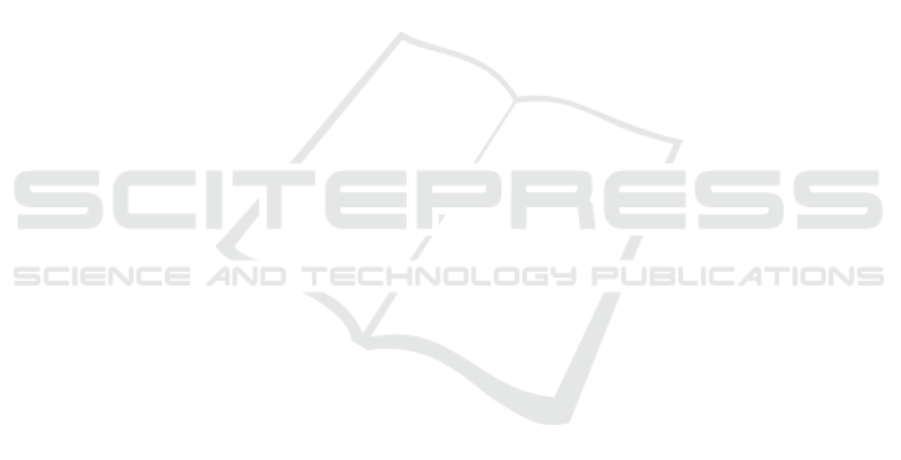
video domain and adapt it to the language domain.
The resulting sparse S matrices were then (1) pro-
jected back into the word space (compare figure 1),
(2) verbalized using extraction summarization (i.e.
with their surrounding sentences) and placed one af-
ter another to form the storyline, (3) we asked col-
leagues to evaluate the storylines (e.g. (Janaszkiewicz
et al., 2018)). In this informal evaluation the method
of (Zhou and Tao, 2011) gave the best results.
5 CONCLUSION
To stem the information deluge, many researchers
have proposed algorithms and techniques to mitigate
the often overwhelming stream of information. These
approaches are most often tailored to specific users,
kinds of information, or circumstances, see the very
comprehensive overview of (Strother et al., 2012).
We take the view that different kinds of informa-
tion streams, from news feeds, to mail exchanges,
to twitterstorms, all keep the reader in suspense of
the developing storyline. This allows us the unify-
ing approach of studying how to capture such sto-
rylines. We presented the analogy of book pages
to video frames, hence borrowed heavily from tech-
niques from the processing of surveillance videos. We
used the mathematics developed in the area of com-
pressed sensing and showed how it can be applied in
the linguistic domain for the discovery of storylines.
We have not extensively experimented to validate the
approach, but we showed that the sound underlying
mathematics, the cognitive plausibility, and the infor-
mal experiments are promising and warrant further in-
vestigation.
REFERENCES
Alashkar, T., Amor, B. B., Daoudi, M., and Berretti, S.
(2018). Spontaneous expression detection from 3D
dynamic sequences by analyzing trajectories on grass-
mann manifolds. IEEE Trans. Affective Computing,
9(2):271–284.
Allan, J., Carbonell, J., and Doddington, G. (1998). Topic
detection and tracking pilot study final report. In
Proc. DARPA Broadcast News Transcription and Un-
derstanding Workshop, pages 194–218.
AlSumait, L., Barbar
´
a, D., and Domeniconi, C. (2008).
On-line LDA: Adaptive topic models for mining text
streams with applications to topic detection and track-
ing. In Proc. 2008 Eighth IEEE International Confer-
ence on Data Mining, ICDM ’08, pages 3–12, Wash-
ington, DC, USA. IEEE Computer Society.
Baraniuk, R. G. (2007). Compressive Sensing. IEEE Signal
Processing Magazine, 24(118-120,124).
Bingham, E. and Mannila, H. (2001). Random projec-
tion in dimensionality reduction: Applications to im-
age and text data. In Proceedings of the Seventh
ACM SIGKDD International Conference on Knowl-
edge Discovery and Data Mining, KDD ’01, pages
245–250, New York, NY, USA. ACM.
Bouwmans, T., Javed, S., Zhang, H., Lin, Z., and Otazo,
R. (2018). On the applications of robust pca in im-
age and video processing. Proceedings of the IEEE,
106(8):1427–1457.
Cand
`
es, E. J., Li, X., Ma, Y., and Wright, J. (2011). Robust
principal component analysis? J. ACM, 58(3):11:1–
11:37.
C.Deerwester, S., Dumais, S. T., W.Furnas, G., Harshman,
R. A., Landauer, T. K., Lochbaum, K. E., and Streeter,
L. A. (1989). U.S. Patent No. 4,839,853. Washington,
DC: U.S. Patent and Trademark Office.
Edelman, A., Arias, T. A., and Smith, S. T. (1998). The ge-
ometry of algorithms with orthogonality constraints.
Siam J. Matrix Anal. Appl, 20(2):303–353.
Griffiths, T. L. and Steyvers, M. (2004). Finding scien-
tific topics. Proc. National Academy of Sciences,
101(5):5228–523.
Hage, C. and Kleinsteuber, M. (2013). Robust PCA and
subspace tracking from incomplete observations using
l
0
-surrogates. Computational Statistics, 29(3):467–
487.
Hearst, M. A. (1997). Texttiling: Segmenting text into
multi-paragraph subtopic passages. Comput. Lin-
guist., 23(1):33–64.
Hoenkamp, E. (2003). Unitary operators on the document
space. Journal of the American Society for Informa-
tion Science and Technology, 54(4):314–320.
Hoenkamp, E. (2012). Taming the terabytes: a human-
centered approach to surviving the information-
deluge. In Strother, J., Ulijn, J., and Fazal, Z., editors,
Information Overload : A Challenge to Professional
Engineers and Technical Communicators, IEEE PCS
professional engineering communication series, pages
147–170. John Wiley & Sons, Ltd, Hoboken, New Jer-
sey.
Hoenkamp, E. and Bruza, P. (2015). How everyday lan-
guage can and will boost effective information re-
trieval. Journal of the Association for Information Sci-
ence and Technology, 66(8):1546–1558.
Hofmann, T. (1999). Probabilistic latent semantic indexing.
In Proc. 22Nd Annual International ACM SIGIR Con-
ference on Research and Development in Information
Retrieval, SIGIR ’99, pages 50–57, New York, NY,
USA. ACM.
Janaszkiewicz, P., Krysi
´
nska, J., Prys, M., Kieruzel, M.,
Lipczy
´
nski, T., and R
´
o
˙
zewski, P. (2018). Text Sum-
marization For Storytelling: Formal Document Case,
volume 126, pages 1154 – 1161. Elsevier.
Johnson, W. B. and Lindenstrauss, J. (1984). Extensions of
lipschitz mappings into a hilbert space. In Conference
in modern analysis and probability, volume 26, pages
189–206. Amer. Math. Soc.
Luhn, H. P. (1957). A statistical approach to mechanized
Discovering the Geometry of Narratives and their Embedded Storylines
489