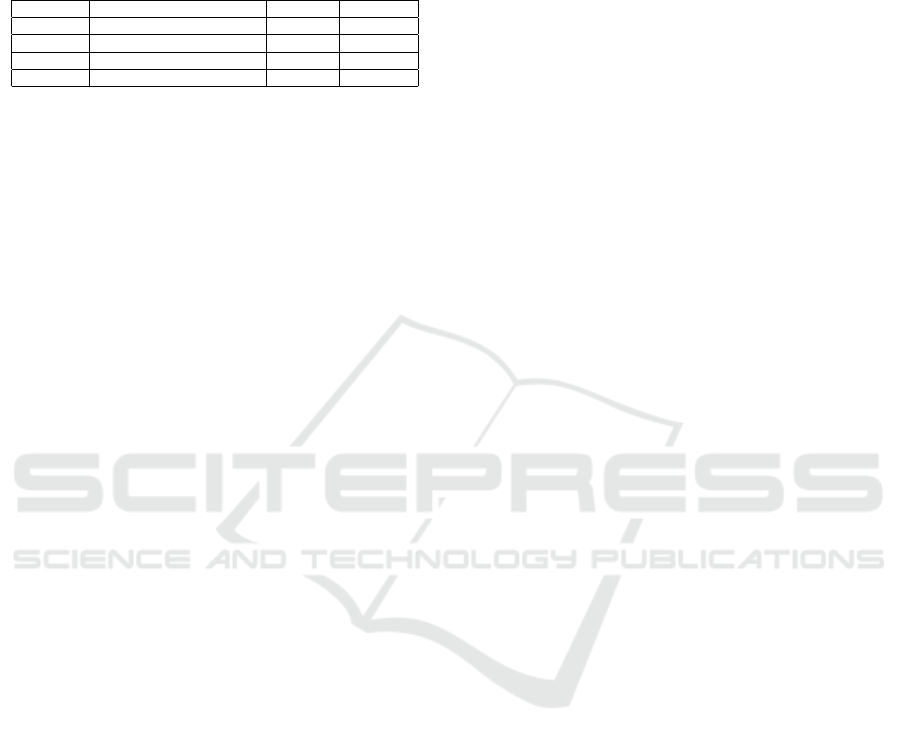
but also a big importance in constructing the Algerian
vocabulary.
For more details, see table2 , which summarises
the results of each dataset individually.
Table 2: Best classification results of each dataset.
Accuracy F-measure
wacht7ass PV+SVM+TF-IDF 81,63 % 84,47 %
G-Form PVT+Naive bayes+TF-IDF 80,87 %
G-Form PVT+SVM+TF 78,32 %
Brandt PVT+SVM+TF-IDF 94,24 % 90,67 %
For instance, some results labled Facebook
comments are given at https://drive.google.com/file/
d/1oFmoETRYys8ZHjcZcQCZqubIJ3Ceex66/view?
usp=sharing
5 CONCLUSIONS
In this paper, we presented a supervised approach for
sentiment analysis in Algerian dialect written in Latin
script, which gave interesting results despite the many
specific aspects of the dialect and complexity of Ara-
bizi analysis. We report results from an extensive
empirical evaluation assessing the effects of classi-
fiers, the effects of presentation types (count, TF, TF-
IDF) and those of novel contributions in preprocess-
ing phase, notably, vowels removing. Three data sets
were annotated with their respective sentiment labels
using crowdsourcing in this experiment. We achieved
an F-score of 87 % and an accuracy of 83 % using
this approach. Results revealed also that SVM out-
performs the other classifiers. Finally, the preprocess-
ing allowed us to impove f-score of SVM by 9,20 %,
which is considerable and shows the relevance of our
prior premises.
Our work can be improved in various directions. First,
we will test other models (random forest, gradient-
boosted trees, Latent Dirichlet Allocation model). We
could also explore other characteristics and feature
such as emoji interpretation and Irony/Sarcasm detec-
tion or other areas of opinion mining field, notably,
subjectivity analysis and rumor detection.
REFERENCES
Abdul-Mageed, M., Diab, M., and K
¨
ubler, S. (2014).
Samar: Subjectivity and sentiment analysis for ara-
bic social media. Computer Speech & Language,
28(1):20–37.
Abdulla, N., Mohammed, S., Al-Ayyoub, M., Al-Kabi, M.,
et al. (2014). Automatic lexicon construction for ara-
bic sentiment analysis. In 2014 International Confer-
ence on Future Internet of Things and Cloud, pages
547–552. IEEE.
Al-Ayyoub, M., Essa, S. B., and Alsmadi, I. (2015).
Lexicon-based sentiment analysis of arabic tweets.
IJSNM, 2(2):101–114.
Al-Ayyoub, M., Khamaiseh, A. A., Jararweh, Y., and Al-
Kabi, M. N. (2019). A comprehensive survey of arabic
sentiment analysis. Information Processing & Man-
agement, 56(2):320–342.
Ali, C. B., Mulki, H., and Haddad, H. (2018). Impact du
pr
´
etraitement linguistique sur l’analyse des sentiments
du dialecte tunisien. In Actes de la conf
´
erence Traite-
ment Automatique de la Langue Naturelle, TALN
2018, page 383.
Badaro, G., Baly, R., Hajj, H., Habash, N., and El-Hajj, W.
(2014). A large scale arabic sentiment lexicon for ara-
bic opinion mining. In Proceedings of the EMNLP
2014 workshop on arabic natural language process-
ing (ANLP), pages 165–173.
Bayoudhi, A., Ghorbel, H., Koubaa, H., and Belguith, L. H.
(2015). Sentiment classification at discourse segment
level: Experiments on multi-domain arabic corpus.
JLCL, 30(1):1–24.
Bettiche, M., Mouffok, M. Z., and Zakaria, C. (2018).
Opinion mining in social networks for algerian di-
alect. In International Conference on Informa-
tion Processing and Management of Uncertainty in
Knowledge-Based Systems, pages 629–641. Springer.
Cherif, W., Madani, A., and Kissi, M. (2015). Towards
an efficient opinion measurement in arabic comments.
Procedia Computer Science, 73:122–129.
Hadi, W. (2015). Classification of arabic social media data.
Advances in Computational Sciences and Technology,
8(1):29–34.
Ibrahim, H. S., Abdou, S. M., and Gheith, M. (2015). Mika:
A tagged corpus for modern standard arabic and col-
loquial sentiment analysis. In 2015 IEEE 2nd Inter-
national Conference on Recent Trends in Information
Systems (ReTIS), pages 353–358. IEEE.
Mataoui, M., Zelmati, O., and Boumechache, M. (2016). A
proposed lexicon-based sentiment analysis approach
for the vernacular algerian arabic. Res. Comput. Sci,
110:55–70.
Medhaffar, S., Bougares, F., Est
`
eve, Y., and Hadrich-
Belguith, L. (2017). Sentiment analysis of tunisian
dialects: Linguistic ressources and experiments. In
Proceedings of the third Arabic natural language pro-
cessing workshop, pages 55–61.
Medhat, W., Hassan, A., and Korashy, H. (2014). Sentiment
analysis algorithms and applications: A survey. Ain
Shams engineering journal, 5(4):1093–1113.
Mustafa, H. H., Mohamed, A., and Elzanfaly, D. S. (2017).
An enhanced approach for arabic sentiment analy-
sis. International Journal of Artificial Intelligence and
Applications (IJAIA), 8(5).
Zarra, T., Chiheb, R., Moumen, R., Faizi, R., and Afia, A. E.
(2017). Topic and sentiment model applied to the col-
loquial arabic: a case study of maghrebi arabic. In
Proceedings of the 2017 international conference on
smart digital environment, pages 174–181. ACM.
KDIR 2019 - 11th International Conference on Knowledge Discovery and Information Retrieval
482